MIReAD: Simple Method for Learning High-quality Representations from Scientific Documents
conf_acl(2023)
摘要
Learning semantically meaningful representations from scientific documents can facilitate academic literature search and improve performance of recommendation systems. Pre-trained language models have been shown to learn rich textual representations, yet they cannot provide powerful document-level representations for scientific articles. We propose MIReAD, a simple method that learns high-quality representations of scientific papers by fine-tuning transformer model to predict the target journal class based on the abstract. We train MIReAD on more than 500,000 PubMed and arXiv abstracts across over 2,000 journal classes. We show that MIReAD produces representations that can be used for similar papers retrieval, topic categorization and literature search. Our proposed approach outperforms six existing models for representation learning on scientific documents across four evaluation standards.
更多查看译文
关键词
high-quality high-quality,learning
AI 理解论文
溯源树
样例
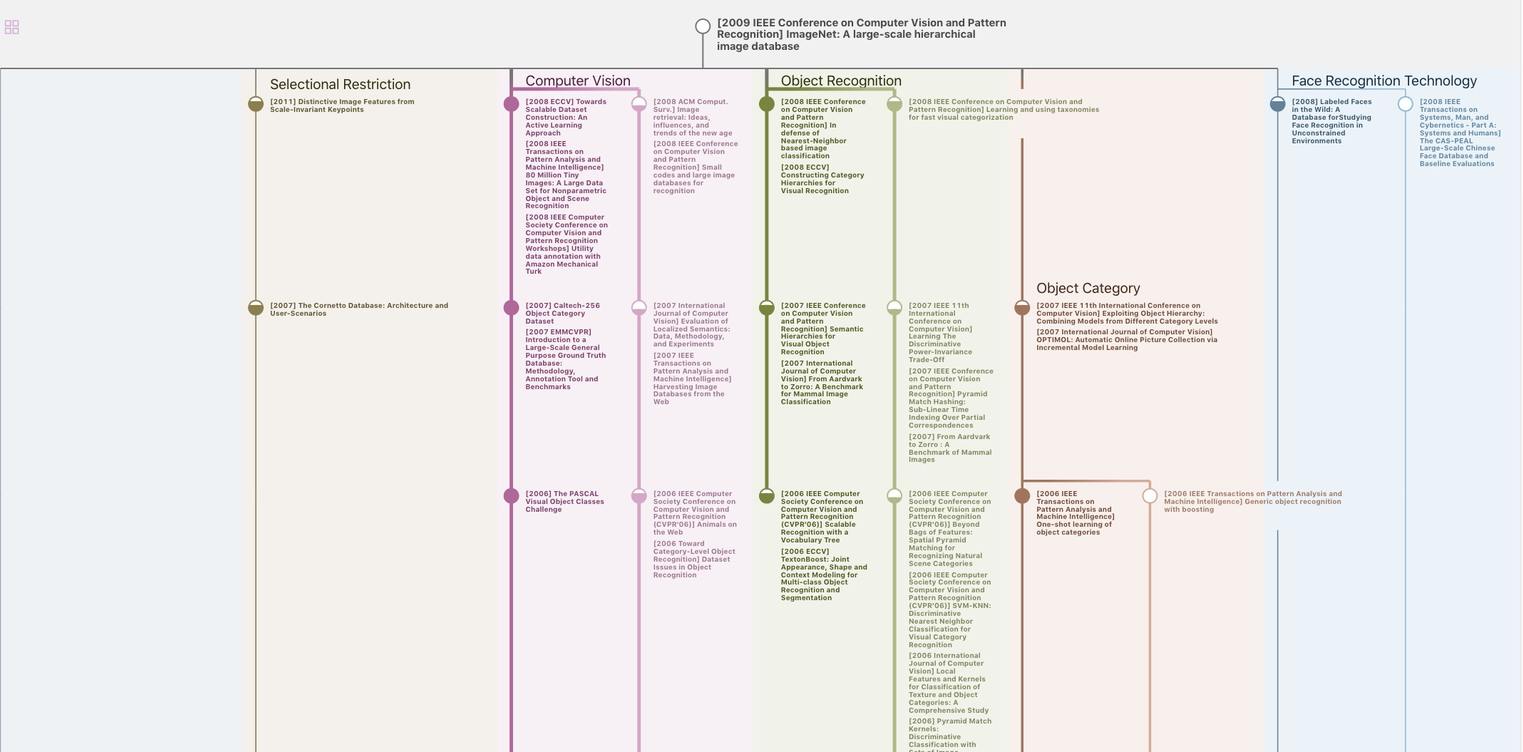
生成溯源树,研究论文发展脉络
Chat Paper
正在生成论文摘要