Trajectory-oriented Optimization of Stochastic Epidemiological Models
2023 Winter Simulation Conference (WSC)(2023)
Key words
Stochastic Model,Epidemiological Models,Stochastic Epidemiological Model,Parameter Settings,Input Parameters,Gaussian Process,Ensemble Members,Actual Trajectory,Goal Of Finding,Epidemic,Symptom Severity,Covariance Matrix,Empirical Data,Alternative Models,Observational Data,Loss Of Generality,White Noise,Computer Simulations,Global Optimization,Stochastic Simulations,Dimensionality Of The Input Space,Bayesian Optimization,Input Space,Predictive Distribution,Simulated Activity,Discrete Set,Kriging,Input Parameter Values
AI Read Science
Must-Reading Tree
Example
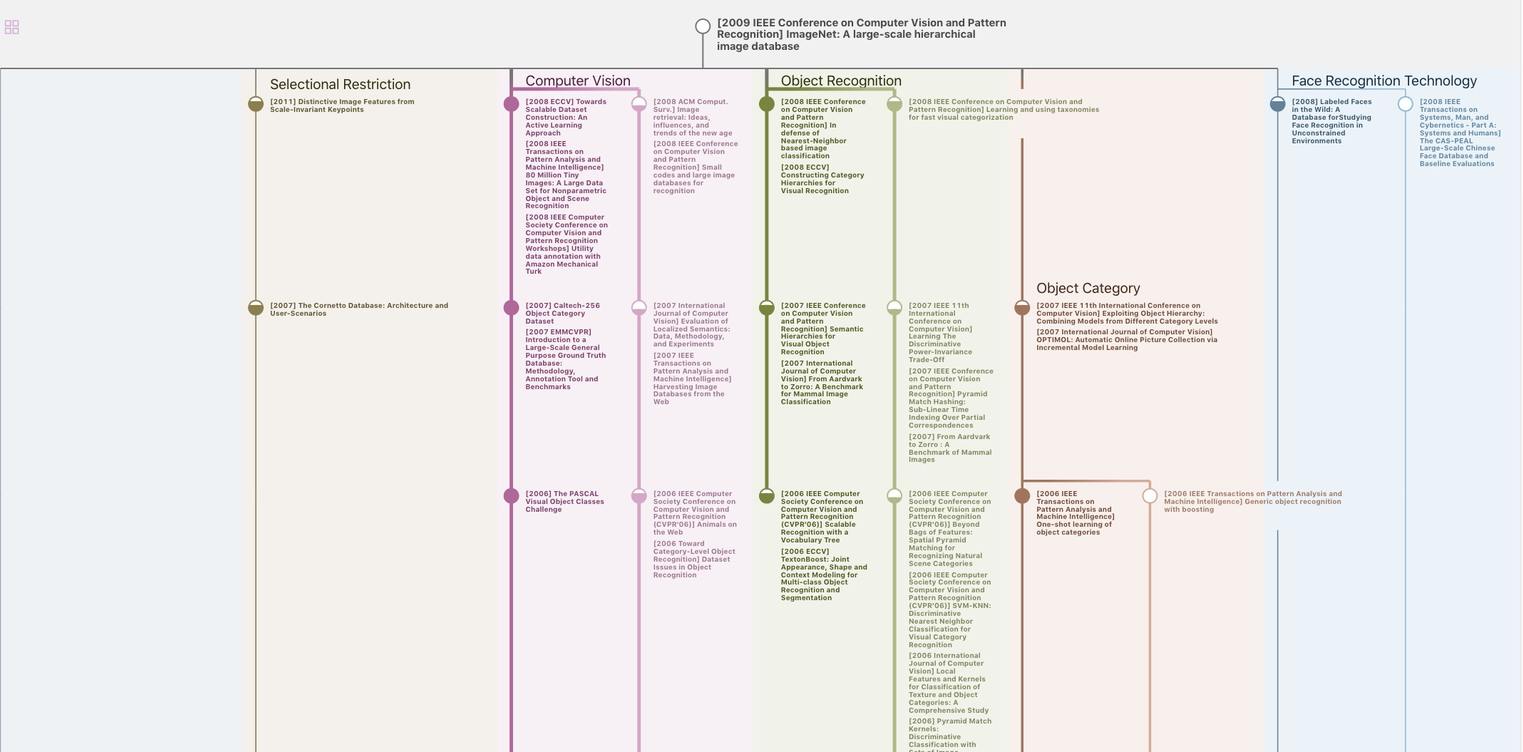
Generate MRT to find the research sequence of this paper
Chat Paper
Summary is being generated by the instructions you defined