Towards the Prediction of Atrial Fibrillation Using Interpretable ECG Features.
Computing in cardiology(2022)
摘要
Atrial fibrillation (AF) is our society's most common cardiac arrhythmic disease, leading to increased morbidity and mortality. Predicting AF episodes during sinus rhythm based on electrocardiograms (ECGs) allows timely interventions. It is known, that changes in selected ECG morphology features are a predictor for the onset of AF, but no systematic investigation of different ECG features' temporal changes has been performed so far. We split sinus rhythm episodes of 60 minutes preceding AF from the MIT-BIH AF database into segments of 5 minutes with 50% overlap $(n=644)$ and calculated 155 features of different domains per segment. Logistic regression analyses between the segments preceding AF and others revealed the most significant effects for segments ending 5 minutes before AF onset, with PQ interval slope (p < 0.01), PQ interval correlation (p < 0.05), and median RR time (p < 0.05) being the most relevant features. A decision tree ensemble, trained with all features, achieved an accuracy of 0.87 when distinguishing 8 segment clusters. Our results confirm expected changes in ECG features (e.g., PQ interval) before AF episodes, indicating impaired atrial excitation, and show that the combination of interpretable features is sufficient to discriminate at different points in time before AF onset. For advanced analyses, more extensive databases should be included.
更多查看译文
关键词
atrial fibrillation,ECG morphology features,interpretable ECG features,interpretable features,MIT-BIH AF database,predicting AF episodes,rhythm episodes,sinus rhythm
AI 理解论文
溯源树
样例
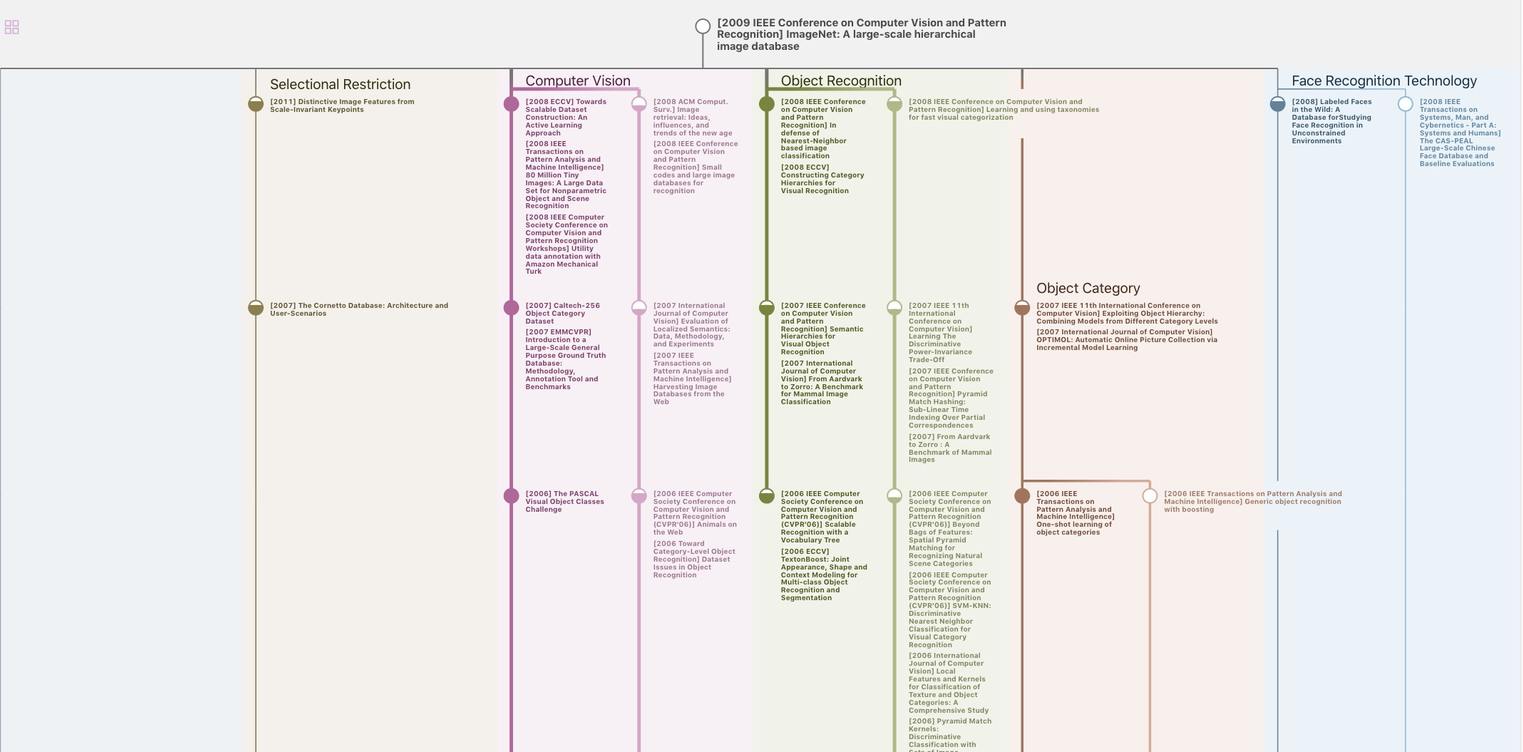
生成溯源树,研究论文发展脉络
Chat Paper
正在生成论文摘要