Deep Domain-Invariant Learning for Facial Age Estimation
Neurocomputing(2023)
摘要
Previous studies in facial age estimation can achieve promising performance when the training and test sets have a similar condition. However, these methods often fail to maintain performance and show significant degradation when encountering unseen domains. Therefore, we propose a novel method named Deep Domain-Invariant Learning (DDIL) to solve the Out-of-Distribution (OOD) generalization problem for facial age estimation. The proposed DDIL consists of the domain-invariant and style-invariant modules. The former extracts domain-specific features and trains a domain-invariant feature extractor by reducing the covariance discrepancy among features from different domains, while the latter leverages style randomization to overcome CNN’s induction bias towards styles. Consolidating these two modules, our DDIL can effectively decrease the influence of domain discrepancy. Extensive experiments on multiple age benchmark datasets under the Leave-One-Domain-Out Cross-Validation setting indicate superior performance in tackling age estimation generalization.
更多查看译文
关键词
Deep learning,Facial age estimation,Domain generalization
AI 理解论文
溯源树
样例
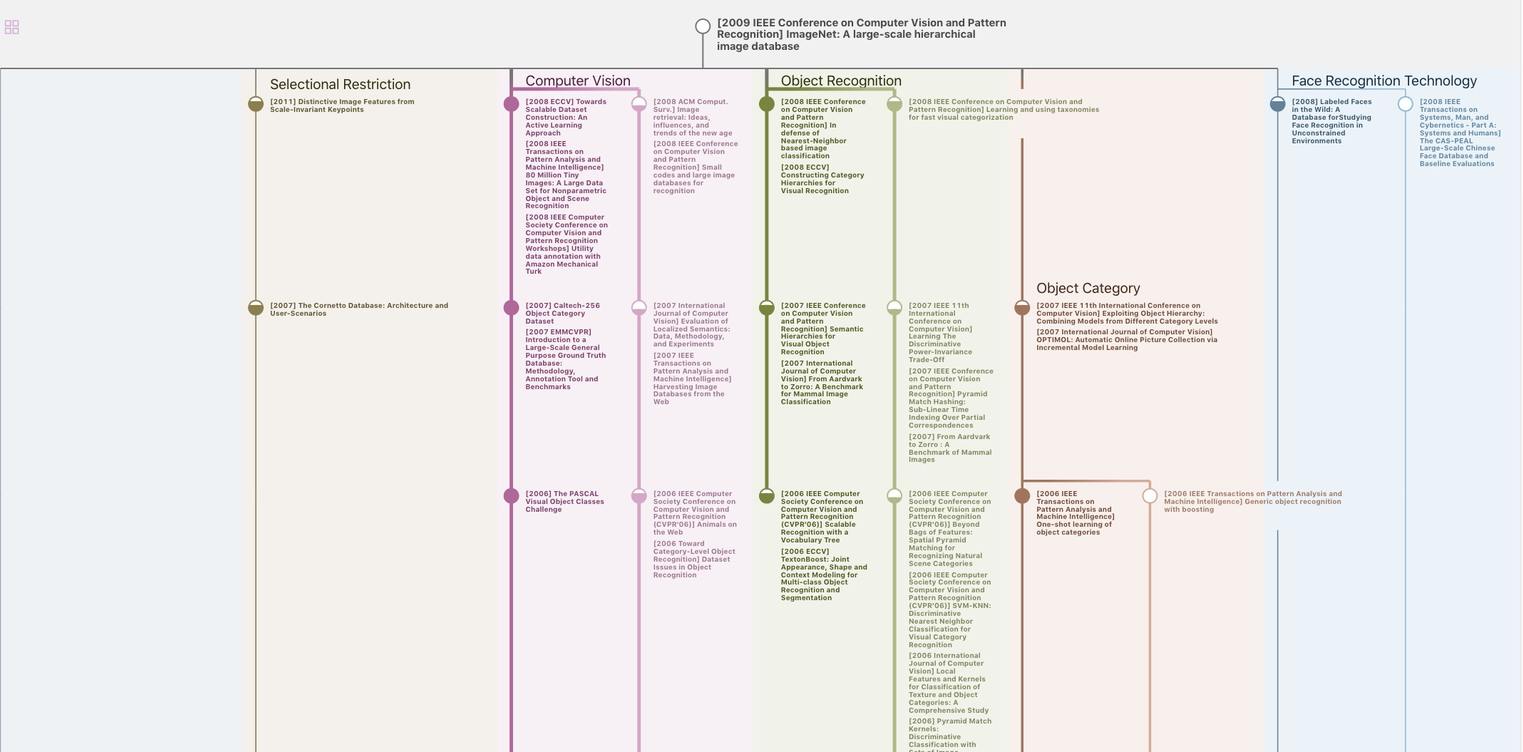
生成溯源树,研究论文发展脉络
Chat Paper
正在生成论文摘要