RainNet: A Large-Scale Dataset for Spatial Precipitation Downscaling.
CoRR(2020)
摘要
Spatial Precipitation Downscaling is one of the most important problems in the geo-science community. However, it still remains an unaddressed issue. Deep learning is a promising potential solution for downscaling. In order to facilitate the research on precipitation downscaling for deep learning, we present the first \textbf{REAL} (non-simulated) Large-Scale Spatial Precipitation Downscaling Dataset, \textbf{RainNet}, which contains \textbf{62,424} pairs of low-resolution and high-resolution precipitation maps for 17 years. Contrary to simulated data, this real dataset covers various types of real meteorological phenomena (e.g., Hurricane, Squall, etc.), and shows the physical characters - \textbf{Temporal Misalignment}, \textbf{Temporal Sparse} and \textbf{Fluid Properties} - that challenge the downscaling algorithms. In order to fully explore potential downscaling solutions, we propose an implicit physical estimation framework to learn the above characteristics. Eight metrics specifically considering the physical property of the data set are raised, while fourteen models are evaluated on the proposed dataset. Finally, we analyze the effectiveness and feasibility of these models on precipitation downscaling task. The Dataset and Code will be available at \url{this https URL}.
更多查看译文
关键词
spatial precipitation downscaling,large-scale
AI 理解论文
溯源树
样例
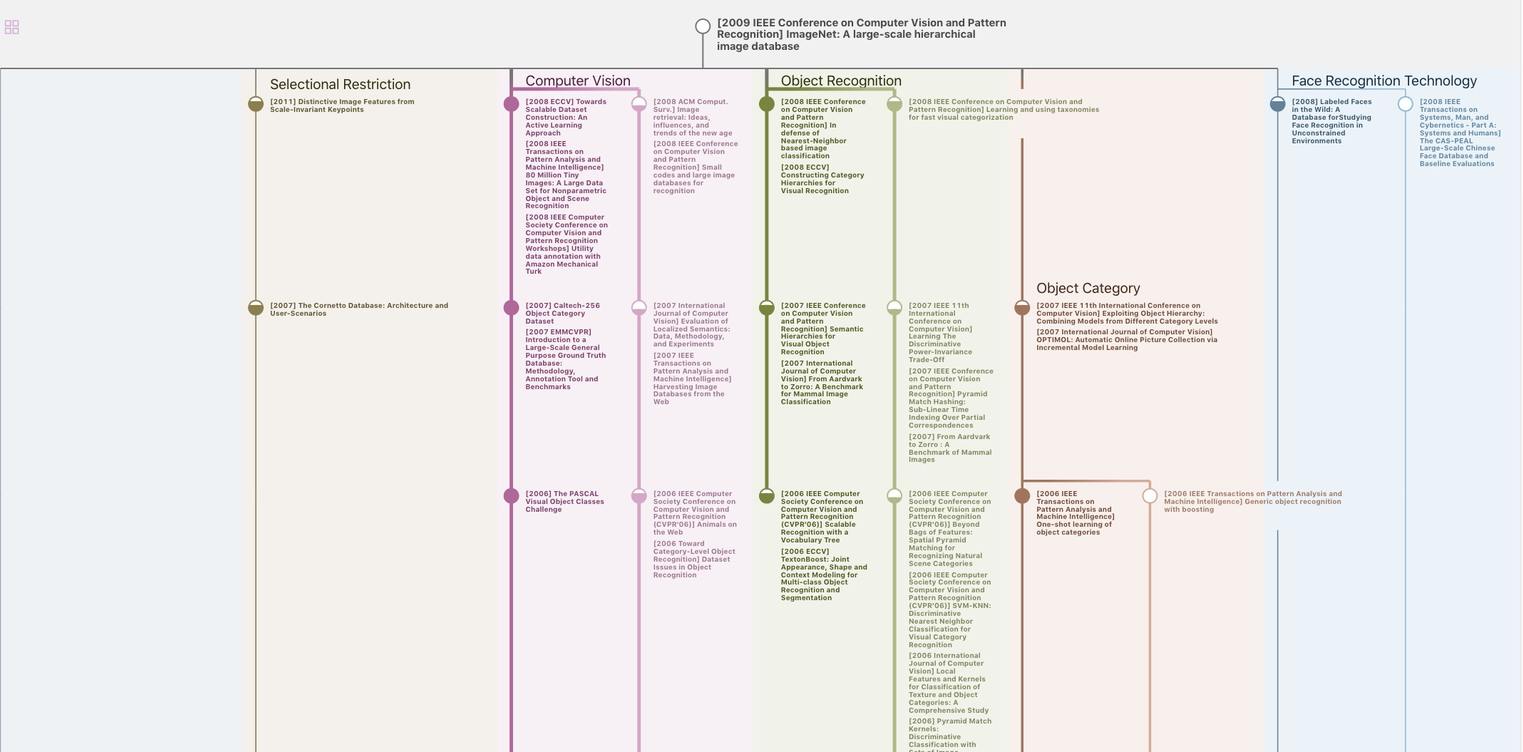
生成溯源树,研究论文发展脉络
Chat Paper
正在生成论文摘要