Quantitative Learning of LTL from Finite Traces.
CoRR(2021)
摘要
In this paper, we present a novel method for learning LTL properties from a set of traces. The main novelty of our method, as compared to many existing ones, is that we learn formulae in a quantitative sense : given a sample $\mathcal{S} = (P, N)$ consisting of positive traces $P$ and negative traces $N$, we find the formula $\varphi$ which describes the sample such that all positive traces satisfy $\varphi$ and all negative traces do not satisfy $\varphi$. To decide how good a formula is with respect to the sample, we have developed a scheme of assigning a value for a formula for a given trace under various schemes. We use the schemes to encode the optimal property synthesis problem, namely, finding the best property/LTL formula into an optimization problem. Then we use an SMT solver to find the best fit formula for a given set of traces. Finally, we present a hybrid approach combining classical LTL satisfaction and the ranking scheme that works on a fragment of LTL and greatly improves performance while maintaining reasonable expressiveness. We have developed a tool QuantLearn based on our method and applied on some benchmarks. We also compared different valuation schemes. Our experiments suggest that QuantLearn is successful in mining formulae which are reasonably good representations of the sample with high resilience to noise in the data.
更多查看译文
关键词
traces,ltl,quantitative learning
AI 理解论文
溯源树
样例
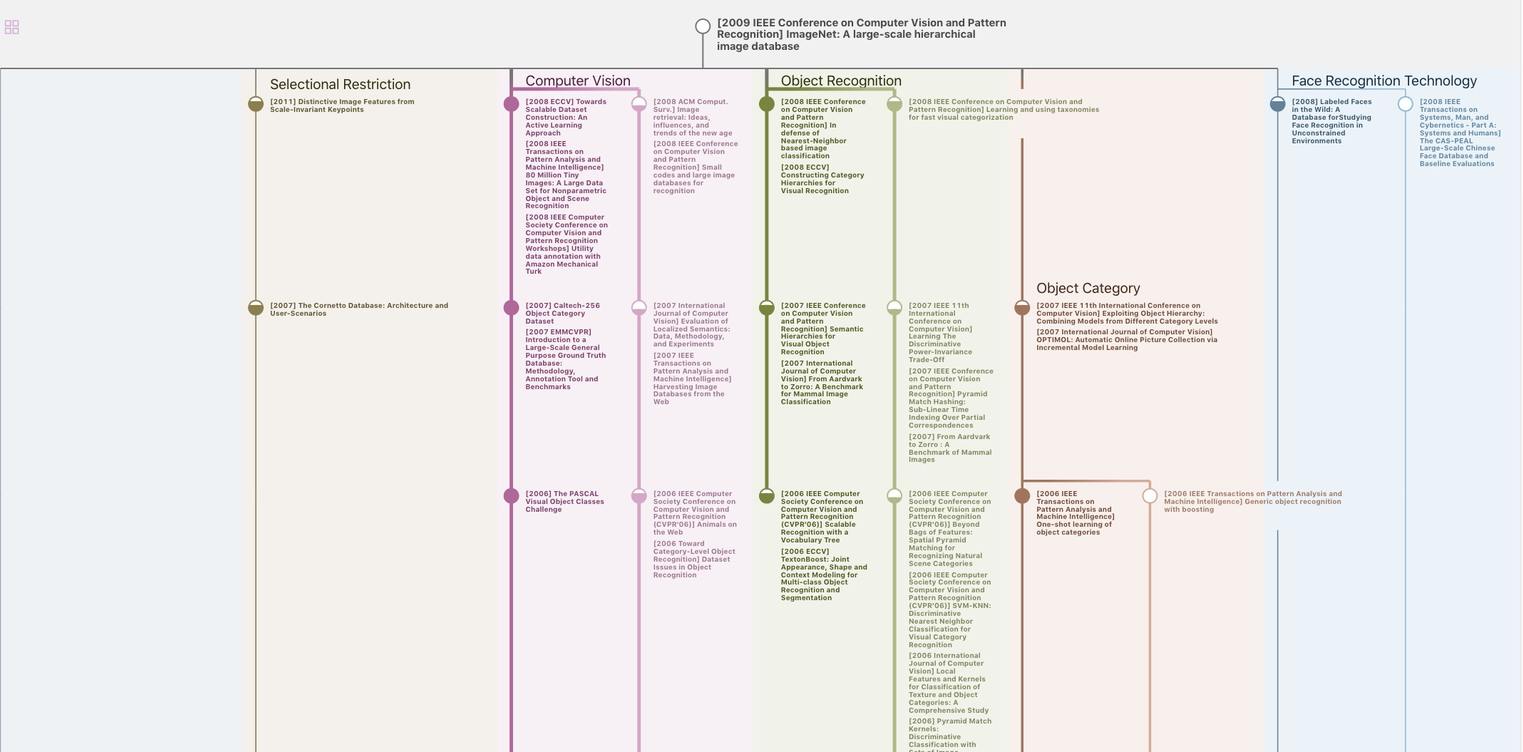
生成溯源树,研究论文发展脉络
Chat Paper
正在生成论文摘要