SimSC: A Simple Framework for Semantic Correspondence with Temperature Learning
CoRR(2023)
摘要
We propose SimSC, a remarkably simple framework, to address the problem of semantic matching only based on the feature backbone. We discover that when fine-tuning ImageNet pre-trained backbone on the semantic matching task, L2 normalization of the feature map, a standard procedure in feature matching, produces an overly smooth matching distribution and significantly hinders the fine-tuning process. By setting an appropriate temperature to the softmax, this over-smoothness can be alleviated and the quality of features can be substantially improved. We employ a learning module to predict the optimal temperature for fine-tuning feature backbones. This module is trained together with the backbone and the temperature is updated online. We evaluate our method on three public datasets and demonstrate that we can achieve accuracy on par with state-of-the-art methods under the same backbone without using a learned matching head. Our method is versatile and works on various types of backbones. We show that the accuracy of our framework can be easily improved by coupling it with more powerful backbones.
更多查看译文
关键词
semantic correspondence,temperature
AI 理解论文
溯源树
样例
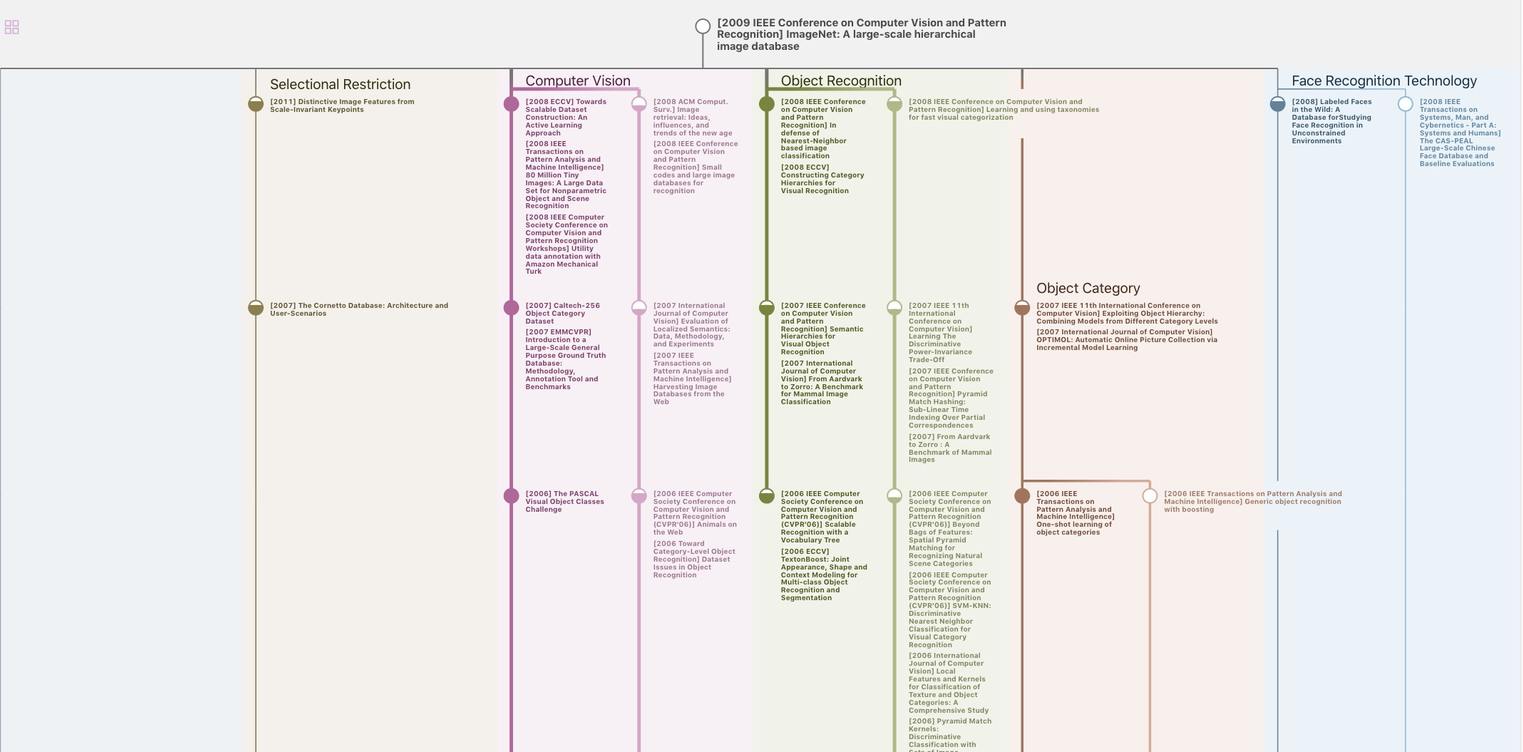
生成溯源树,研究论文发展脉络
Chat Paper
正在生成论文摘要