Spatial-Language Attention Policies for Efficient Robot Learning
CoRR(2023)
摘要
We investigate how to build and train spatial representations for robot decision making with Transformers. In particular, for robots to operate in a range of environments, we must be able to quickly train or fine-tune robot sensorimotor policies that are robust to clutter, data efficient, and generalize well to different circumstances. As a solution, we propose Spatial Language Attention Policies (SLAP). SLAP uses three-dimensional tokens as the input representation to train a single multi-task, language-conditioned action prediction policy. Our method shows 80% success rate in the real world across eight tasks with a single model, and a 47.5% success rate when unseen clutter and unseen object configurations are introduced, even with only a handful of examples per task. This represents an improvement of 30% over prior work (20% given unseen distractors and configurations).
更多查看译文
AI 理解论文
溯源树
样例
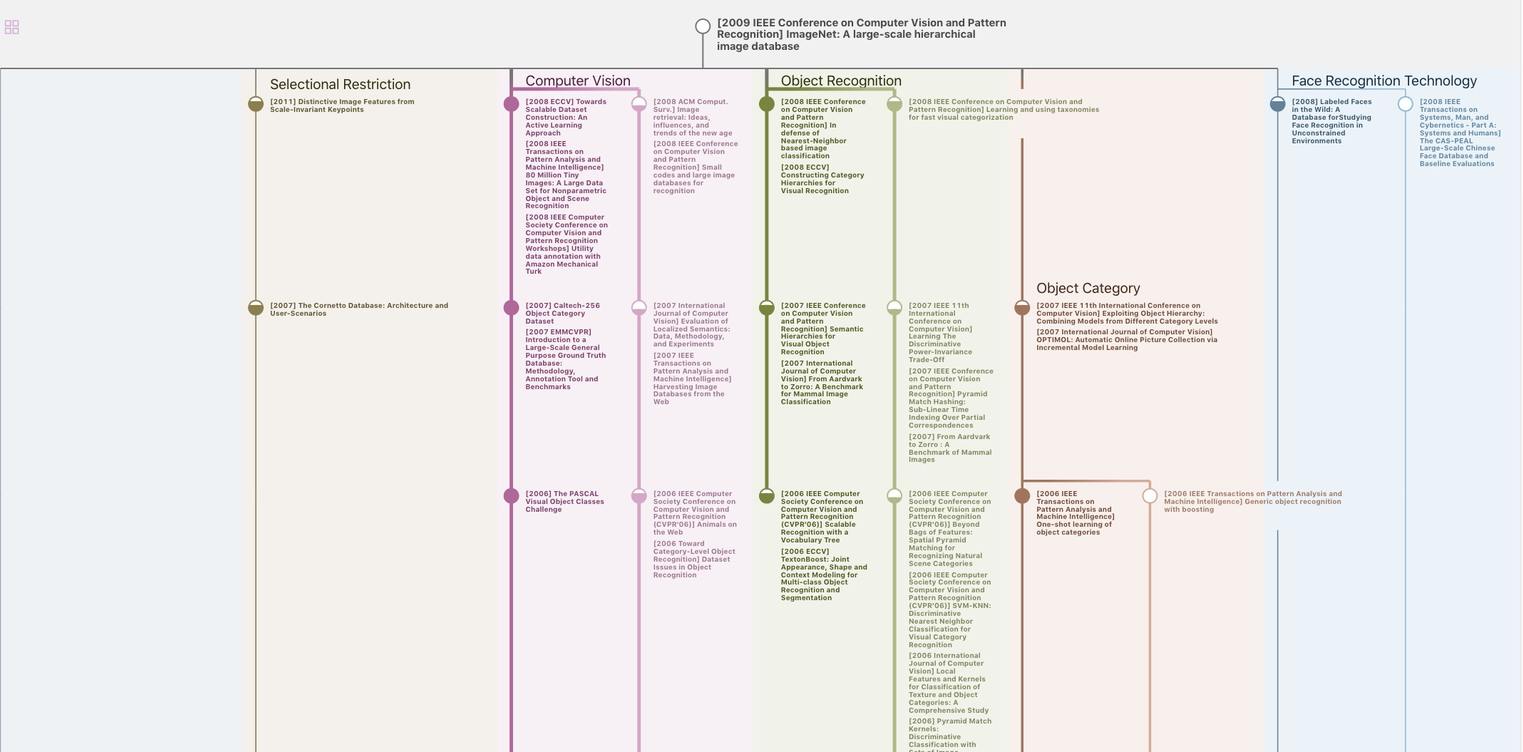
生成溯源树,研究论文发展脉络
Chat Paper
正在生成论文摘要