Unified understanding of nonparametric causality detection in time series
biorxiv(2023)
摘要
Most complex systems in the real world are driven by multiple interactions among components. Identifying these interactions is critical for understanding, forecasting, and controlling system-level phenomena. Transfer entropy (TE) and convergent cross mapping (CCM) are two widely-used approaches for nonparametric causality detection based on time-series data. However, the theoretical relationship between TE and CCM has not been formally explained. Here, we provide a theoretical formulation that links TE and CCM in an information-theoretic framework, showing that they have different definitions of causal influence. Furthermore, from the formulation, we propose a novel nonparametric causality test, named unified information-theoretic causality (UIC), which has lower data requirements than those of TE (due to robustness to noise) and lower false-positive rates than those of CCM. Numerical experiments confirmed that UIC outperforms TE and CCM in terms of the performance of causality detection for both linear and nonlinear dynamical systems. Finally, we demonstrate the practical importance of the conditional test based on UIC using numerical simulations with indirect causality and empirical data for microbial communities in irrigated water samples from rice fields. Our results contribute to a unified understanding of nonparametric causality tests in time series and the accurate reconstruction of complex real-world systems.
### Competing Interest Statement
The authors have declared no competing interest.
更多查看译文
AI 理解论文
溯源树
样例
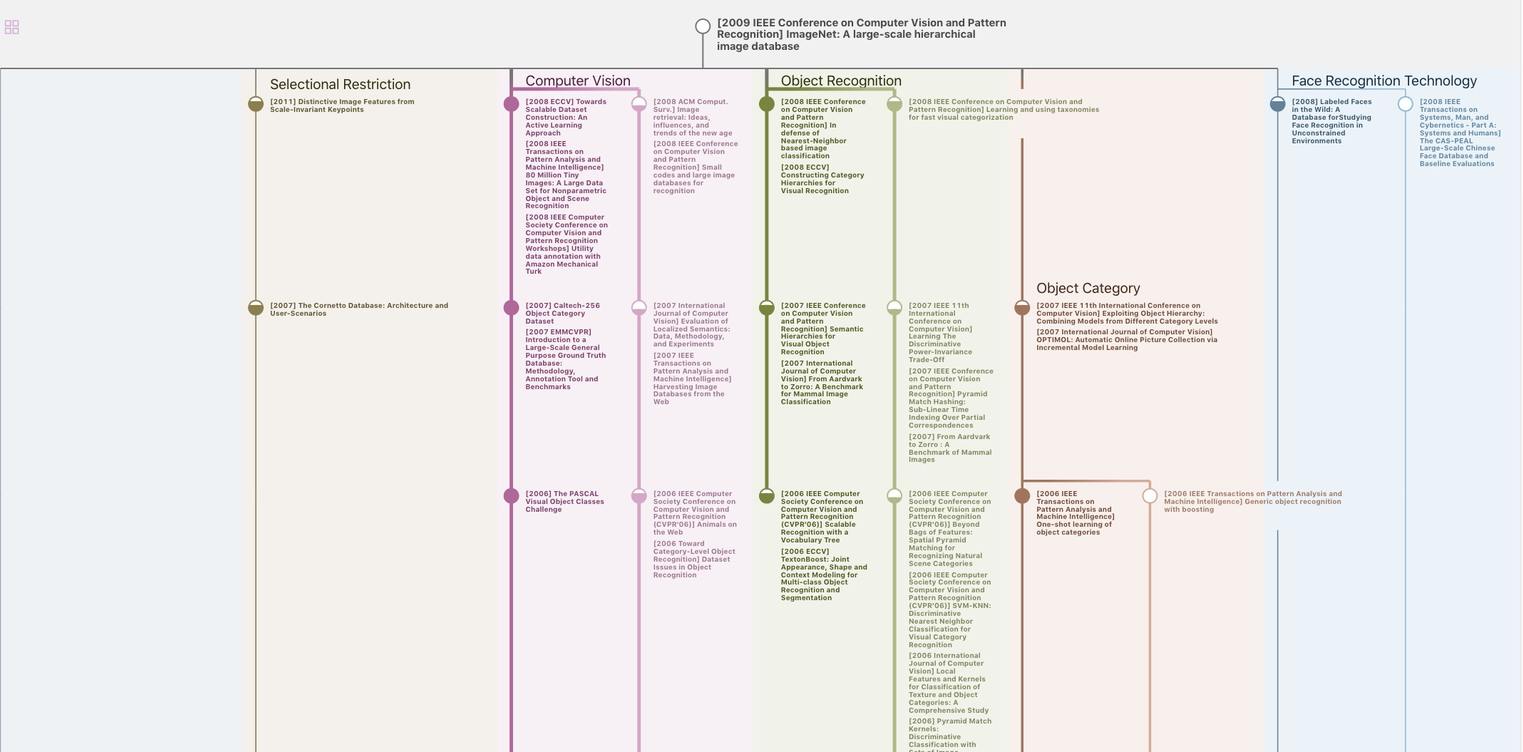
生成溯源树,研究论文发展脉络
Chat Paper
正在生成论文摘要