Scaling the leading accuracy of deep equivariant models to biomolecular\n simulations of realistic size
SC23: International Conference for High Performance Computing, Networking, Storage and Analysis(2023)
摘要
This work brings the leading accuracy, sample efficiency, and robustness of deep equivariant neural networks to the extreme computational scale. This is achieved through a combination of innovative model architecture, massive parallelization, and models and implementations optimized for efficient GPU utilization. The resulting Allegro architecture bridges the accuracy-speed tradeoff of atomistic simulations and enables description of dynamics in structures of unprecedented complexity at quantum fidelity. To illustrate the scalability of Allegro, we perform nanoseconds-long stable simulations of protein dynamics and scale up to a 44-million atom structure of a complete, all-atom, explicitly solvated HIV capsid on the Perlmutter supercomputer. We demonstrate excellent strong scaling up to 100 million atoms and 70% weak scaling to 5120 A100 GPUs.
更多查看译文
关键词
Biomolecular Simulations,Equivariant Models,Neural Network,Scalable,Strong Scaling,Excellent Scalability,Molecular Dynamics,Molecular Dynamics Simulations,Density Functional Theory,Chemical Processes,PyTorch,Multilayer Perceptron,Chemical Calculations,Tensor Product,Beginning Of The Simulation,Statistical Potentials,Simulation Speed,Input Tensor,Expensive Operations,Negligible Cost,Interatomic Potential,Higher-order Tensors,Feature Tensor,Thousands Of Atoms,Einstein Summation,Neighbor List,Biomolecular Systems,Computational Biology,Variety Of Systems,Spatial Decomposition
AI 理解论文
溯源树
样例
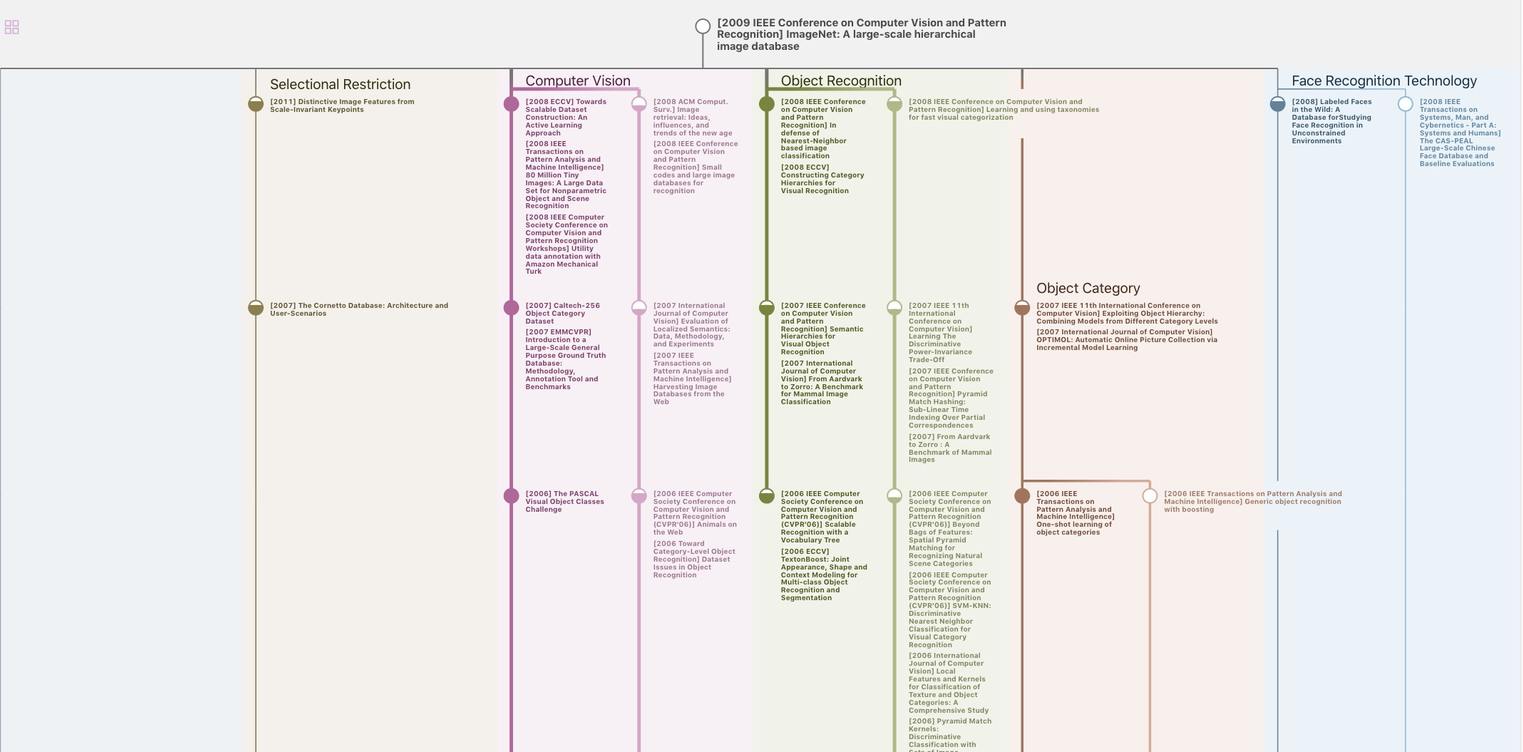
生成溯源树,研究论文发展脉络
Chat Paper
正在生成论文摘要