Multifidelity uncertainty quantification with models based on dissimilar parameters
Computer Methods in Applied Mechanics and Engineering(2023)
摘要
Multifidelity uncertainty quantification (MF UQ) sampling approaches have been shown to significantly reduce the variance of statistical estimators while preserving the bias of the highest-fidelity model, provided that the low-fidelity models are well correlated. However, maintaining a high level of correlation can be challenging, especially when models depend on different input uncertain parameters, which drastically reduces the correlation. Existing MF UQ approaches do not adequately address this issue. In this work, we propose a new sampling strategy that exploits a shared space to improve the correlation among models with dissimilar parameterization. We achieve this by transforming the original coordinates onto an auxiliary manifold using the adaptive basis (AB) method (Tipireddy and Ghanem, 2014). The AB method has two main benefits: (1) it provides an effective tool to identify the low-dimensional manifold on which each model can be represented, and (2) it enables easy transformation of polynomial chaos representations from high- to low-dimensional spaces. This latter feature is used to identify a shared manifold among models without requiring additional evaluations. We present two algorithmic flavors of the new estimator to cover different analysis scenarios, including those with legacy and non-legacy high-fidelity (HF) data. We provide numerical results for analytical examples, a direct field acoustic test, and a finite element model of a nuclear fuel assembly. For all examples, we compare the proposed strategy against both single-fidelity and MF estimators based on the original model parameterization.
更多查看译文
关键词
Uncertainty quantification,Multi-fidelity,Monte Carlo,Polynomial chaos,Dimension reduction,Finite element method
AI 理解论文
溯源树
样例
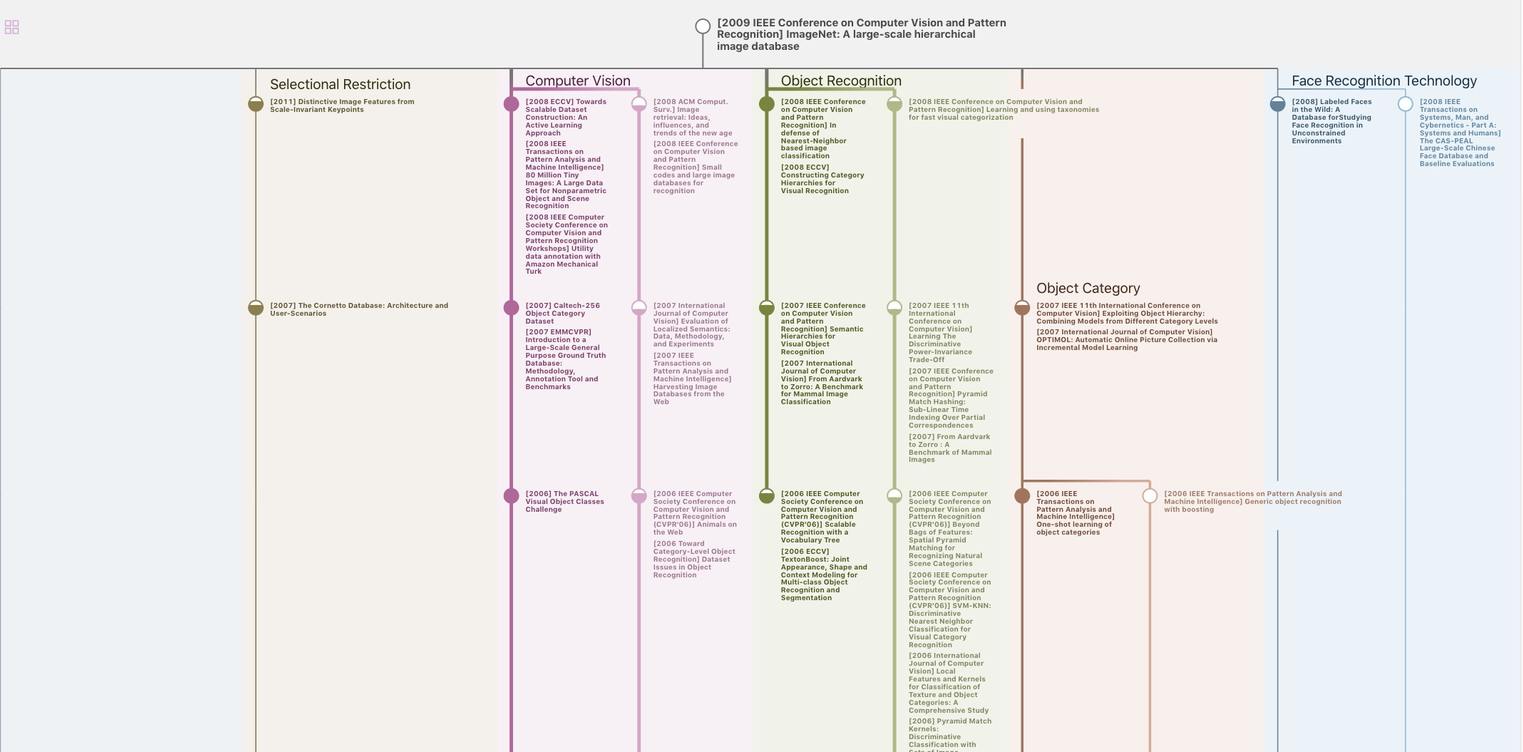
生成溯源树,研究论文发展脉络
Chat Paper
正在生成论文摘要