Hybrid Forecasting System Considering the Influence of Seasonal Factors under Energy Sustainable Development Goals
Measurement(2023)
摘要
As environmental concerns and the energy crisis intensify, advancing the use of new energy sources such as wind and photovoltaic is an important means to achieve sustainable energy development. However, the random fluctuation characteristics and seasonality of wind and light make them difficult to predict, which brings many operational risks to grid security and power dispatch. Therefore, this paper uses the Complete Ensemble Empirical Mode Decomposition with Adaptive Noise (CEEMDAN) as the decomposition algorithm, which can effectively avoid the problems of modal confusion and noise residuals and better identify the trend, seasonality and nonlinear characteristics of the series. The Particle Swarm Optimization (PSO) algorithm is also utilized to improve the prediction accuracy and stability of the Extreme Learning Machine (ELM). Combining CEEMDAN and PSO-ELM models, a high-precision hybrid forecasting system that can effectively reduce the effects of sto-chastic fluctuations is proposed. In this paper, wind speed data from four different seasons at Changma wind farm in China are selected to verify the effectiveness and generalization ability of this hybrid prediction system. The results show that the improvement of ELM by PSO and CEEMDAN significantly improves the prediction accuracy of the model, and the hybrid prediction system can be applied to time series with different data characteristics and variation patterns in each season, and its prediction accuracy and model performance are significantly better than other comparative models.
更多查看译文
关键词
Renewable energy,Hybrid prediction system,Data processing,PSO,CEEMDAN
AI 理解论文
溯源树
样例
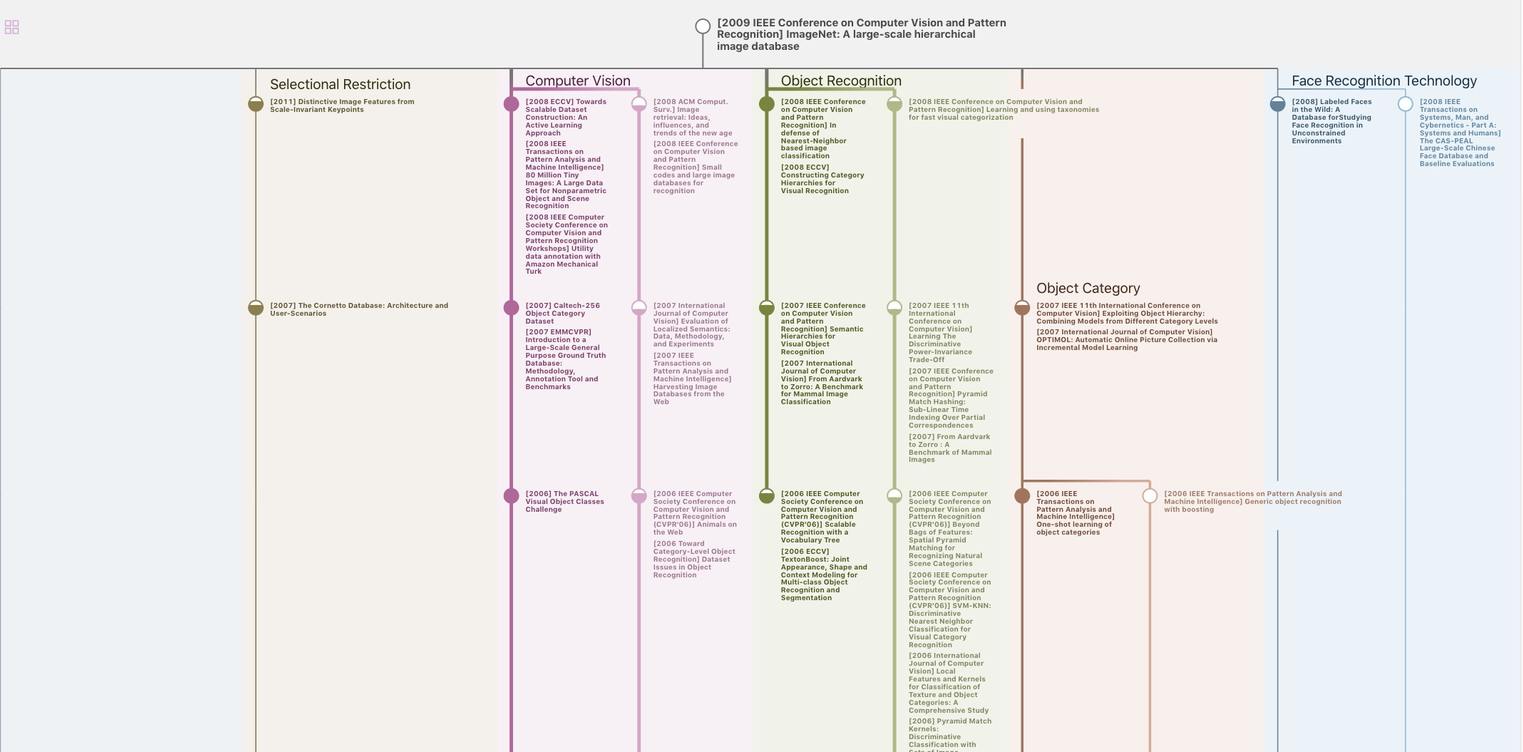
生成溯源树,研究论文发展脉络
Chat Paper
正在生成论文摘要