Light Field Image Super-Resolution Network via Joint Spatial-Angular and Epipolar Information
IEEE Transactions on Computational Imaging(2023)
摘要
This paper proposes a novel efficient convolutional neural network (CNN) for light field (LF) spatial super-resolution (SR). Due to the high dimensionality of LF data, it is important to decompose the original 4D LF data structure into a lower data subspace helping to reduce memory and computational costs. In this context, we propose a novel hybrid CNN structure working on different 2D data representations to fully handle the 4D structure. Our proposed network can simultaneously exploit information from three different types of 4D LF representations: spatial, angular, and epipolar plane image (EPI) information. Based on pixel similarities of each data subspace, we propose an inter-intra spatial feature extractor (II-SFE) and an inter-intra angular feature extractor (II-AFE) that can exploit the pixel correlations among the spatial-angular domain. Moreover, based on the correlated epipolar geometry among pixels, we also propose a multi-orientation epipolar feature extractor (MO-EFE) by decomposing an LF image into four orientation stacks of views and feeding them into different network branches to learn rich sub-pixel information in the EPI domain. The extracted features of each 2D subspace are integrated by our proposed attention fusion module (AFM), which adaptively rescales the embedding weights based on their correlations. Experimental results on both small- and wide-baseline benchmark LF datasets confirm the effectiveness of the proposed method compared to the well-known state-of-the-art methods in terms of objective and subjective performance.
更多查看译文
关键词
Light field super-resolution,hybrid data representation,inter-intra spatial-angular features,attention fusion module
AI 理解论文
溯源树
样例
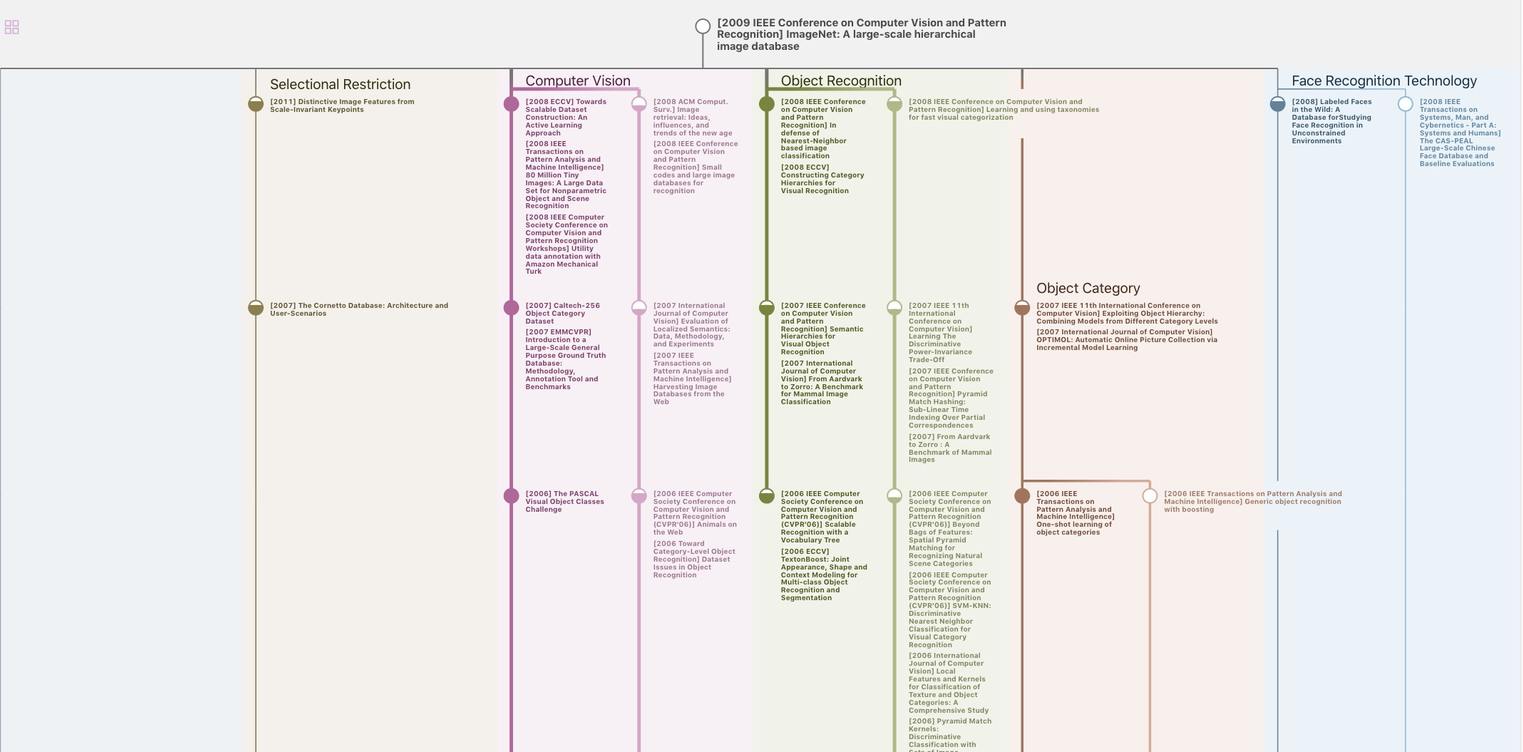
生成溯源树,研究论文发展脉络
Chat Paper
正在生成论文摘要