A Deep Transfer Learning based approach for forecasting spatio-temporal features to maximize yield in cotton crops
2023 57th Annual Conference on Information Sciences and Systems (CISS)(2023)
摘要
Cotton is an important economic crop farmed in the United States. Monitoring cotton crop growth metrics during in-season growth, from early season growth to harvest, is critical. Because cotton crop output is directly related to management decisions made to regulate growth parameters during a cultivation season, utilizing forecasting models to predict future values of canopy indices has piqued the interest of researchers. In this paper, we have used the canopy feature data i.e. Canopy Cover, Canopy Height and Excess Green Index recorded in the year 2020 and trained a multi-layer stacked LSTM model. Next, a Deep Transfer Learning based approach was used to freeze the weights of the initial layers of the trained LSTM model, and the weights of the last few layers were fine-tuned based on the 2021 cultivation year canopy index data to predict the canopy features from 28th day of cultivation to the end of the harvesting period.
更多查看译文
关键词
deep transfer learning,canopy cover,canopy height,excess green index
AI 理解论文
溯源树
样例
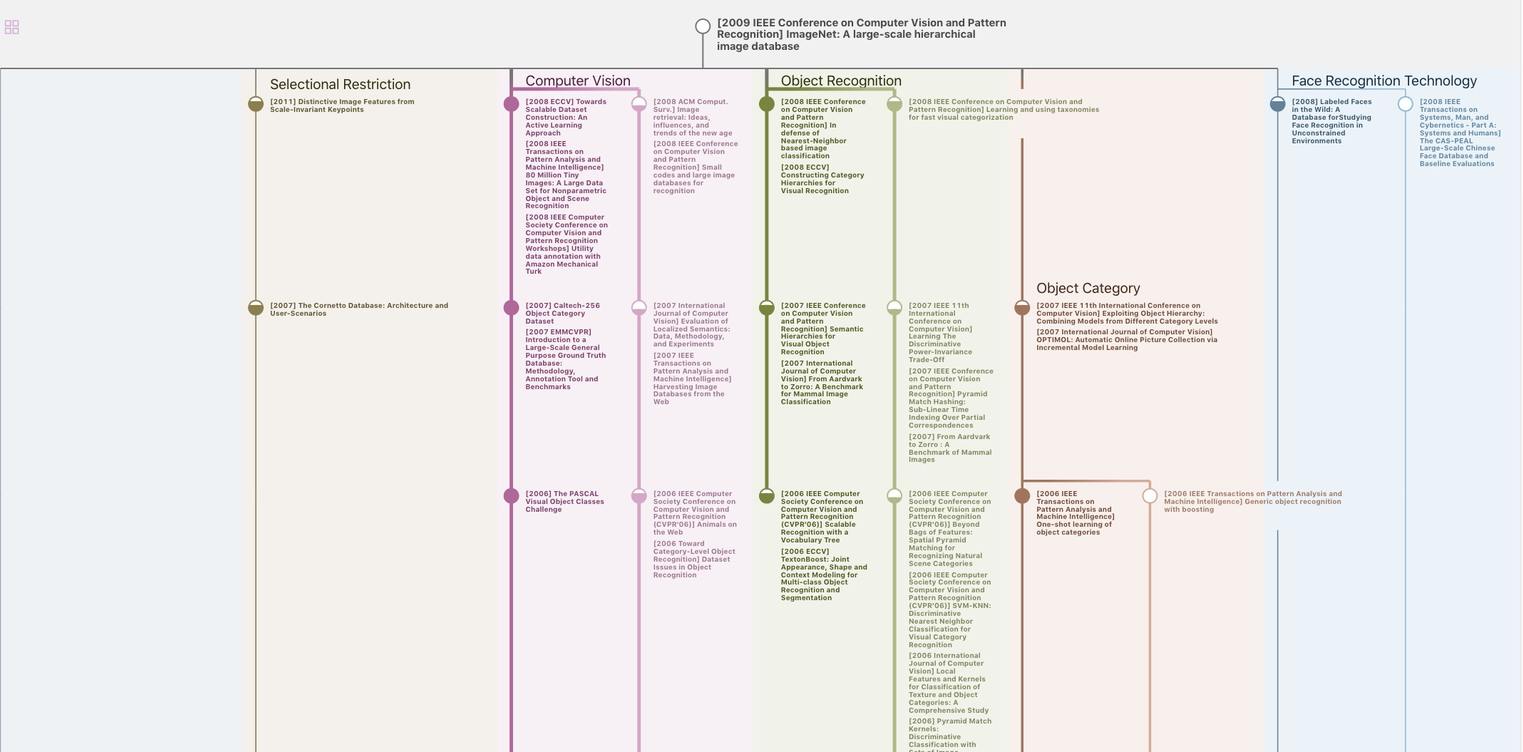
生成溯源树,研究论文发展脉络
Chat Paper
正在生成论文摘要