Differentiable graph-structured models for inverse design of lattice materials
CELL REPORTS PHYSICAL SCIENCE(2023)
Abstract
Architected materials possessing physico-chemical properties adaptable to disparate environmental conditions embody a disruptive new domain of materials science. Fueled by advances in digital design and fabrication, materials shaped into lattice topologies enable a degree of property customization not afforded to bulk ma-terials. A promising venue for inspiration toward their design is in the irregular micro-architectures of nature. However, the immense design variability unlocked by such irregularity is challenging to probe analytically. Here, we propose a new computational approach using graph-based representation for regular and irregular lattice materials. Our method uses differentiable message passing algorithms to calculate mechanical properties, allowing automatic differentiation with surrogate derivatives to adjust geometric structure and local attributes of individual lattice elements to achieve inversely designed materials with desired properties. We further introduce a graph neural network surrogate model for structural analysis at scale. The methodology is generalizable to any system representable as heterogeneous graphs.
MoreTranslated text
Key words
inverse design,lattice,models,graph-structured
AI Read Science
Must-Reading Tree
Example
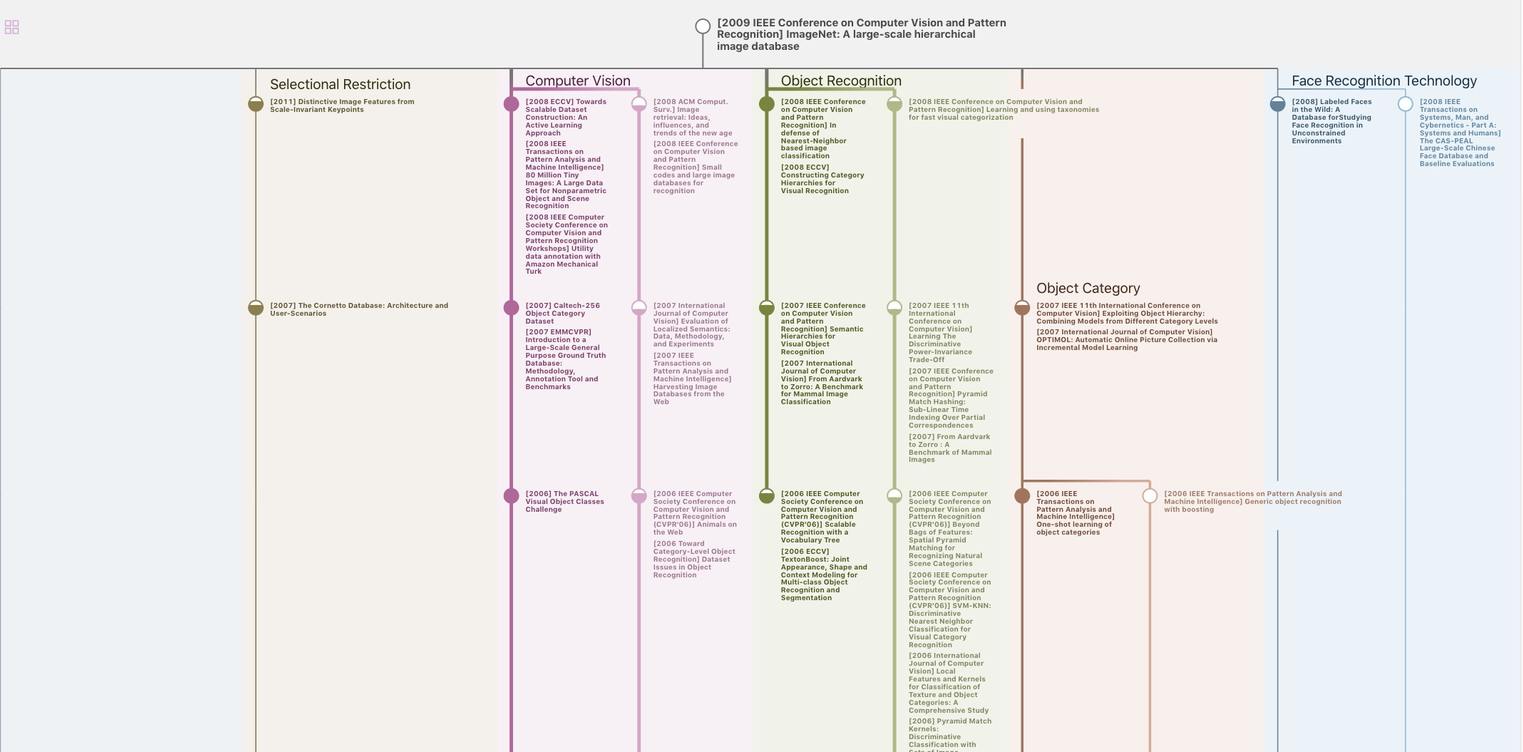
Generate MRT to find the research sequence of this paper
Chat Paper
Summary is being generated by the instructions you defined