Dual-Tracer PET Image Separation by Deep Learning: A Simulation Study
Applied Sciences(2023)
摘要
Multiplexed positron emission tomography (PET) imaging provides perfectly registered simultaneous functional and molecular imaging of more than one biomarker. However, the separation of the multiplexed PET signals within a single PET scan is challenging due to the fact that all PET tracers emit positrons, which, after annihilating with a nearby electron, give rise to 511 keV photon pairs that are detected in coincidence. Compartment modelling can separate single-tracer PET signals from multiplexed signals based on the differences in bio-distribution kinetics and radioactive decay. However, the compartment-modelling-based method requires staggered injections and assumes that each tracer's input function is known. In this paper, we propose a deep-learning-based method to simultaneously separate dual-tracer PET signals without explicitly knowing the input functions. We evaluate the proposed deep-learning-based separation method on dual-tracer [F-18]FDG and [C-11]MET PET simulations and compare its separation performance to that of the compartment-modelling-based method, assessing performance dependence on the time interval between tracer injections as well as on the amount of training data. It is shown that the proposed method implicitly denoises the separated images and offers reduced variance in the separated images compared to compartment modelling.
更多查看译文
关键词
multiplexed PET imaging,dual-tracer separation,deep learning,convolutional encoder-decoder,kinetic modelling,PET image reconstruction
AI 理解论文
溯源树
样例
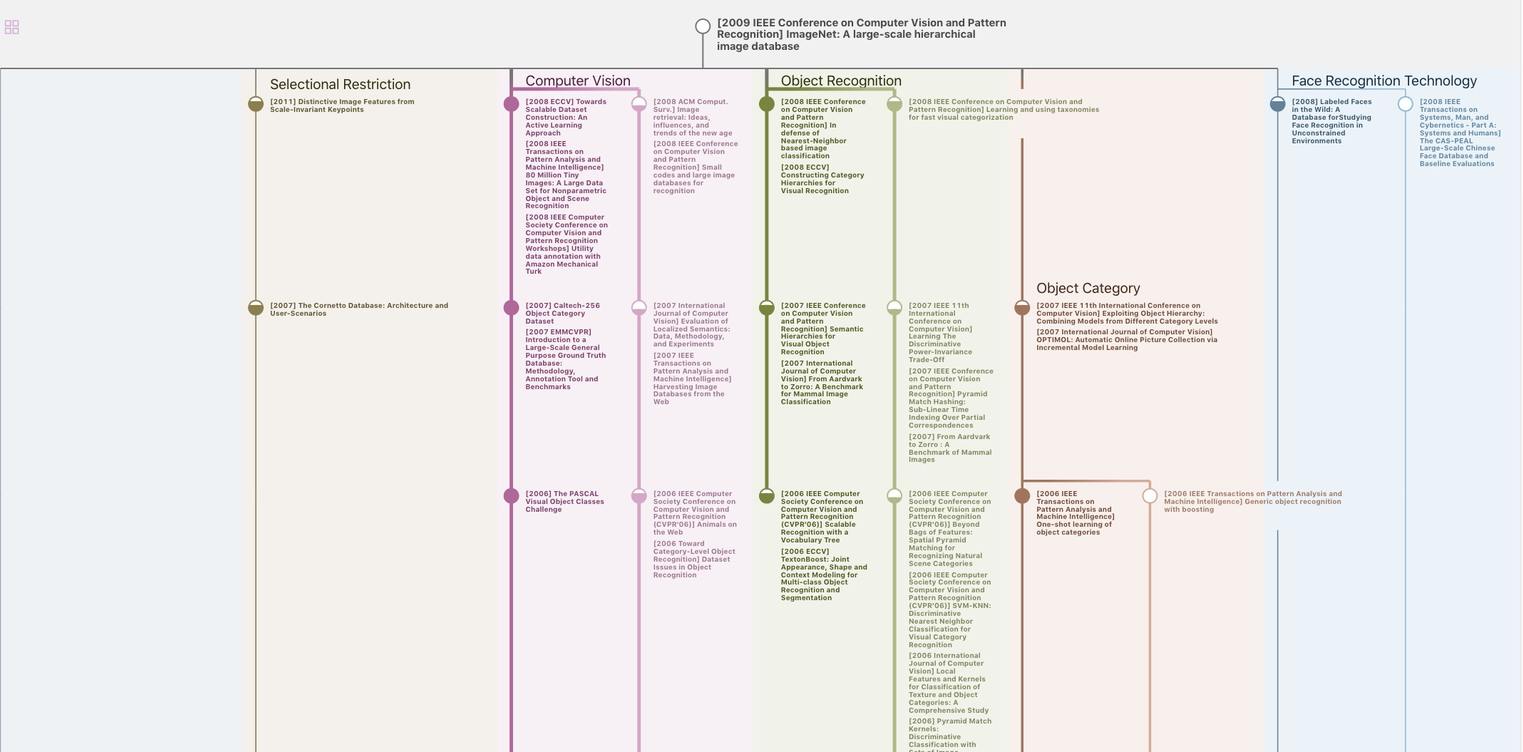
生成溯源树,研究论文发展脉络
Chat Paper
正在生成论文摘要