Deep Learning for Improved Subsurface Imaging: Enhancing GPR Clutter Removal Performance Using Contextual Feature Fusion and Enhanced Spatial Attention
Remote Sensing(2023)
摘要
In engineering practice, ground penetrating radar (GPR) records are often hindered by clutter resulting from uneven underground media distribution, affecting target signal characteristics and precise positioning. To address this issue, we propose a method combining deep learning preprocessing and reverse time migration (RTM) imaging. Our preprocessing approach introduces a novel deep learning framework for GPR clutter, enhancing the network’s feature-capture capability for target signals through the integration of a contextual feature fusion module (CFFM) and an enhanced spatial attention module (ESAM). The superiority and effectiveness of our algorithm are demonstrated by RTM imaging comparisons using synthetic and laboratory data. The processing of actual road data further confirms the algorithm’s significant potential for practical engineering applications.
更多查看译文
关键词
ground-penetrating radar (GPR),contextual feature fusion module (CFFM),enhanced spatial attention module (ESAM),clutter removal,reverse time migration (RTM)
AI 理解论文
溯源树
样例
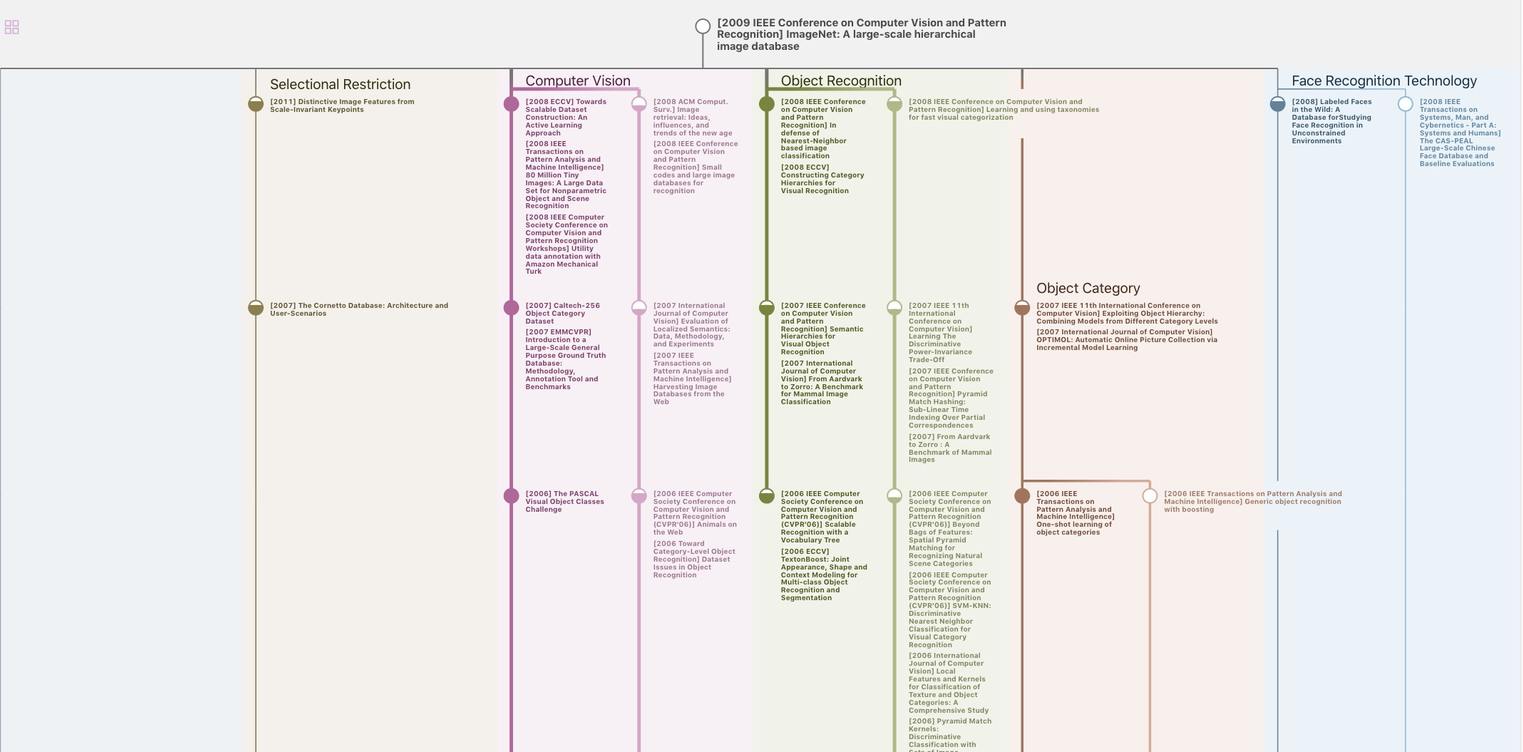
生成溯源树,研究论文发展脉络
Chat Paper
正在生成论文摘要