Human-Aware Collaborative Robots in the Wild: Coping with Uncertainty in Activity Recognition
Sensors(2023)
摘要
This study presents a novel approach to cope with the human behaviour uncertainty during Human-Robot Collaboration (HRC) in dynamic and unstructured environments, such as agriculture, forestry, and construction. These challenging tasks, which often require excessive time, labour and are hazardous for humans, provide ample room for improvement through collaboration with robots. However, the integration of humans in-the-loop raises open challenges due to the uncertainty that comes with the ambiguous nature of human behaviour. Such uncertainty makes it difficult to represent high-level human behaviour based on low-level sensory input data. The proposed Fuzzy State-Long Short-Term Memory (FS-LSTM) approach addresses this challenge by fuzzifying ambiguous sensory data and developing a combined activity recognition and sequence modelling system using state machines and the LSTM deep learning method. The evaluation process compares the traditional LSTM approach with raw sensory data inputs, a Fuzzy-LSTM approach with fuzzified inputs, and the proposed FS-LSTM approach. The results show that the use of fuzzified inputs significantly improves accuracy compared to traditional LSTM, and, while the fuzzy state machine approach provides similar results than the fuzzy one, it offers the added benefits of ensuring feasible transitions between activities with improved computational efficiency.
更多查看译文
关键词
human activity recognition and modelling,deep learning,human-robot collaboration,fuzzy logic,finite state machine,long short—term memory
AI 理解论文
溯源树
样例
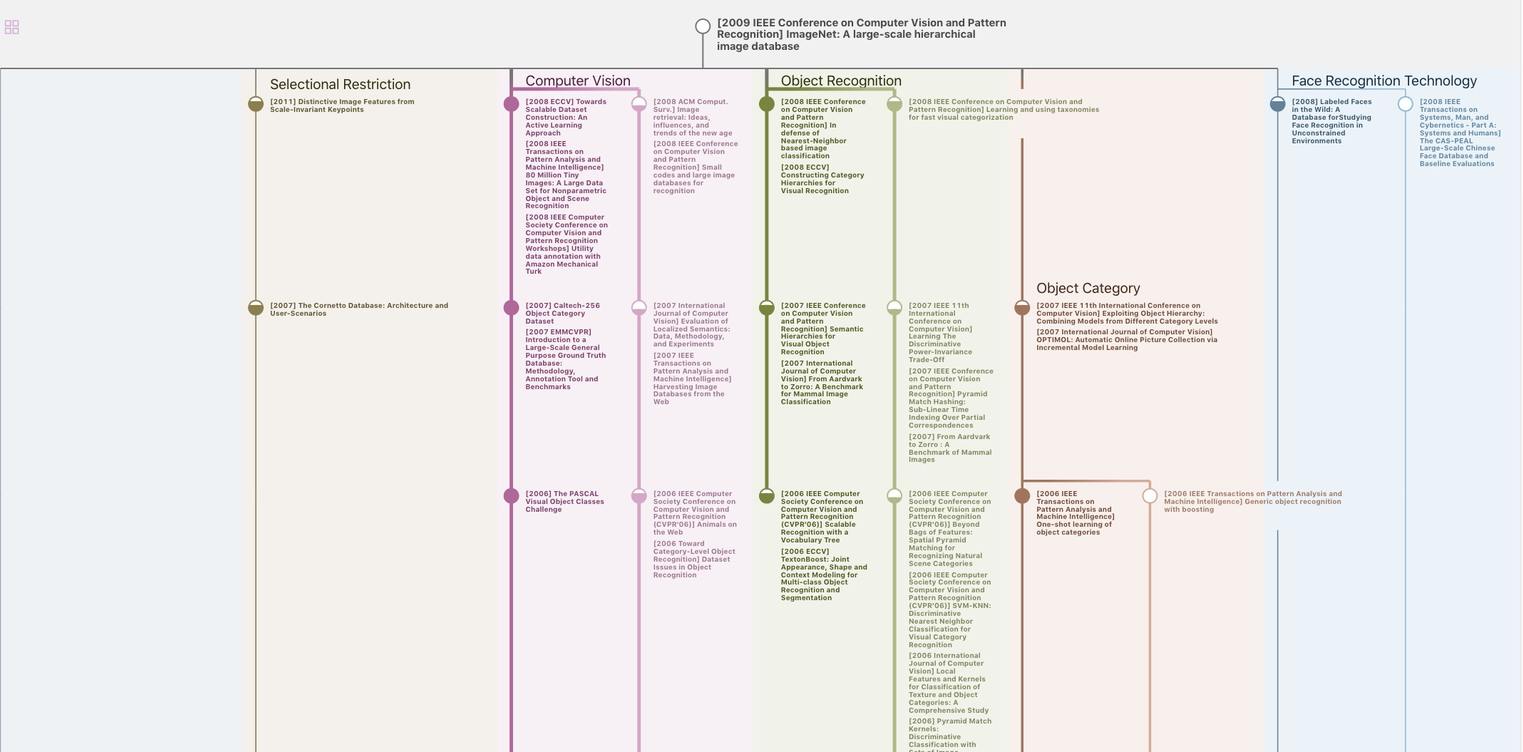
生成溯源树,研究论文发展脉络
Chat Paper
正在生成论文摘要