Inner Approximations of Stochastic Programs for Data-driven Stochastic Barrier Function Design
2023 62ND IEEE CONFERENCE ON DECISION AND CONTROL, CDC(2023)
摘要
This paper proposes a new framework to compute finite-horizon safety guarantees for discrete-time piece-wise affine systems with stochastic noise of unknown distributions. The approach is based on a novel approach to synthesise a stochastic barrier function (SBF) from noisy data and rely on the scenario optimization theory. In particular, we show that the stochastic program to synthesize a SBF can be relaxed into a chance-constrained optimisation problem on which scenario approach theory applies. We further show that the resulting program can be reduced to a linear programming problem, thus guaranteeing efficiency. In contrast to existing approaches, this method is data efficient as it only requires the number of data to be proportional to the logarithm in the negative inverse of the confidence level and is computationally efficient due to its reduction to linear programming. The efficacy of the method is empirically evaluated on various verification benchmarks. Experiments show a significant improvement with respect to state-of-the-art, obtaining tighter certificates with a confidence that is several orders of magnitude higher.
更多查看译文
关键词
stochastic programs,inner approximations,data-driven
AI 理解论文
溯源树
样例
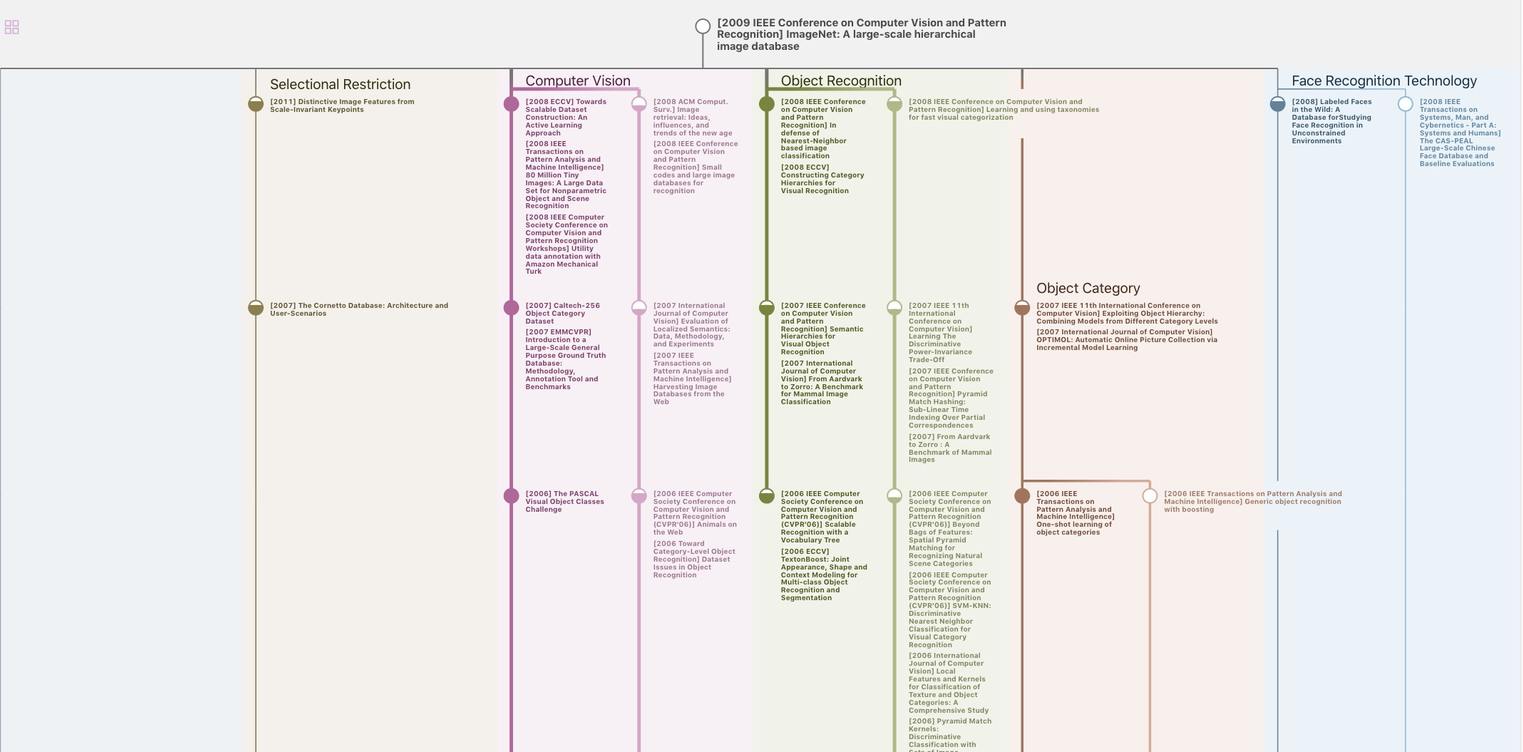
生成溯源树,研究论文发展脉络
Chat Paper
正在生成论文摘要