Revisiting Activation Function Design for Improving Adversarial Robustness at Scale
ICLR 2023(2023)
摘要
Modern ConvNets typically use ReLU activation function. Recently smooth activation functions have been used to improve their accuracy. Here we study the role of smooth activation function from the perspective of adversarial robustness. We find that ReLU activation function significantly weakens adversarial training due to its non-smooth nature. Replacing ReLU with its smooth alternatives allows adversarial training to find harder adversarial training examples and to compute better gradient updates for network optimization.
We focus our study on the large-scale ImageNet dataset. On ResNet-50, switching from ReLU to the smooth activation function SILU improves adversarial robustness from 33.0% to 42.3%, while also improving accuracy by 0.9% on ImageNet. Smooth activation functions also scale well with larger networks: it helps EfficientNet-L1 to achieve 82.2% accuracy and 58.6% robustness, largely outperforming the previous state-of-the-art defense by 9.5% for accuracy and 11.6% for robustness. Models are available at https://rb.gy/qt8jya.
更多查看译文
关键词
adversarial training,activation function,neural network architecture
AI 理解论文
溯源树
样例
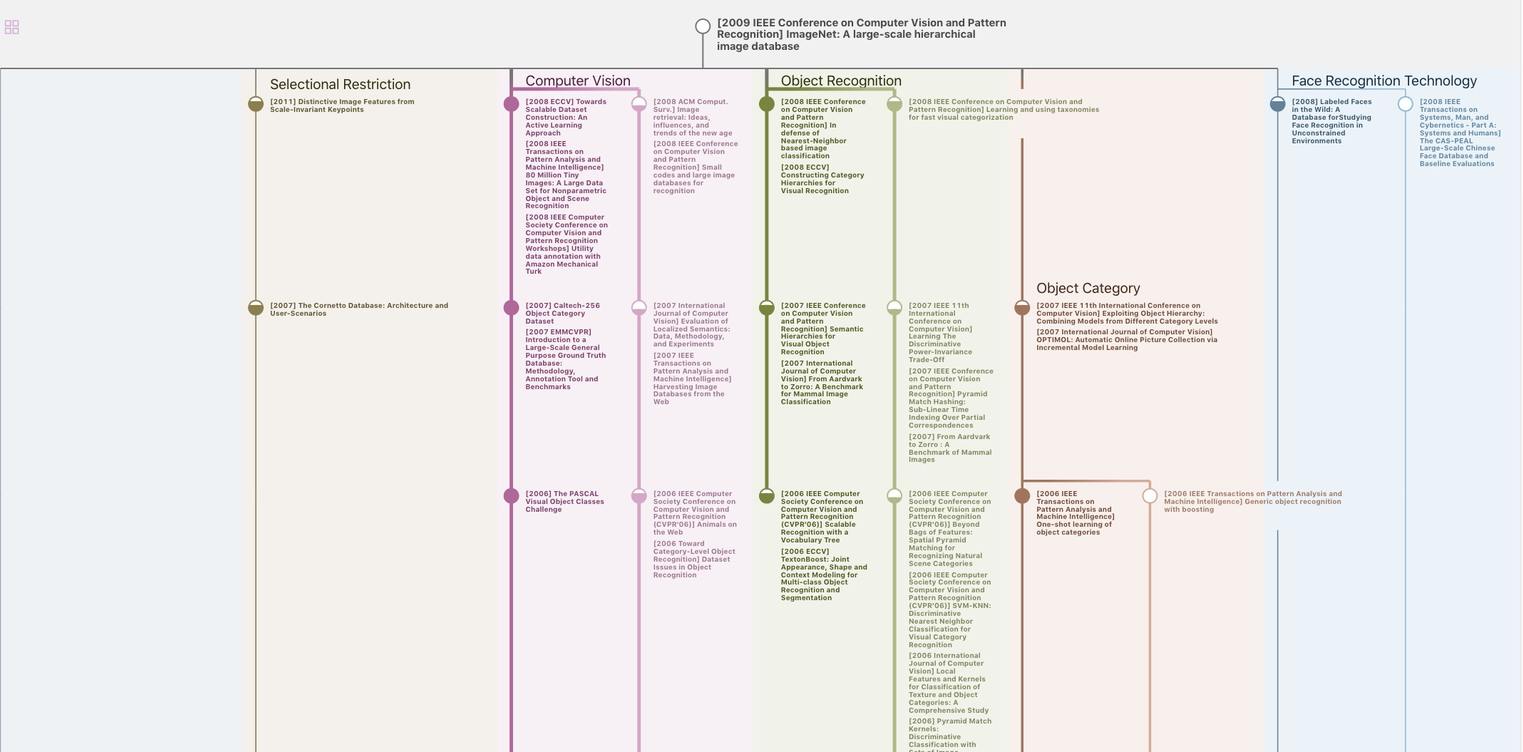
生成溯源树,研究论文发展脉络
Chat Paper
正在生成论文摘要