What Deep Representations Should We Learn? -- A Neural Collapse Perspective
ICLR 2023(2023)
摘要
For classification problems, when sufficiently large networks are trained until convergence, an intriguing phenomenon has recently been discovered in the last-layer classifiers, and features termed neural collapse (NC): (i) the intra-class variability of the features collapses to zero, and (ii) the between-class feature means are maximally and equally separated. Despite of recent endeavors to understand why NC happens, a fundamental question remains: whether NC is a blessing or a curse for deep learning? In this work, we investigate the problem under the setting of transfer learning that we pretrain a model on a large dataset and transfer it to downstream tasks. Through various experiments, our findings on NC are two-fold: (i) when pretrain models, preventing intra-class variability collapse (to a certain extent) better preserve the structures of data, and leads to better model transferability; (ii) when fine-tuning models on downstream tasks, obtaining features with more NC on downstream data results in better test accuracy on the given task. Our findings based upon NC not only explain many widely used heuristics in model pretraining (e.g., data augmentation, projection head, self-supervised learning), but also leads to more efficient and principled transfer learning method on downstream tasks.
更多查看译文
关键词
representation learning,neural collapse,transfer learning
AI 理解论文
溯源树
样例
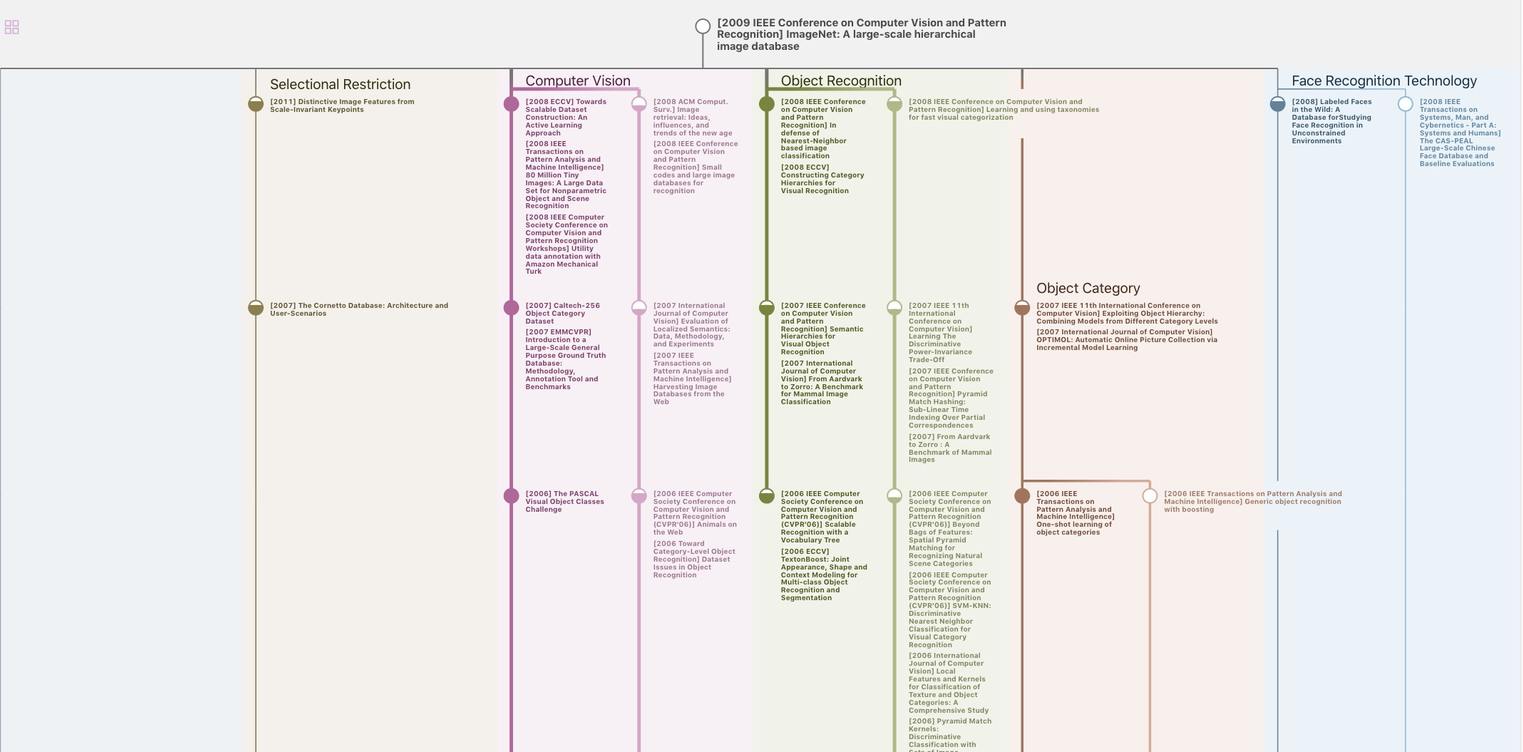
生成溯源树,研究论文发展脉络
Chat Paper
正在生成论文摘要