Certifiably Robust Transformers with 1-Lipschitz Self-Attention
ICLR 2023(2023)
摘要
Recent works have shown that neural networks with Lipschitz constraints will lead to high adversarial robustness. In this work, we propose the first One-Lipschitz Self-Attention (OLSA) mechanism for Transformer models. In particular, we first orthogonalize all the linear operations in the self-attention mechanism. We then bound the overall Lipschitz constant by aggregating the Lipschitz of each element in the softmax with weighted sum. Based on the proposed self-attention mechanism, we construct an OLSA Transformer to achieve model deterministic certified robustness. We evaluate our model on multiple natural language processing (NLP) tasks and show that it outperforms existing certification on Transformers, especially for models with multiple layers. As an example, for 3-layer Transformers we achieve an ℓ2 deterministic certified robustness radius of 1.733 and 0.979 on the word embedding space for the Yelp and SST dataset, while the existing SOTA certification baseline of the same embedding space can only achieve 0.061 and 0.110. In addition, our certification is significantly more efficient than previous works, since we only need the output logits and Lipschitz constant for certification. We also fine-tune our OLSA Transformer as a downstream classifier of a pre-trained BERT model and show that it achieves significantly higher certified robustness on BERT embedding space compared with previous works (e.g. from 0.071 to 0.368 on the QQP datasets).
更多查看译文
关键词
Certified robustness,Transformers
AI 理解论文
溯源树
样例
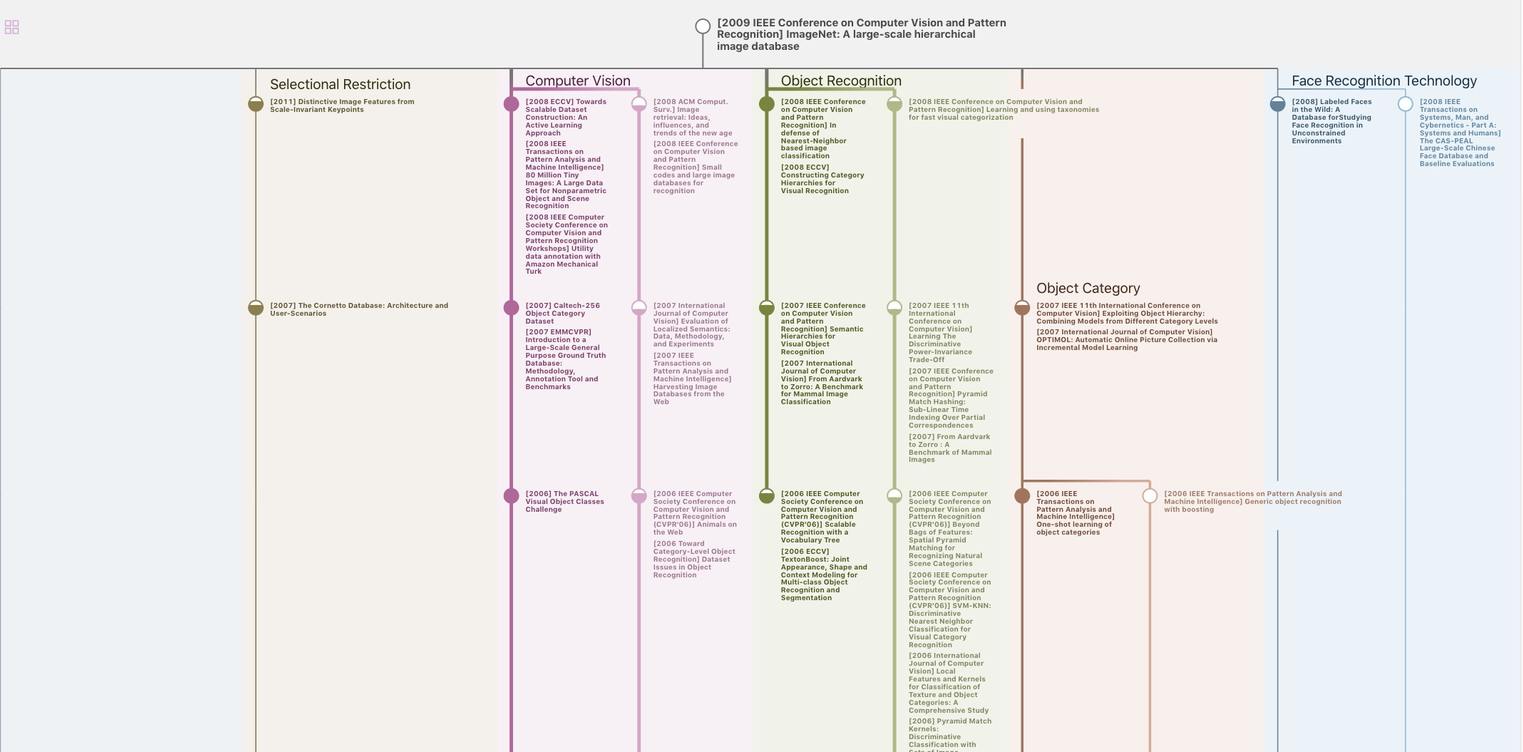
生成溯源树,研究论文发展脉络
Chat Paper
正在生成论文摘要