Latent Linear ODEs with Neural Kalman Filtering for Irregular Time Series Forecasting
ICLR 2023(2023)
摘要
Over the past four years, models based on Neural Ordinary Differential Equations have become state of the art in the forecasting of irregularly sampled time series. Describing the data-generating process as a dynamical system in continuous time allows predictions at arbitrary time points. However, the numerical integration of Neural ODEs typically comes with a high computational burden or may even fail completely. We propose a novel Neural ODE model that embeds the observations into a latent space with dynamics governed by a linear ODE. Consequently, we do not require any specialized numerical integrator but only an implementation of the matrix exponential readily available in many numerical linear algebra libraries. We also introduce a novel state update component inspired by the classical Kalman filter, which, to our knowledge, makes our model the first Neural ODE variant to explicitly satisfy a specific self-consistency property. It allows forecasting irregularly sampled time series with missing values and comes with some numerical stability guarantees. We evaluate the performance on medical and climate benchmark datasets, where the model outperforms the state of the art by margins up to 30%.
更多查看译文
关键词
Time Series Forecasting,Neural ODE,Kalman Filter,Koopman Operator,Missing Values
AI 理解论文
溯源树
样例
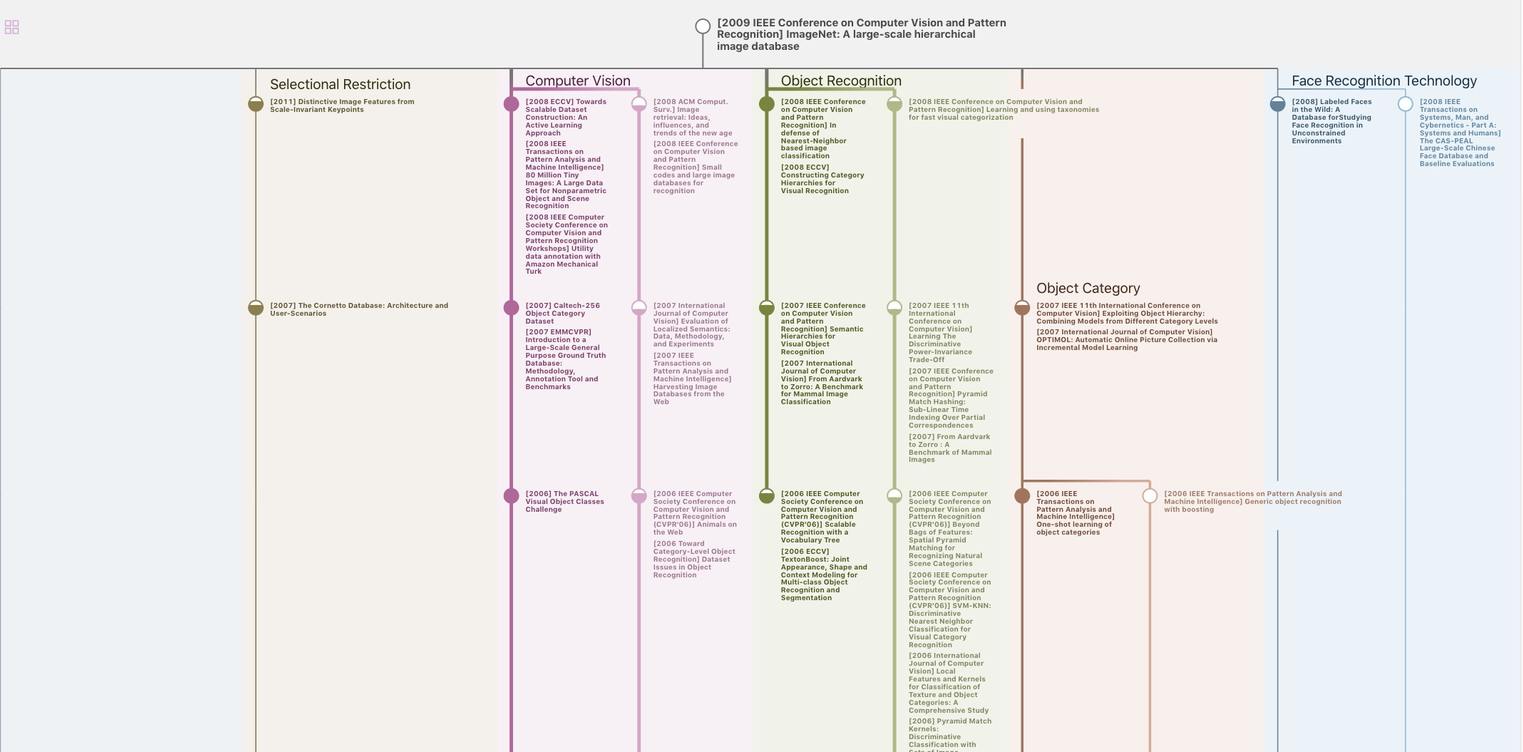
生成溯源树,研究论文发展脉络
Chat Paper
正在生成论文摘要