A Survey on Traditional and Deep Learning Copy Move Forgery Detection (CMFD) Techniques
Multimedia tools and applications(2023)
摘要
Digital image forgeries become one of the most challenges faced by technology users today. A copy-move forgery is one of the most common types of these forgeries. This type is not only common, but it is also very simple to execute to create deceptive images. Many algorithms use various technologies to create a high-performance Copy Move Forgery Detection (CMFD) system. There are currently two major CMFD trends: traditional CMFD algorithms and deep-learning-based CMFD algorithms. The main concept behind CMFD algorithms is to extract image features using various techniques and then search for matching features. This main idea can be carried out by using different feature extraction techniques with various matching techniques. For traditional algorithms, there are many types of features extractors used such as invariant image moments, texture and intensity descriptors, Scale-Invariant Feature Transform (SIFT), Speed Up Roust Features (SURF), Discrete Cosine Transform (DCT), Singular Value Decomposition (SVD), Principal Component Analysis (PCA), and other extractors. The strength of these extractors varies according to the image properties and the forgery’s performance perfection. Deep learning-based CMFD algorithms operate as a black box, by extracting image features using layers with complex training and validation processes to achieve the best scenario, such as Convolutional Neural Network (CNN), Convolutional Long Short-Term Memory (CovLSTM), Multi-domain CNN, and Buster-Net. This paper discusses different types of digital image forgery with focusing on copy-move forgery. A detailed survey of various types of CMFD algorithms, on the other hand, has been displayed. The paper provides a comprehensive comparison of various algorithms and evaluates them using various types of evaluation parameters. Finally, the paper focuses on the different datasets used by various algorithms to evaluate their performance accuracy and testing time. These datasets are detailed in terms of image numbers, the number of original images, the number of forged images, the resolution variety in each dataset, the size of the tampered region in each dataset, and the strength of each dataset compared with each other.
更多查看译文
关键词
Copy move forgery detection,SIFT,SURF,CNN,CovLSTM,Multi-domain CNN,Buster-net
AI 理解论文
溯源树
样例
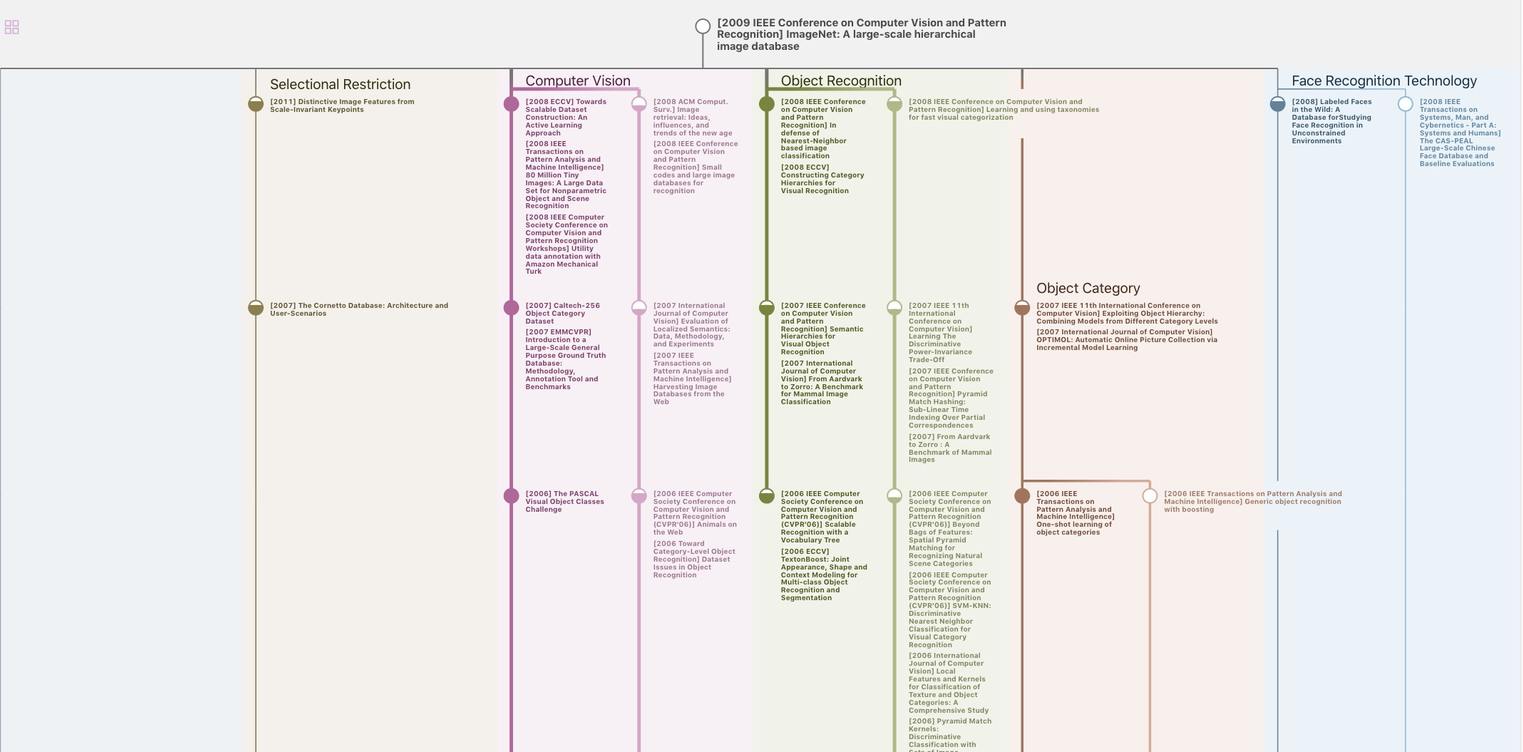
生成溯源树,研究论文发展脉络
Chat Paper
正在生成论文摘要