A New Approach for Super-Resolution and Classification Applications on Neonatal Thermal Images
Quantitative infra red thermography journal/Quantitative infrared thermography(2023)
摘要
Thermal imaging systems are harmless to human health and enable contactless heat measurements. The thermal cameras are used in many public sectors where it is necessary to detect the change of temperature values. However, thermal cameras are costly and produce images with low edge information. This situation prevents the widespread use of thermal cameras. Therefore, in recent years, the researches to advance the quality of thermal images have increased. Within the scope of the studies in this paper, first of all, three different datasets consisting of thermal images in the colourful format of neonates were created. Also, TSRGAN+ deep network model was presented for super-resolution studies. The super-resolution images obtained visually approached ground truth images to a great extent. In addition, these results were compared using the peak signal to noise ratio (PSNR) and the structural similarity index measure (SSIM) image quality metrics. The proposed model showed a superior success in terms of the values of PSNR and SSIM compared to the state-of-the-art models. Here, the PSNR value of the proposed TSRGAN+ model increased by 1-1.5 dB compared to the TSRGAN network architecture, while the SSIM value increased by around 2-3%. Finally, the unhealthy-healthy image classification applications were performed on all thermal image sets in order to implement both the task-based evaluation and a real-life application. Thus, a new method is presented to evaluate the results of the super-resolution studies. Here, firstly, a CNN-based classifier was designed and the classification metrics were obtained for all three datasets. Then, transfer learning was applied using state-of-the-art models (ResNet101, Xception) to increase classification success. Here, the most successful results were obtained in applications using the ResNet101 model. Also, the developed model outperformed the TSRGAN, which achieved the second-best result. When all the obtained results are evaluated, it has been observed that the super-resolution models increase the success of unhealthy-healthy classification by about 10% compared to the low resolution images. In other words, the effects of super-resolution techniques on classification applications are clearly seen. In summary, the logical use of the super-resolution research will enable the common use of low-cost thermal cameras in the application fields such as medicine.
更多查看译文
关键词
Thermal image processing,deep learning models,super-resolution,image classification,transfer learning
AI 理解论文
溯源树
样例
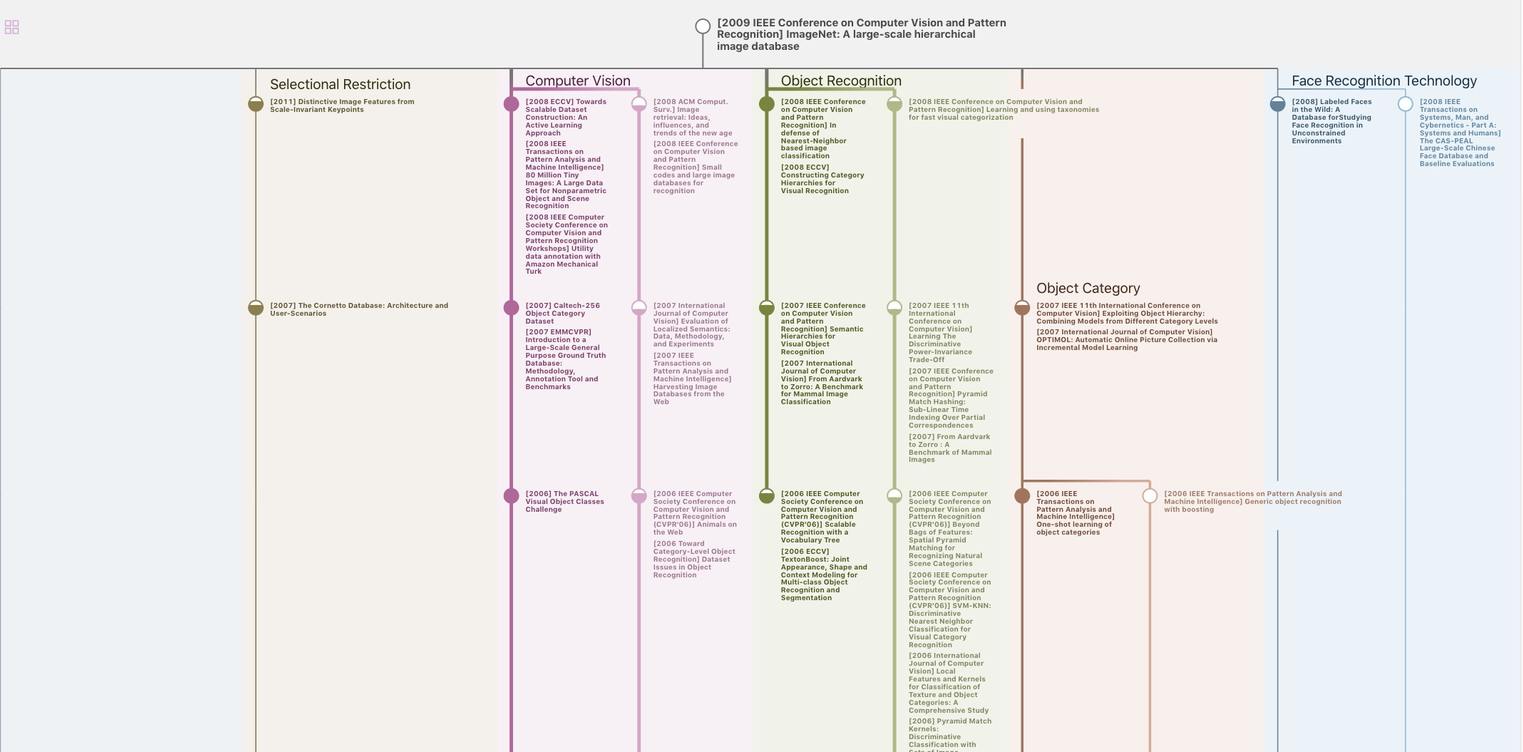
生成溯源树,研究论文发展脉络
Chat Paper
正在生成论文摘要