A transformer with layer-cross decoding for remaining useful life prediction
JOURNAL OF SUPERCOMPUTING(2023)
Abstract
Remaining useful life (RUL) prediction is critical for industrial equipment status detection, and the accurate prediction results provide decision-makers with actionable information. Preventive maintenance can be carried out to prevent the sudden failure of the equipment effectively based on the predicted results. However, as Industry 4.0 technologies develop, the amount of data collected by sensors is also rapidly increasing. The existing RUL prediction methods are gradually unable to cope with complex industrial equipment data, and deep learning methods gradually come to the fore. In this background, this paper proposes a transformer-based model with a multi-layer encoder–decoder structure to extract domain-invariant features. The decoder in the traditional transformer structure only obtains a single piece of information from the last layer of the encoder, and this paper uses an integrated layer-cross decoding strategy to compensate. Based on the encoder–decoder cross-connection, each decoder layer is provided with global view information from the final encoder layer simultaneously, improving the model’s performance. The validity and superiorities of the proposed method are evaluated through several experiments on the publicly available C-MAPSS dataset provided by NASA. As can be seen from the results, the proposed method gets higher prediction accuracy than other network architectures and state-of-the-art approaches.
MoreTranslated text
Key words
Remaining useful life prediction,Deep learning,Transformer,Layer-cross decoding,Prognostics
AI Read Science
Must-Reading Tree
Example
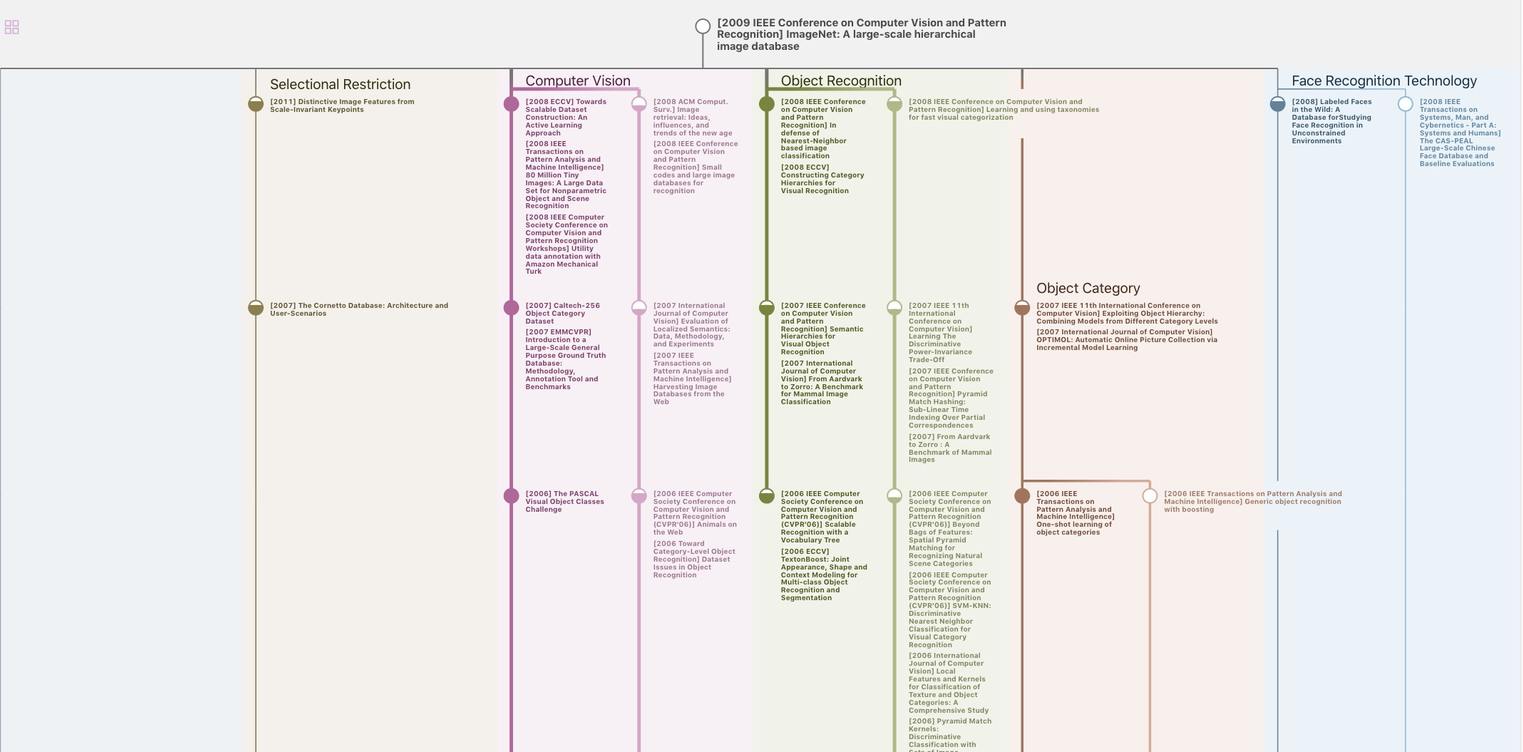
Generate MRT to find the research sequence of this paper
Chat Paper
Summary is being generated by the instructions you defined