Efficient feature selection using BoWs and SURF method for leaf disease identification
MULTIMEDIA TOOLS AND APPLICATIONS(2023)
Abstract
When plant diseases appear, they have an adverse effect on agricultural output. Food insecurity would worsen if plant diseases were not identified accurately in time. Without early identification of plant diseases, agricultural production management and decision-making would not be feasible. Now a days deep learning model is widely used for image classification to get more accurate result. But machine learning based classifier also produces good result if good feature selection technique is used. In this paper, we have used hybrid machine learning techniques for classifying leaf diseases present in species such as tomato, potato and pepper bell. We have used bag-of- feature for visually representing diseased leaf features. SURF technique is used to extract strongest number of features and for classification task SVM is used. The proposed method gives good result in terms of precision, accuracy, recall, F1-score, FPR, FNR, and MCC on all the three employed datasets. The classification accuracy obtained in our proposed model on dataset1, dataset2 and dataset3 are 97%, 97% and 93% respectively. We have also calculated percentage feature reduction for all three types of species which are approximately 45.21%, 40.65% and 34.73% for tomato, potato and pepper bell respectively. We have compared disease classification accuracy of several previous work done by various authors on tomato leaf dataset using various machine learning and deep learning technique with our proposed work and find that our method is performing better.
MoreTranslated text
Key words
Leaf disease,BoWs,SURF,K-means,Features,SVM
AI Read Science
Must-Reading Tree
Example
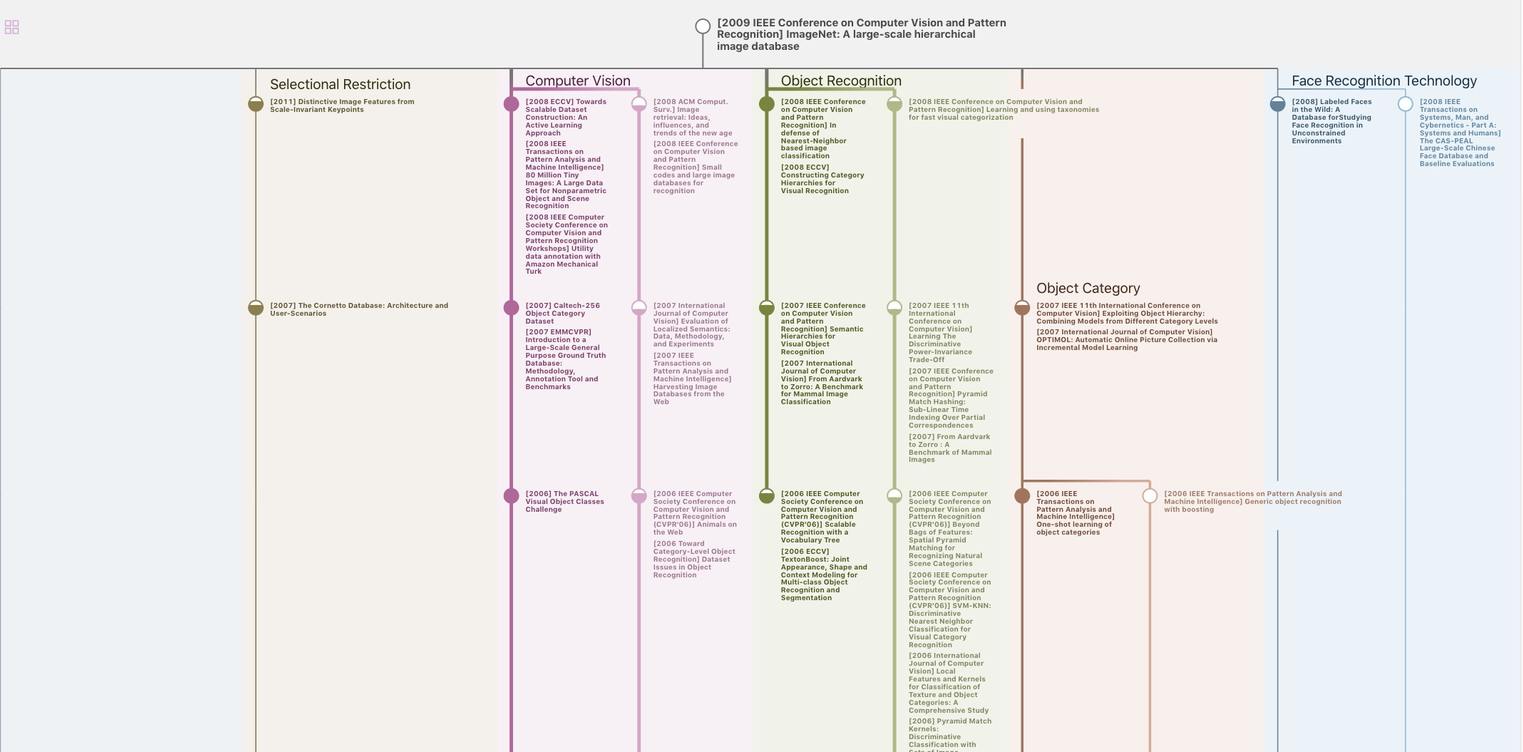
Generate MRT to find the research sequence of this paper
Chat Paper
Summary is being generated by the instructions you defined