A measurement error prediction framework for smart meters under extreme natural environment stresses
ELECTRIC POWER SYSTEMS RESEARCH(2023)
Abstract
Accurate prediction of measurement errors is essential for the operational status assessment of smart meters. So far, however, there has been little discussion on the prediction of performance metrics for instruments under extreme natural environmental stress. So, we propose a new measurement error prediction framework in the paper. First, the Weighted Principal Component Analysis (WPCA) is used to convert environmental factors with correlations into an environmental comprehensive index to simplify the structure of our prediction model. Second, a new Multiple Adaptive Genetic Algorithm (MAGA) is proposed based on individual fitness and iter-ation times to optimize the initial weights and thresholds of the Back Propagation Neural Network (BPNN). A new measurement error prediction model, namely MAGA-BPNN, is then given based on MAGA and BPNN. Be-sides, the prediction residuals of MAGA-BPNN are corrected using Auto-Regressive Moving Average (ARMA). Finally, the prediction framework composed of MAGA-BPNN and ARMA is experimentally validated using real -world data from a high-dry-heat area. The mean absolute error of predictions is 3.80e-3, and the mean square error is 3.29e-5. The results show that our framework has excellent prediction ability and generalization ability. It can provide a basis for device maintenance and replacement, especially under extreme natural environmental stresses.
MoreTranslated text
Key words
Smart meters,Measurement error,Natural environment stresses,Multiple adaptive genetic algorithm,Residual correction
AI Read Science
Must-Reading Tree
Example
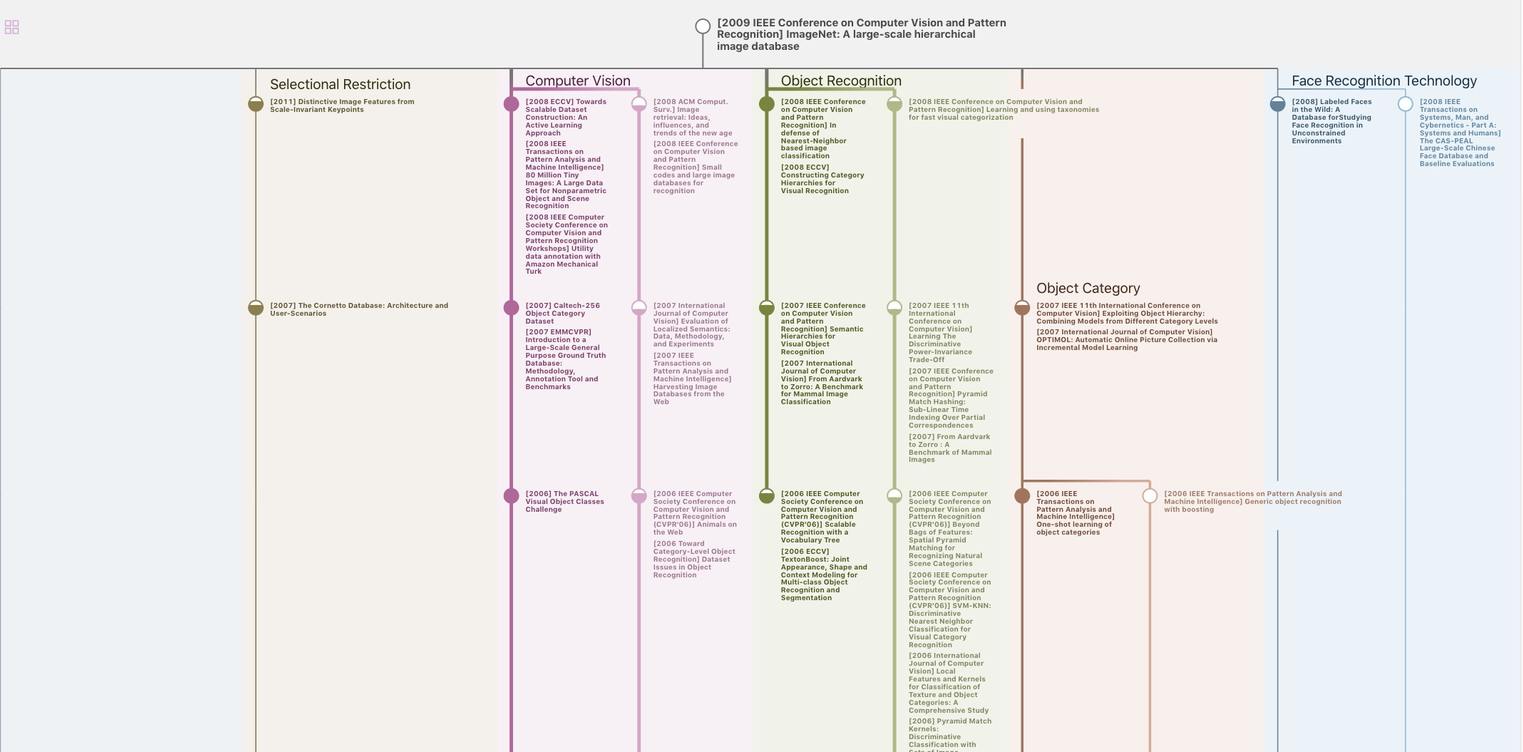
Generate MRT to find the research sequence of this paper
Chat Paper
Summary is being generated by the instructions you defined