Attention Knowledge Network Combining Explicit and Implicit Information
MATHEMATICS(2023)
摘要
The existing knowledge graph embedding (KGE) method has achieved good performance in recommendation systems. However, the relevancy degree among entities reduces gradually along the spread in the knowledge graph. Focusing on the explicit and implicit relationships among entities, this paper proposes an attention knowledge network combining explicit and implicit information (AKNEI) to effectively capture and exactly describe the correlation between entities in the knowledge graph. First, we design an information-sharing layer (ISL) to realize information sharing between projects and entities through implicit interaction. We innovatively propose a cross-feature fusion module to extract high-order feature information in the model. At the same time, this paper uses the attention mechanism to solve the problem of the decline of information relevance in the process of knowledge graph propagation. Finally, the features of KGE and cross feature fusion module are integrated into the end-to-end learning framework, the item information in the recommendation task and the knowledge graph entity information are interacted implicitly and explicitly, and the characteristics between them are automatically learned. We performed extensive experiments on multiple public datasets that include movies, music, and books. According to the experimental results, our model has a great improvement in performance compared with the latest baseline.
更多查看译文
关键词
recommendation system,knowledge graph embedding,multi-task learning,attention mechanism
AI 理解论文
溯源树
样例
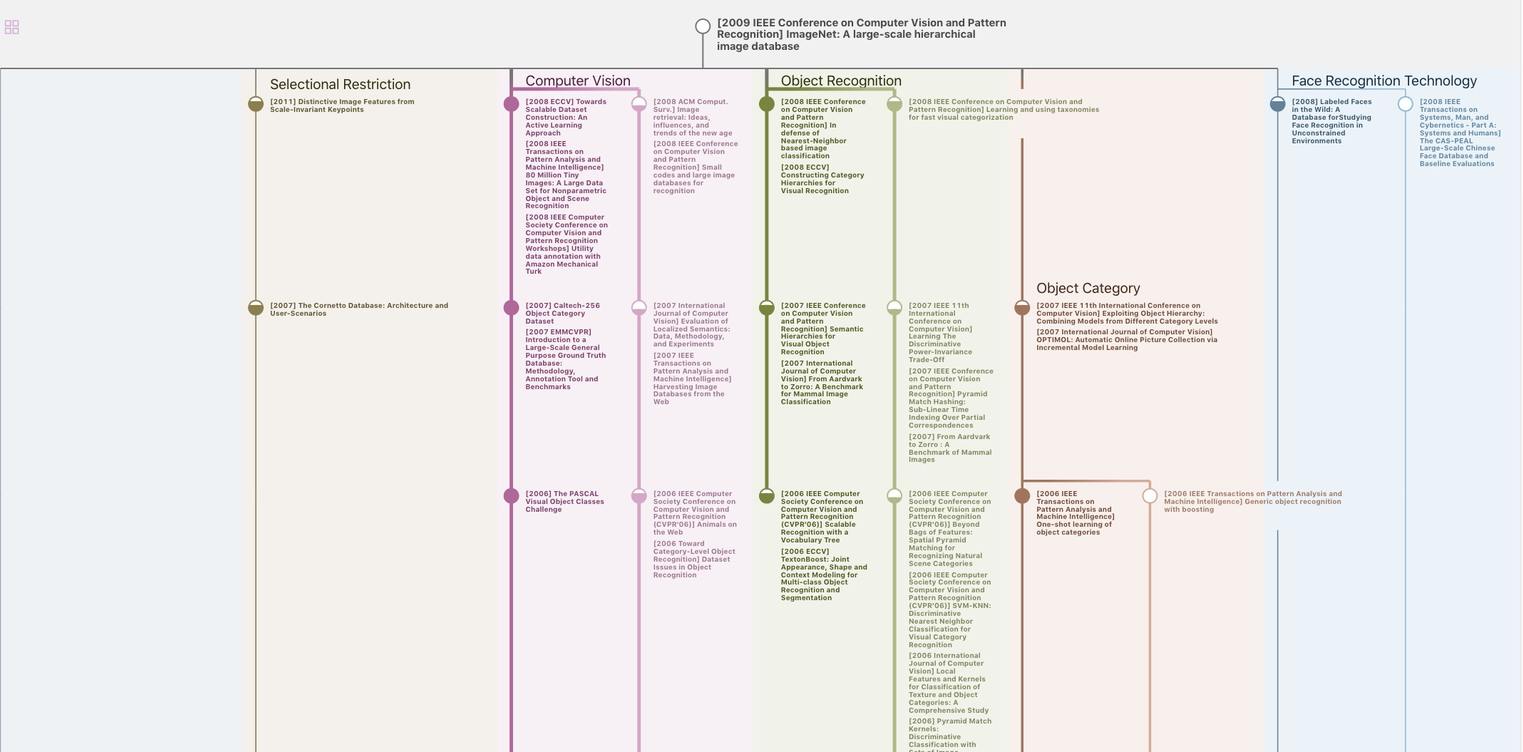
生成溯源树,研究论文发展脉络
Chat Paper
正在生成论文摘要