Machine Learning-Aided Band Gap Engineering of BaZrS3 Chalcogenide Perovskite
ACS applied materials & interfaces(2023)
摘要
The non-toxic and stable chalcogenide perovskite BaZrS3 fulfills many key optoelectronic properties for a high-efficiency photovoltaic material. It has been shown to possess a direct band gap with a large absorption coefficient and good carrier mobility values. With a reported band gap of 1.7-1.8 eV, BaZrS3 is a good candidate for tandem solar cell materials; however, its band gap is significantly larger than the optimal value for a high-efficiency single-junction solar cell (similar to 1.3 eV, Shockley-Queisser limit)thus doping is required to lower the band gap. By combining first-principles calculations and machine learning algorithms, we are able to identify and predict the best dopants for the BaZrS3 perovskites for potential future photovoltaic devices with a band gap within the Shockley- Queisser limit. It is found that the Ca dopant at the Ba site or Ti dopant at the Zr site is the best candidate dopant. Based on this information, we report for the first time partial doping at the Ba site in BaZrS3 with Ca (i.e., Ba1-xCaxZrS3) and compare its photoluminescence with Ti-doped perovskites [i.e., Ba(Zr1-xTix)S-3]. Synthesized (Ba,Ca)ZrS3 perovskites show a reduction in the band gap from similar to 1.75 to similar to 1.26 eV with <2 atom % Ca doping. Our results indicate that for the purpose of band gap tuning for photovoltaic applications, Ca-doping at the Ba-site is superior to Ti-doping at the Zr-site reported previously.
更多查看译文
关键词
BaZrS3 films,band gap engineering,machine learning,random forest regression,density functional theory
AI 理解论文
溯源树
样例
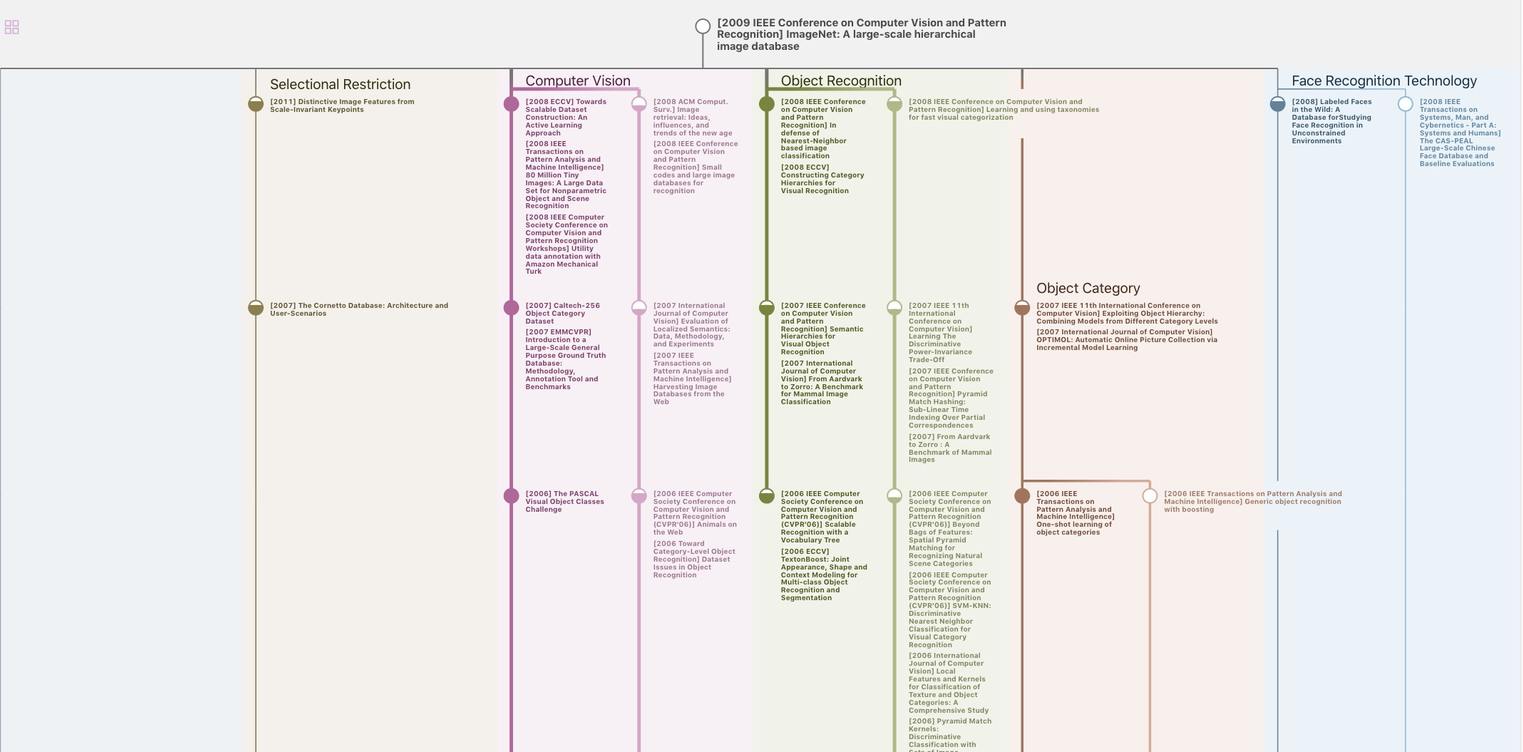
生成溯源树,研究论文发展脉络
Chat Paper
正在生成论文摘要