A Decision Support System Based on Artificial Intelligence and Systems Biology for the Simulation of Pancreatic Cancer Patient Status
CPT-PHARMACOMETRICS & SYSTEMS PHARMACOLOGY(2023)
Anaxom Biotech SL | Iteraset Solut SL | Univ Pompeu Fabra | Univ Autonoma Barcelona | Hosp La Mancha Ctr
Abstract
Oncology treatments require continuous individual adjustment based on the measurement of multiple clinical parameters. Prediction tools exploiting the patterns present in the clinical data could be used to assist decision making and ease the burden associated to the interpretation of all these parameters. The goal of this study was to predict the evolution of patients with pancreatic cancer at their next visit using information routinely recorded in health records, providing a decision-support system for clinicians. We selected hematological variables as the visit's clinical outcomes, under the assumption that they can be predictive of the evolution of the patient. Multivariate models based on regression trees were generated to predict next-visit values for each of the clinical outcomes selected, based on the longitudinal clinical data as well as on molecular data sets streaming from in silico simulations of individual patient status at each visit. The models predict, with a mean prediction score (balanced accuracy) of 0.79, the evolution trends of eosinophils, leukocytes, monocytes, and platelets. Time span between visits and neutropenia were among the most common factors contributing to the predicted evolution. The inclusion of molecular variables from the systems-biology in silico simulations provided a molecular background for the observed variations in the selected outcome variables, mostly in relation to the regulation of hematopoiesis. In spite of its limitations, this study serves as a proof of concept for the application of next-visit prediction tools in real-world settings, even when available data sets are small.
MoreTranslated text
Key words
Tumor Microenvironment,Spatial Profiling
求助PDF
上传PDF
View via Publisher
AI Read Science
AI Summary
AI Summary is the key point extracted automatically understanding the full text of the paper, including the background, methods, results, conclusions, icons and other key content, so that you can get the outline of the paper at a glance.
Example
Background
Key content
Introduction
Methods
Results
Related work
Fund
Key content
- Pretraining has recently greatly promoted the development of natural language processing (NLP)
- We show that M6 outperforms the baselines in multimodal downstream tasks, and the large M6 with 10 parameters can reach a better performance
- We propose a method called M6 that is able to process information of multiple modalities and perform both single-modal and cross-modal understanding and generation
- The model is scaled to large model with 10 billion parameters with sophisticated deployment, and the 10 -parameter M6-large is the largest pretrained model in Chinese
- Experimental results show that our proposed M6 outperforms the baseline in a number of downstream tasks concerning both single modality and multiple modalities We will continue the pretraining of extremely large models by increasing data to explore the limit of its performance
Upload PDF to Generate Summary
Must-Reading Tree
Example
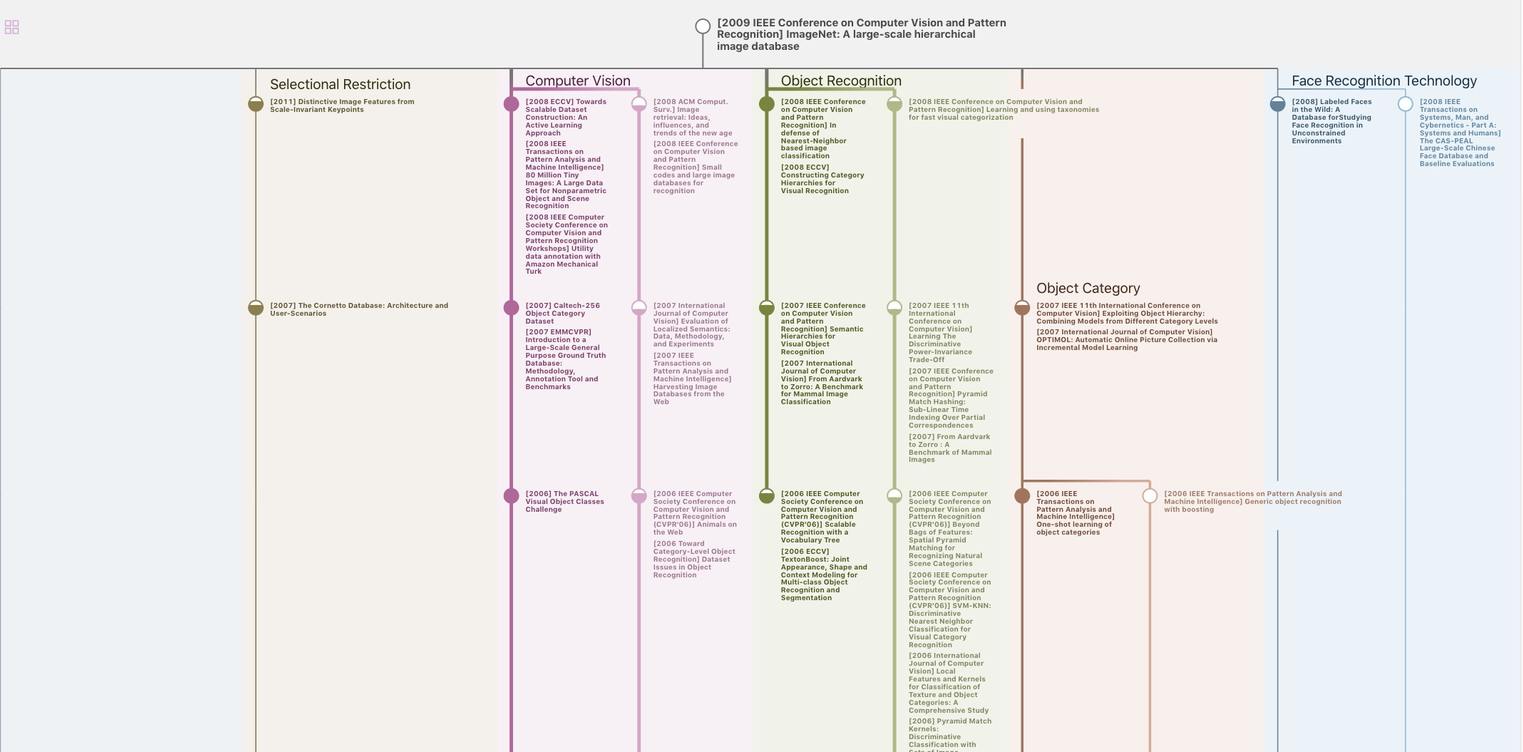
Generate MRT to find the research sequence of this paper
Related Papers
CPT-PHARMACOMETRICS & SYSTEMS PHARMACOLOGY 2023
被引用3
INTERNATIONAL JOURNAL OF SURGERY 2023
被引用0
Multiorgan Locked-State Model of Chronic Diseases and Systems Pharmacology Opportunities.
Drug Discovery Today 2024
被引用1
Data Disclaimer
The page data are from open Internet sources, cooperative publishers and automatic analysis results through AI technology. We do not make any commitments and guarantees for the validity, accuracy, correctness, reliability, completeness and timeliness of the page data. If you have any questions, please contact us by email: report@aminer.cn
Chat Paper