Prediction of Compressive Strength of Corncob Ash Concrete for Environmental Sustainability Using an Artificial Neural Network: A Soft Computing Techniques
Journal of Soft Computing in Civil Engineering(2023)
摘要
Agricultural waste materials are increasingly being used as partial replacements for cement in concrete. Several experimental studies are available to evaluate the mechanical properties of plastic waste reinforced concrete but there are limited evaluations on agricultural waste material. In this study, an attempt is made to investigate the compressive strength of Corn Cob Ash (CCA) concrete at different replacement levels by implementing an Artificial Neural Network (ANN). As the percentage of CCA increases, workability, density and compressive strength decreases, hence the developed ANN model consists of 3 input parameters (cement content, CCA content, and curing ages) in the input layer, 4 hidden neurons in the hidden layer and 3 output parameters (slump, density, and compressive strength) in the output layer. Training is done by adopting Levenberg-Marquardt back-propagation algorithm by considering 80% of experimental data with log-sigmoid activation function for both hidden and output layers. The developed model has a high correlation coefficient of 0.999 for both the training and testing data sets. It has low MSE and MAPE values of 2.2768x10-5 and 1.25 for training data respectively and 3.0463x10-5 and 1.37 for testing data respectively. Hence, it is concluded that the developed model predicts the output at an average rate of 98% accuracy. The predicted 2.5% replaced CCA concrete shows the best performance at all curing ages. Therefore, this percentage level is considered as an optimum replacement level which does not much affect the hardened properties of concrete.
更多查看译文
关键词
soft-computing techniques,levenberg-marquardt,agriculture waste,construction materials,pozzolanic material
AI 理解论文
溯源树
样例
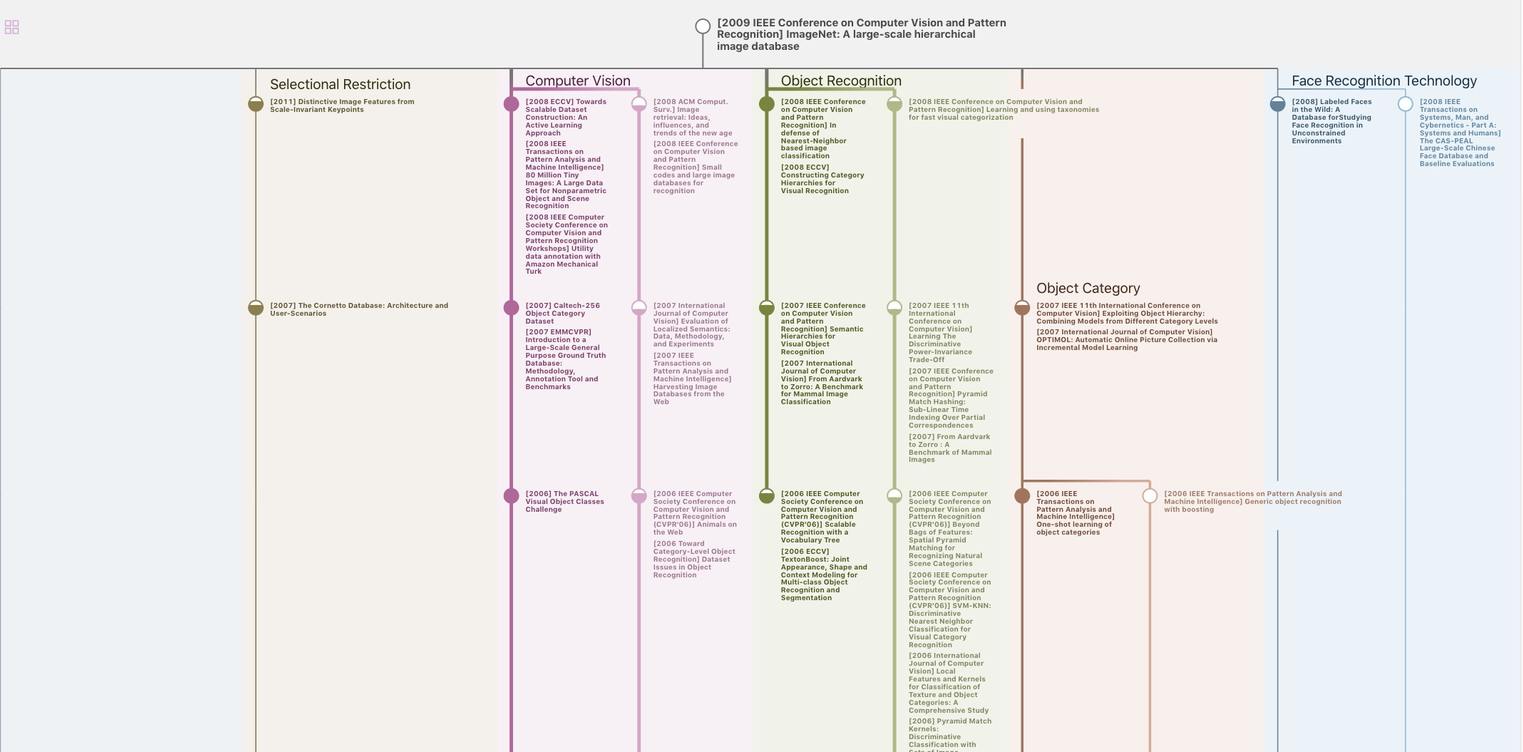
生成溯源树,研究论文发展脉络
Chat Paper
正在生成论文摘要