RUemo—The Classification Framework for Russia-Ukraine War-Related Societal Emotions on Twitter Through Machine Learning
ALGORITHMS(2023)
Key words
Russia Ukraine war,emoroberta,BERT,emotion detection,Twitter,transfer learning,machine learning,social media,society,transformers
AI Read Science
Must-Reading Tree
Example
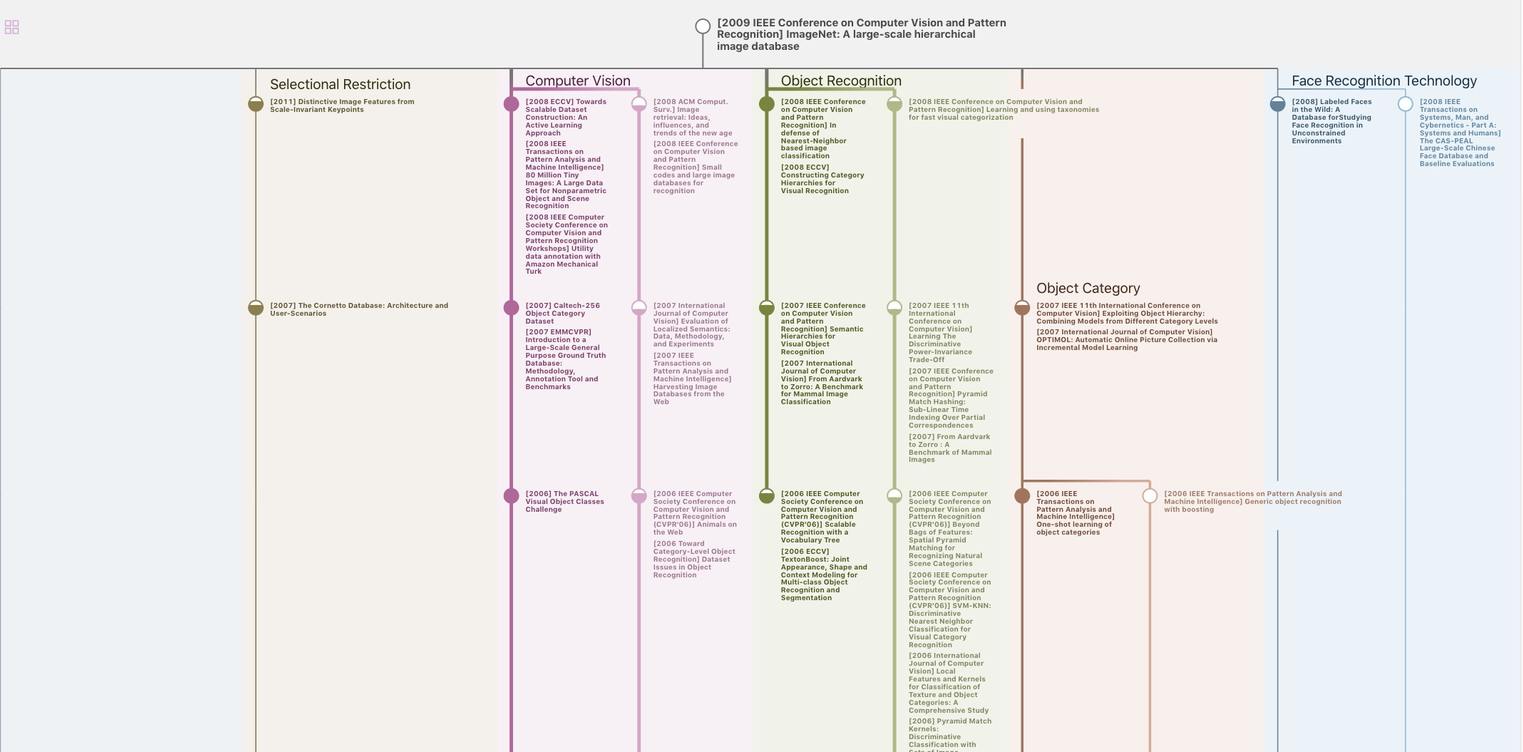
Generate MRT to find the research sequence of this paper
Chat Paper
Summary is being generated by the instructions you defined