LBM-MHD Data-Driven Approach to Predict Rayleigh-Bénard Convective Heat Transfer by Levenberg-Marquardt Algorithm.
Axioms(2023)
摘要
This study aims to consider lattice Boltzmann method (LBM)-magnetohydrodynamics (MHD) data to develop equations to predict the average rate of heat transfer quantitatively. The present approach considers a 2D rectangular cavity with adiabatic side walls, and the bottom wall is heated while the top wall is kept cold. Rayleigh-Benard (RB) convection was considered a heat-transfer phenomenon within the cavity. The Hartmann (Ha) number, by varying the inclination angle (theta), was considered in developing the equations by considering the input parameters, namely, the Rayleigh (Ra) numbers, Darcy (Da) numbers, and porosity (epsilon) of the cavity in different segments. Each segment considers a data-driven approach to calibrate the Levenberg-Marquardt (LM) algorithm, which is highly linked with the artificial neural network (ANN) machine learning method. Separate validations have been conducted in corresponding sections to showcase the accuracy of the equations. Overall, coefficients of determination (R-2) were found to be within 0.85 to 0.99. The significant findings of this study present mathematical equations to predict the average Nusselt number (Nu over bar ). The equations can be used to quantitatively predict the heat transfer without directly simulating LBM. In other words, the equations can be considered validations methods for any LBM-MHD model, which considers RB convection within the range of the parameters in each equation.
更多查看译文
关键词
lattice Boltzmann,Rayleigh-Benard convection,magnetohydrodynamics,Levenberg-Marquardt algorithm,data-driven analysis,Nusselt number,Hartmann number,porosity,rectangular cavity
AI 理解论文
溯源树
样例
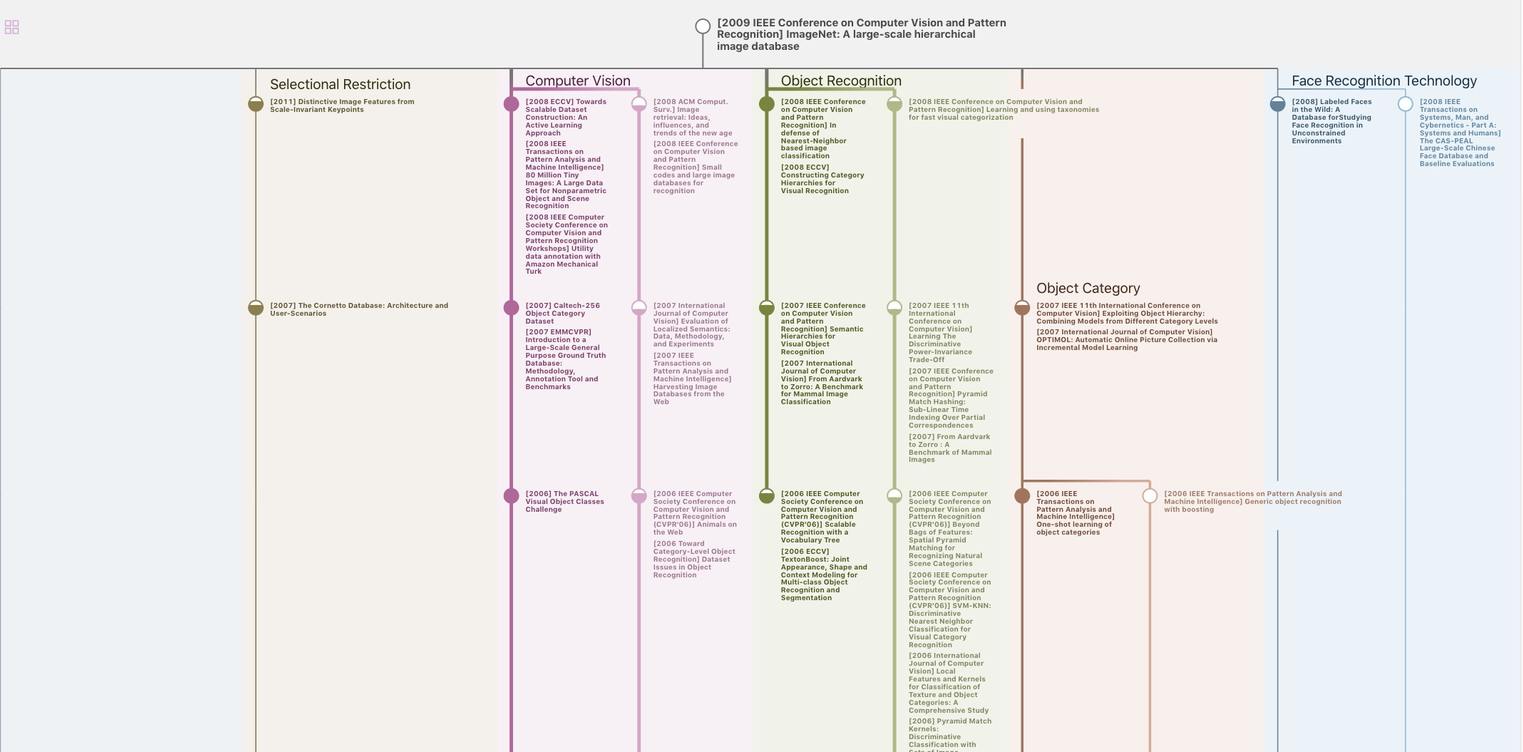
生成溯源树,研究论文发展脉络
Chat Paper
正在生成论文摘要