On the Estimation of the Binary Response Model.
Axioms(2023)
摘要
The binary logistic regression model (LRM) is practical in situations when the response variable (RV) is dichotomous. The maximum likelihood estimator (MLE) is generally considered to estimate the LRM parameters. However, in the presence of multicollinearity (MC), the MLE is not the correct choice due to its inflated standard deviation (SD) and standard errors (SE) of the estimates. To combat MC, commonly used biased estimators, i.e., the Ridge estimators (RE) and Liu estimators (LEs), are preferred. However, most of the time, the traditional LE attains a negative value for its Liu parameter (LP), which is considered to be a major drawback. Therefore, to overcome this issue, we proposed a new adjusted LE for the binary LRM. Owing to numerical evaluation purposes, Monte Carlo simulation (MCS) study is performed under different conditions where bias and mean squared error are the performance criteria. Findings showed the superiority of our proposed estimator in comparison with the other estimation methods due to the existence of high but imperfect multicollinearity, which clearly means that it is consistent when the regressors are multicollinear. Furthermore, the findings demonstrated that whenever there is MC, the MLE is not the best choice. Finally, a real application is being considered to be evidence for the advantage of the intended estimator. The MCS and the application findings pointed out that the considered adjusted LE for the binary logistic regression model is a more efficient estimation method whenever the regressors are highly multicollinear.
更多查看译文
关键词
bias,binary logistic regression model,Liu estimator,multicollinearity,maximum likelihood estimator,ridge regression
AI 理解论文
溯源树
样例
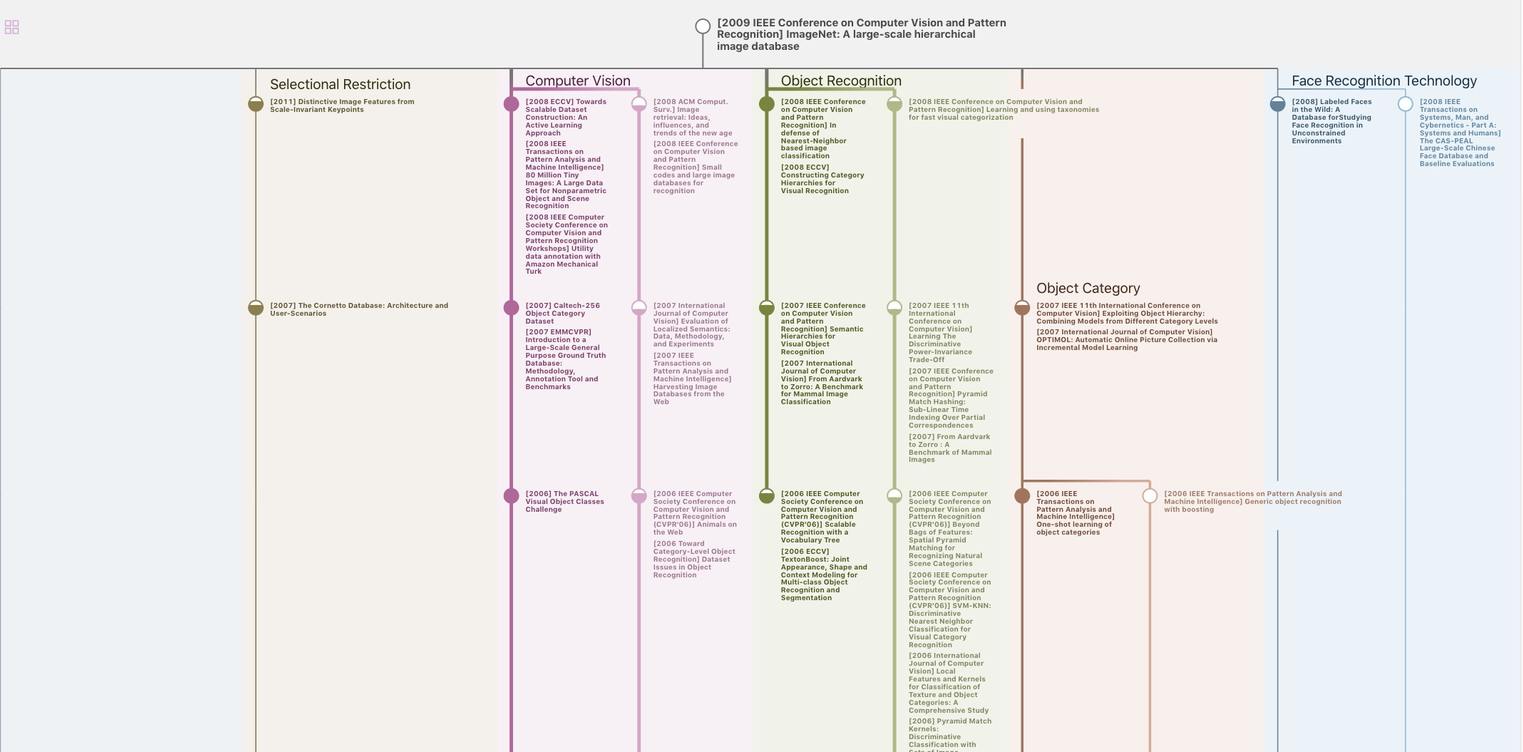
生成溯源树,研究论文发展脉络
Chat Paper
正在生成论文摘要