Discovering Wiring Patterns Influencing Neural Network Performance
ECML/PKDD (3)(2023)
摘要
The search for optimal neural network architecture is a well-known problem in deep learning. However, as many algorithms have been proposed in this domain, little attention is given to the analysis of wiring properties that are beneficial or detrimental to the network performance. We take a step at addressing this issue by performing a massive evaluation of artificial neural networks with various computational architectures, where the diversity of the studied constructions is obtained by basing the wiring topology of the networks on different types of random graphs. Our goal is to investigate the structural and numerical properties of the graphs and assess their relation to the test accuracy of the corresponding neural networks. We find that none of the classical numerical graph invariants by itself allows to single out the best networks. Consequently, we introduce a new numerical graph characteristic, called quasi-1-dimensionality, which is able to identify the majority of the best-performing graphs.
更多查看译文
关键词
Deep learning,Artificial neural networks,Neural architectures,Network analysis,Image classification
AI 理解论文
溯源树
样例
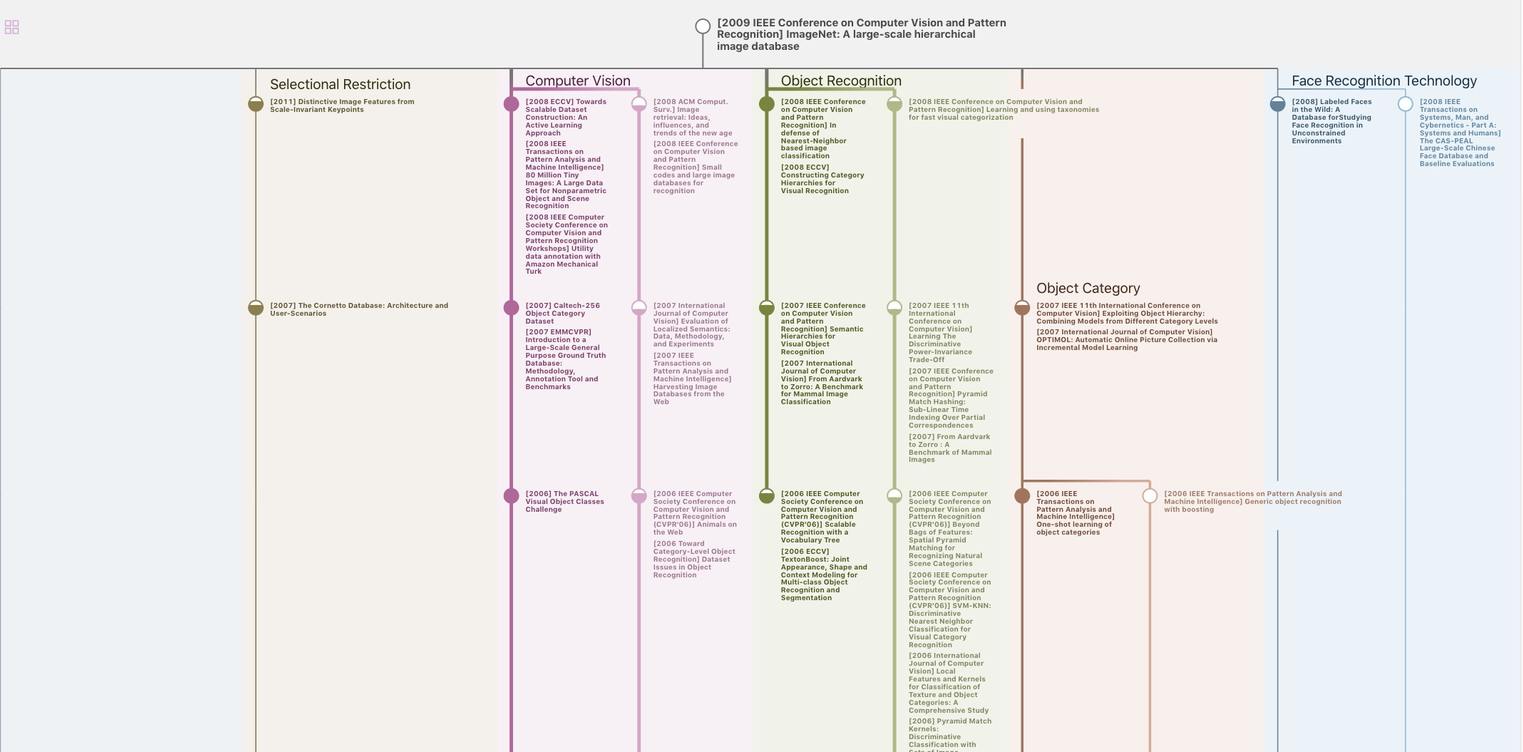
生成溯源树,研究论文发展脉络
Chat Paper
正在生成论文摘要