Statistics-Guided Accelerated Swarm Feature Selection in Data-Driven Soft Sensors for Hybrid Engine Performance Prediction
IEEE transactions on industrial informatics(2023)
摘要
The accurate prediction of soft sensors is essential for the development of modern combustion engines to achieve better performance, lower emissions, and reduced fuel consumption. To precisely predict engine performance, i.e., indicated thermal efficiency, volumetric efficiency, and fuel consumption rate of a hybrid engine, in this article, we propose a novel data-driven approach of statistics-guided accelerated swarm feature selection to find the most effective features for engine soft sensors. Differing from the existing filter or wrapper feature selection approaches, this approach uses external measure information to direct velocity updates in the accelerated swarm feature selection. Several filter and wrapper methods are developed and comprehensively compared. The experimental dataset is collected from a BYD 1.5 L gasoline engine. Validated by bench test, the results demonstrate that the proposed approach finds the most effective features and optimal network structure for data-driven performance prediction of the hybrid engine that was studied.
更多查看译文
关键词
Engines,Feature extraction,Soft sensors,Correlation,Artificial neural networks,Finite impulse response filters,Principal component analysis,Accelerated particle swarm optimization (PSO),deep neural network,engine soft sensors,feature selection
AI 理解论文
溯源树
样例
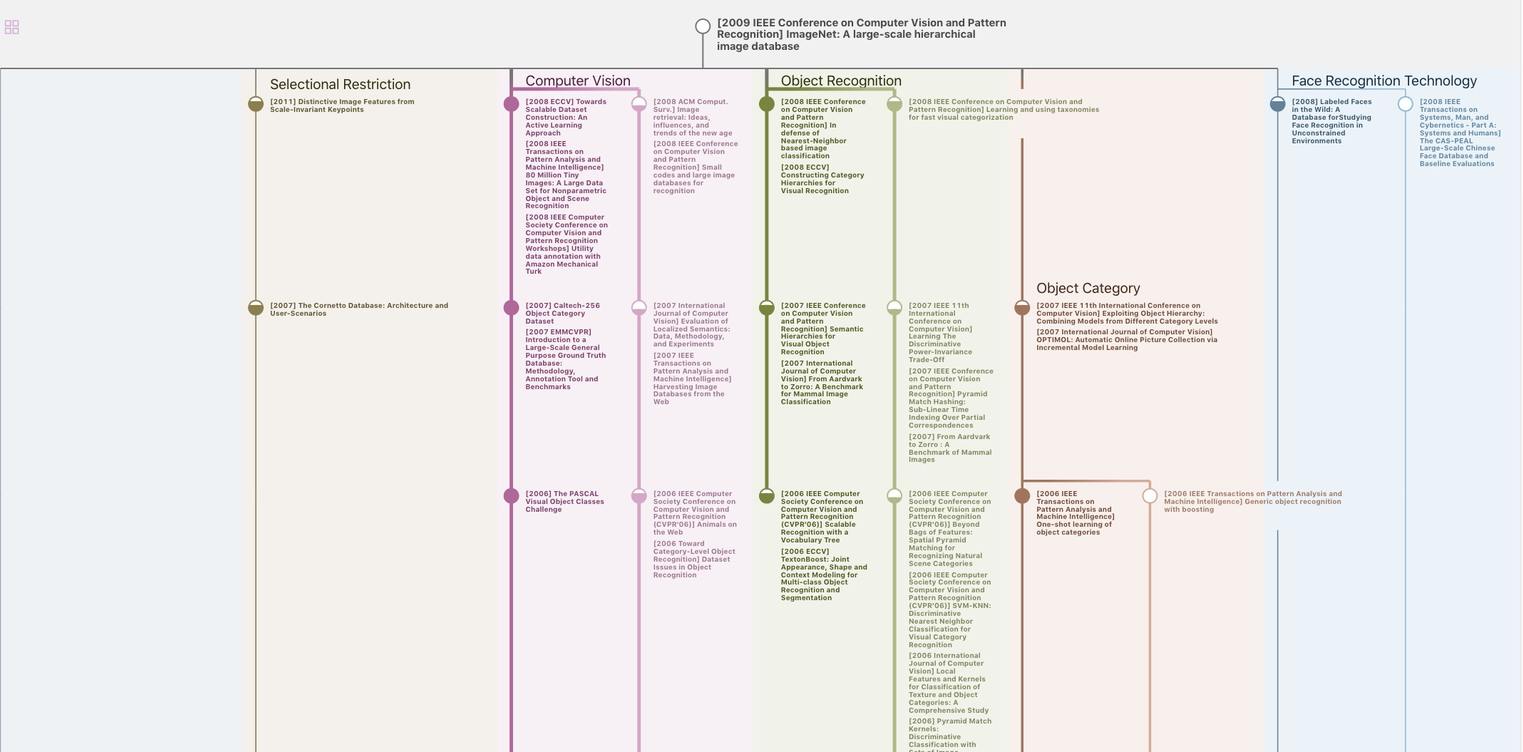
生成溯源树,研究论文发展脉络
Chat Paper
正在生成论文摘要