Group Guided Data Association for Multiple Object Tracking.
ACCV (7)(2022)
摘要
Multiple Object Tracking (MOT) usually adopts the Tracking-by-Detection paradigm, which transforms the problem into data association. However, these methods are restricted by detector performance, especially in dense scenes. In this paper, we propose a novel group-guided data association, which improves the robustness of MOT to error detections and increases tracking accuracy in occlusion areas. The tracklets are firstly clustered into groups of related motion patterns by a graph neural network. Using the idea of grouping, the data association is divided into two stages: intra-group and inter-group. For the intra-group, based on the structural relationship between objects, detections are recovered and associated by min-cost network flow. For inter-group, the tracklets are associated with the proposed hypotheses to solve long-term occlusion and reduce false positives. The experiments on the MOTChallenge benchmark prove our method’s effects, which achieves competitive results over state-of-the-art methods.
更多查看译文
关键词
group guided data association,tracking
AI 理解论文
溯源树
样例
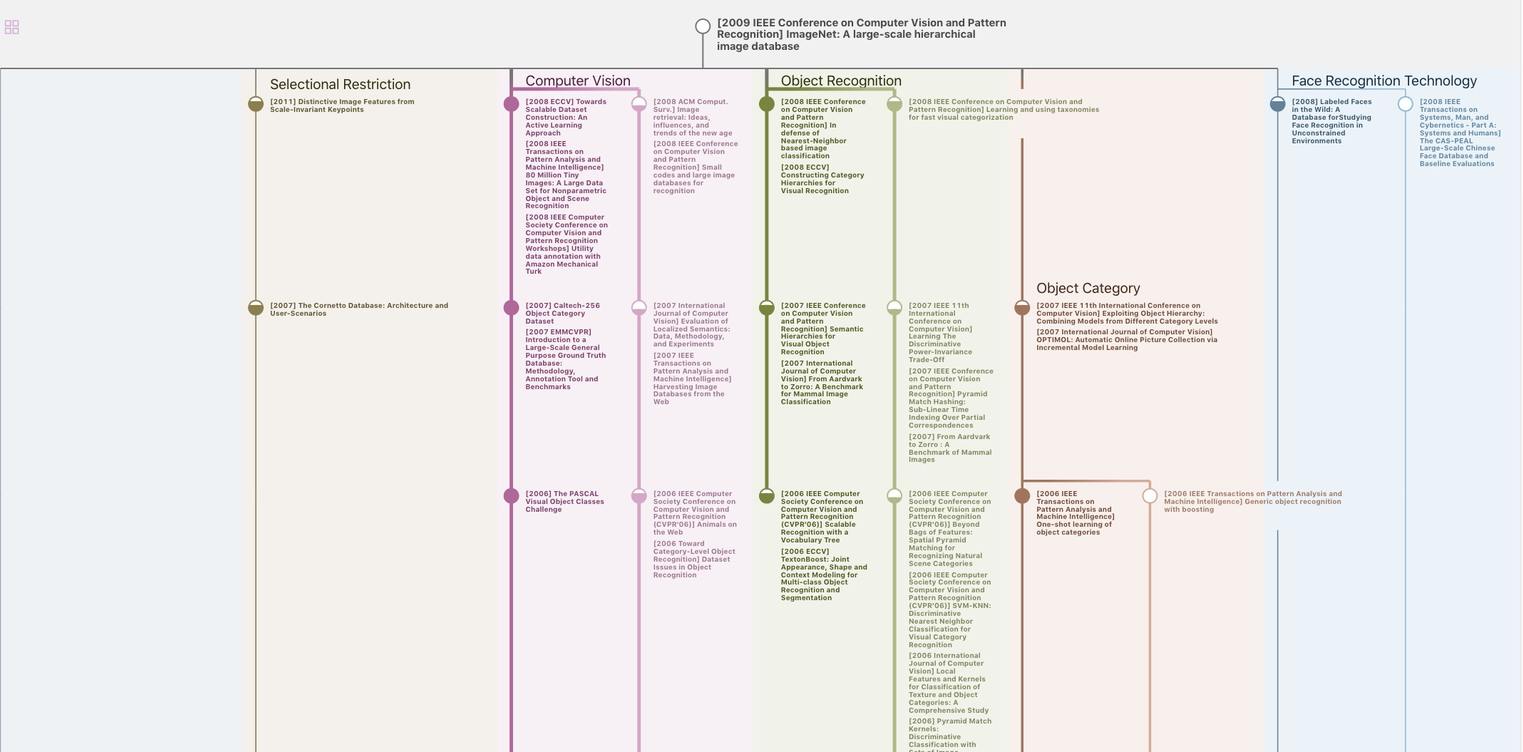
生成溯源树,研究论文发展脉络
Chat Paper
正在生成论文摘要