Computation Offloading Strategy Based on Multi-armed Bandit Learning in Microservice-enabled Vehicular Edge Computing Networks
2023 INTERNATIONAL CONFERENCE ON INFORMATION NETWORKING, ICOIN(2023)
摘要
One popular technology to improve the processing and storage capacities of vehicular networks (VNs) through the offloading of computing tasks is vehicular edge computing (VEC). Moreover, to provide better services for users in proximity, microservices can be dynamically deployed, easily migrated among edge clouds on demand, and launched rapidly in a VEC environment. However, the environment of VNs is rapidly changing and unpredictable, making it difficult to provide service with low latency. Therefore, in order to deliver real-time services in microservice-enabled VNs, a multi-armed bandit (MAB) learning-based computation offloading (MLCO) strategy is introduced in this study. The proposed scheme enables that vehicles can learn the offloading delay performance of the candidates while offloading computing tasks. Furthermore, we modified the MAB algorithms and added an input-awareness strategy to our proposed algorithm for adapting to a rapidly changing task offloading vehicular environment. Extensive simulation results show that our proposal outperforms other existing baselines in terms of average service latency and successfully offloads more tasks in different scenarios.
更多查看译文
关键词
vehicular edge computing,microservice,MAB theory,task offloading,vehicular networks
AI 理解论文
溯源树
样例
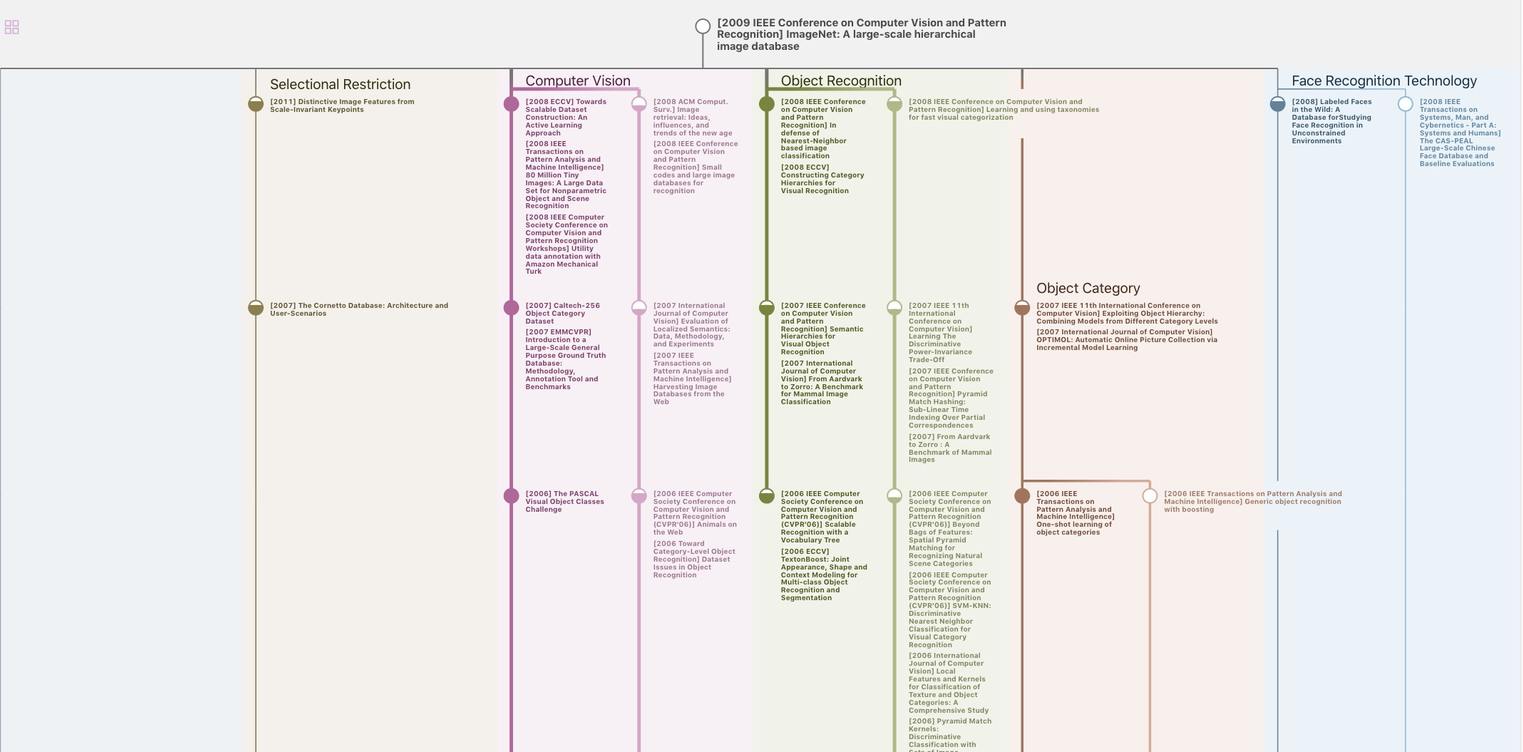
生成溯源树,研究论文发展脉络
Chat Paper
正在生成论文摘要