MedSeq: Semantic Segmentation for Medical Image Sequences
2022 IEEE International Conference on Bioinformatics and Biomedicine (BIBM)(2022)
摘要
Medical image segmentation plays a critical role in computer-aided diagnosis, while the diversity and complexity of medical images make it difficult to segment precisely. In practice, medical images of specific modalities (e.g. Magnetic Resonance Imaging, Colonoscopy and Ultrasonography) are collected as sequences independently for every patient. However, 1) there exists few works exploiting sequence information among successive frames, neglecting inter-frame relationships that are useful to locate target objects; 2) the performance of medical image segmentation is limited to the low contrast or blurry boundary of medical images, and intra-frame dependencies are not fully explored. Thus in this paper, we propose MedSeq for segmenting objects of interest in medical image sequences. Following the “locate-then-refine” paradigm, we locate target regions by modeling cross-frame relationships and then perform refinement on coarse masks. More specifically, we design a Cross-frame Attention module to learn correlations among frames, taking advantages of their similar appearances. For refinement, we propose a novel Boundary-aware Transformer to improve the segmentation of boundary patches. Extensive experiments are conducted on benchmark datasets of Cardiac Segmentation and Video Polyp Segmentation. Our method achieves superior performance over the state-of-the-art methods.
更多查看译文
关键词
Medical Image Sequence,Semantic Segmentation,Cross-frame Attention,Boundary-aware Transformer
AI 理解论文
溯源树
样例
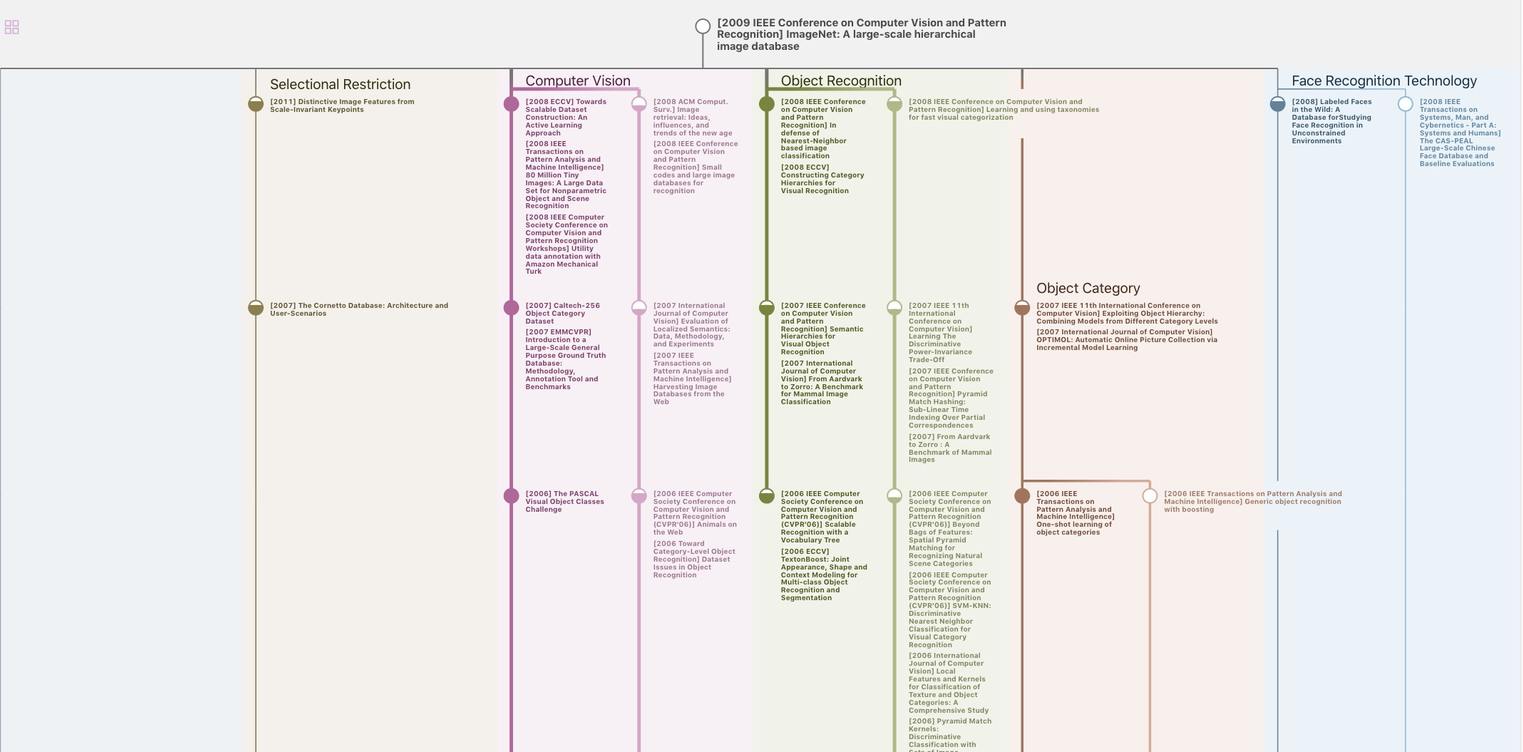
生成溯源树,研究论文发展脉络
Chat Paper
正在生成论文摘要