A Novel Graph Kernel Based on the Wasserstein Distance and Spectral Signatures
S+SSPR(2022)
摘要
Spectral signatures have been used with great success in computer vision to characterise the local and global topology of 3D meshes. In this paper, we propose to use two widely used spectral signatures, the Heat Kernel Signature and the Wave Kernel Signature, to create node embeddings able to capture local and global structural information for a given graph. For each node, we concatenate its structural embedding with the one-hot encoding vector of the node feature (if available) and we define a kernel between two input graphs in terms of the Wasserstein distance between the respective node embeddings. Experiments on standard graph classification benchmarks show that our kernel performs favourably when compared to widely used alternative kernels as well as graph neural networks.
更多查看译文
关键词
Graph kernel,Wasserstein distance,Spectral signature
AI 理解论文
溯源树
样例
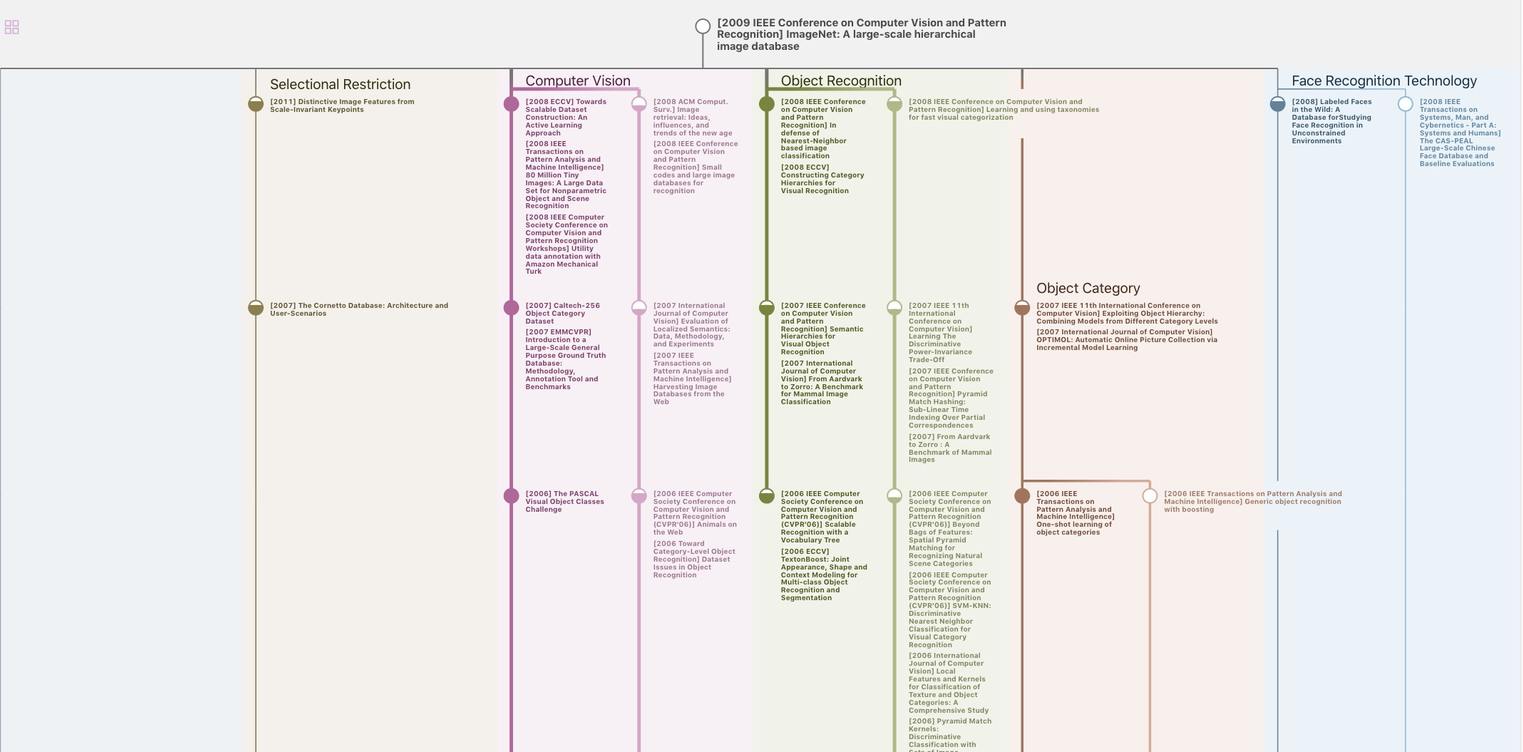
生成溯源树,研究论文发展脉络
Chat Paper
正在生成论文摘要