Distilling DETR-Like Detectors with Instance-Aware Feature.
ICIP(2022)
摘要
DEtection TRansformer (DETR) has achieved great success in object detection but suffers from slow convergence during training process. Knowledge distillation (KD) can speed up model training but has the problem of locating knowledgeable regions in object detection. Related methods mainly locate knowledgeable regions empirically. To address those challenges, we propose a novel distillation framework for DETR-like transformer-based detectors. The key idea is to connect each instance with its corresponding response region in the feature map through cross attention. To better fuse the attention maps between different queries and heads, we introduce an attention fusion module to balance instances of different scales. Extensive experiments on DETR and Conditional DETR are conducted to verify our proposed method. Our method improves the mAP by 3.19% for Conditional DETR with ResNet-50 backbone trained for 50 epochs, which outperforms the strong teacher trained for 108 epochs. We also boost DETR with ResNet-50 backbone from 33.97% to 42.13% mAP (+8.16%) under 50 epochs.
更多查看译文
关键词
Object Detection, Knowledge Distillation, Attention Mechanism
AI 理解论文
溯源树
样例
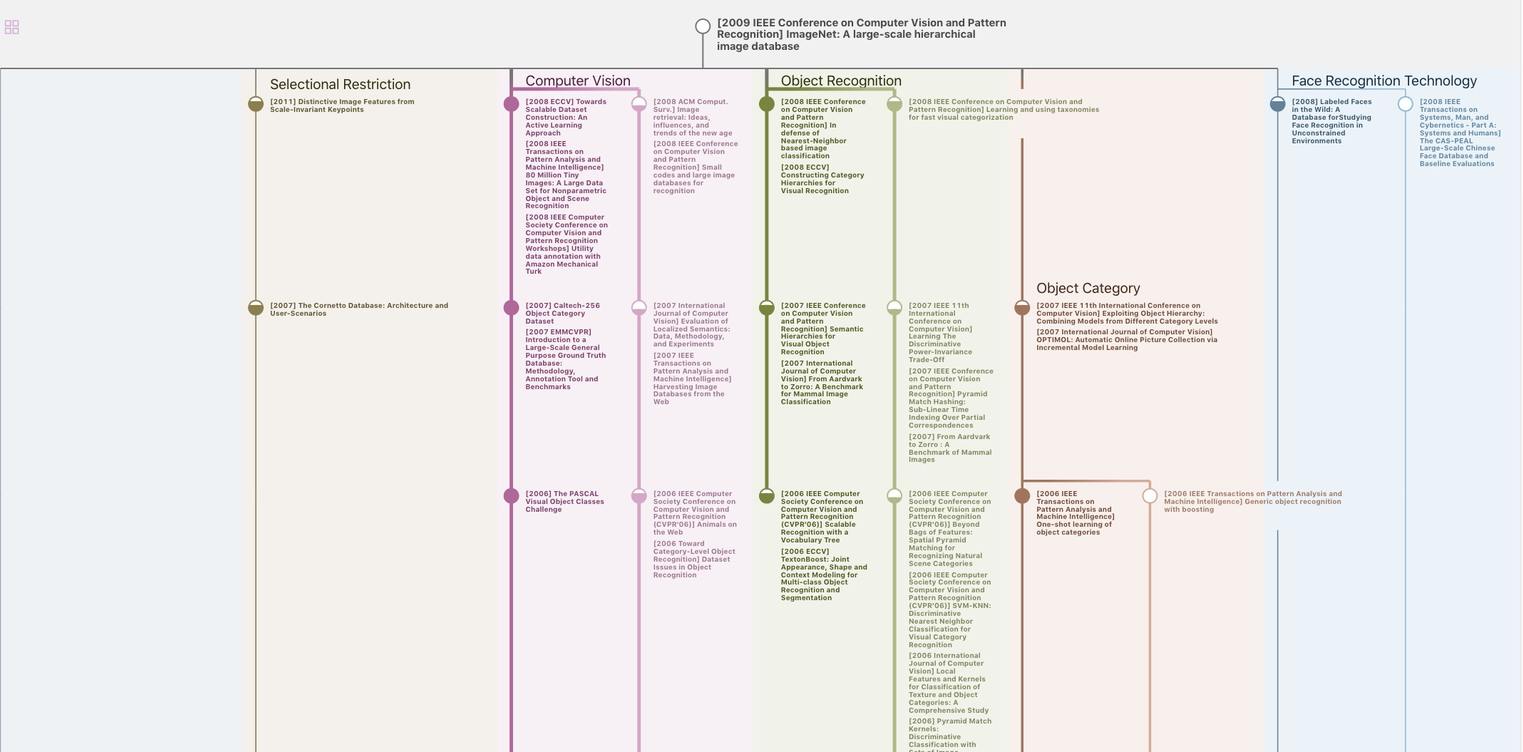
生成溯源树,研究论文发展脉络
Chat Paper
正在生成论文摘要