Fast and Light Object Classification from Aerial Drone Image.
SII(2023)
摘要
This paper proposes a fast and solid method to classify objects from the aerial drone image with different altitudes and perspective angles. To classify objects from drone datasets with various characteristics, there are two difficulties. The first difficulty is that the object size is too small. The second difficulty is that the object has many various characteristics. Therefore, we proposed a simple convolution blocks and shortcut blocks with a dilate convolution. Since the drone image is a 4k (3840x2160) in size, thus a very high resolution, neighboring pixels for a pixel of interest are similar to each other, so it is advantageous in terms of speed to use the skipped pixels. However, as the network goes deeper, the feature map size becomes smaller and there is a possibility that the surrounding pixel values become much different. Therefore, the normal convolution layer was also arranged and constructed. In addition, since the objects in the drone image have a small size because taken at a high altitude, it loses more features as the network gets deeper. Therefore, the features extracted from the original image were supplemented by periodically calculating each pixel by using the shortcut technique. It records fewer parameters than other networks, thus it executes faster and more accurately. The number of parameters is 1,032,054, which is lower than MobileNet(3,504,872), ShuffleNet(1,366,792), and SqueezeNet(1,248,424). Also, the proposed network outperformed with an accuracy of 98.18%, which is higher than MobileNet(97.28%), ShuffleNet(95.88%), and SqueezeNet(94.32%). when classifying images with 4 GTX3090s, it took in a speed of 1,102 FPS. Therefore, it was proved that fast and accurate network configuration is possible when classification experiments of Aerial Drone Images with various features are conducted using a simple convolution block and shortcut block.
更多查看译文
关键词
aerial drone image,Aerial Drone Images,classifying images,drone datasets,fast method,feature map size,normal convolution layer,object size,perspective angles,shortcut block,simple convolution block,simple convolution blocks,skipped pixels,solid method,surrounding pixel values
AI 理解论文
溯源树
样例
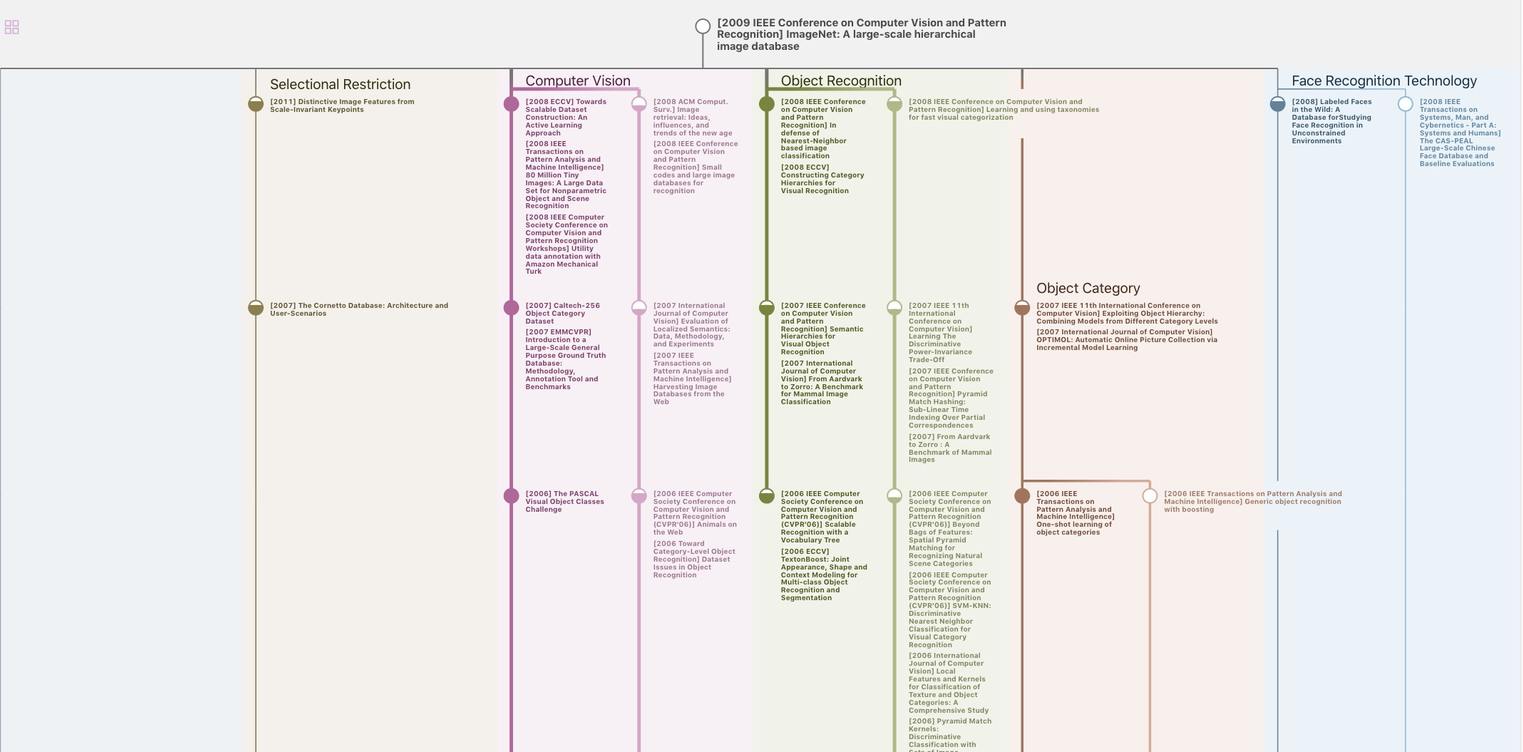
生成溯源树,研究论文发展脉络
Chat Paper
正在生成论文摘要