PPDU: Dynamic Graph Publication with Local Differential Privacy
Knowledge and information systems(2023)
摘要
Local differential privacy (LDP) is an emerging privacy-preserving data collection model that requires no trusted third party. Most privacy-preserving decentralized graph publishing studies adopt LDP technique to ensure individual privacy. However, existing LDP-based synthetic graph generation approaches focus on static graph publishing and can only republish synthetic graphs in a brute-force manner when dealing with dynamic graph problems, resulting in low synthetic graph accuracy. The main difficulties come from the two steps of dynamic graph publishing: excessive noise injection in initial graph generation and over-segmentation of the privacy budget in graph update . We address these two issues by presenting PPDU, the first dynamic graph publication approach under LDP. PPDU uses a privacy-preference-specifying mechanism to untie the noise injection and the graph size, significantly reducing noise injection. We then divide the privacy-preserving graph update problem into three subproblems: node insertion, edge insertion, and edge deletion, and propose update threshold-based dynamic graph releasing methods to avoid excessive segmentation of the privacy budget, thereby significantly improving the accuracy of synthetic graphs. Theoretical analysis and experimental results prove that our solution can continually yield high-quality dynamic graphs while satisfying edge LDP.
更多查看译文
关键词
Decentralized social networks,Dynamic graph publication,Local differential privacy,Security and privacy
AI 理解论文
溯源树
样例
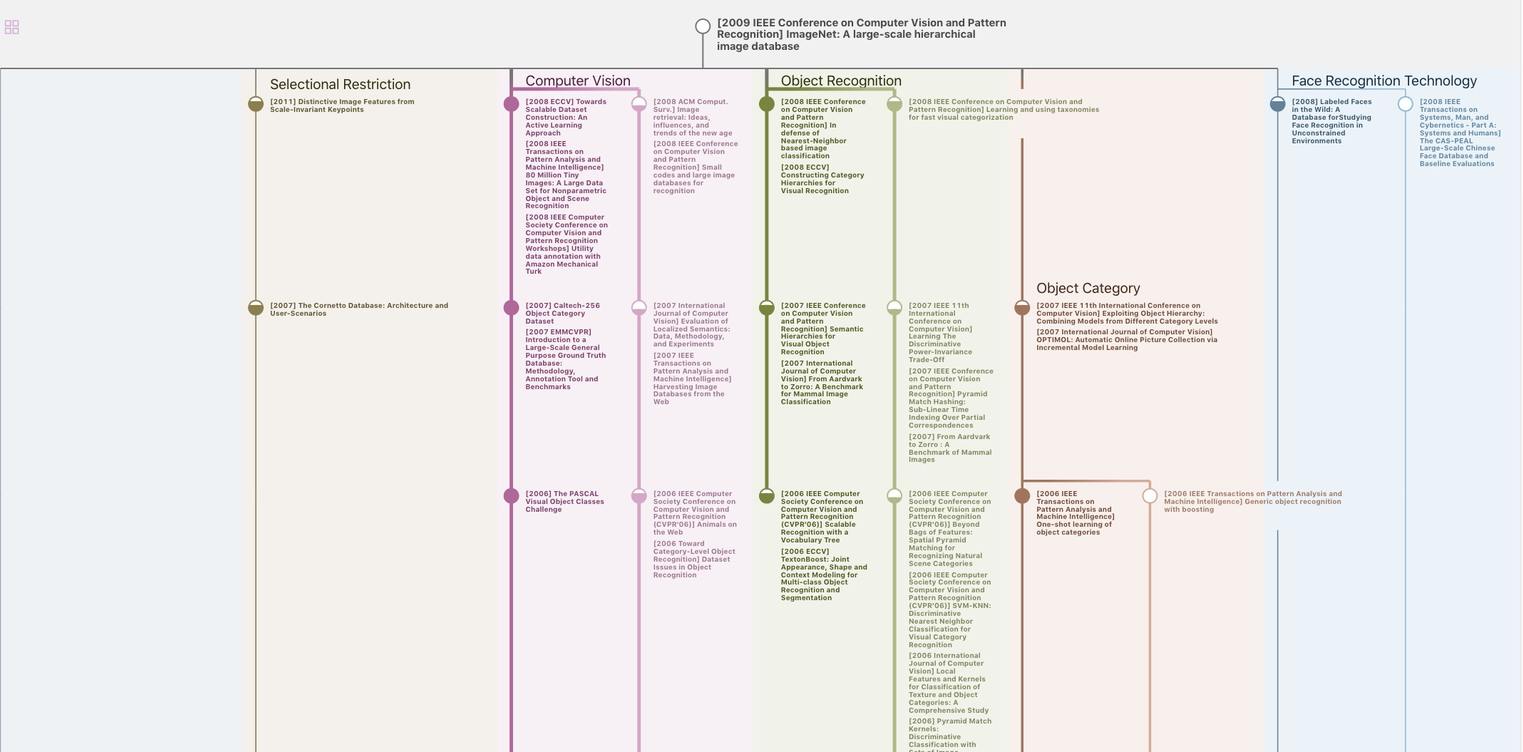
生成溯源树,研究论文发展脉络
Chat Paper
正在生成论文摘要