NEWTON: Neural View-Centric Mapping for On-the-Fly Large-Scale SLAM
IEEE ROBOTICS AND AUTOMATION LETTERS(2024)
摘要
Neural field-based 3D representations have recently been adopted in many areas including SLAM systems. Current neural SLAM or online mapping systems lead to impressive results in the presence of simple captures, but they rely on a world-centric map representation as only a single neural field model is used. To define such a world-centric representation, accurate and static prior information about the scene, such as its boundaries and initial camera poses, are required. However, in real-time and on-the-fly scene capture applications, this prior knowledge cannot be assumed as fixed or static, since it dynamically changes and it is subject to significant updates based on run-time observations. Particularly in the context of large-scale mapping, significant camera pose drift is inevitable, necessitating the correction via loop closure. To overcome this limitation, we propose NEWTON , a view-centric mapping method that dynamically constructs neural fields based on run-time observation. In contrast to prior works, our method enables camera pose updates using loop closures and scene boundary updates by representing the scene with multiple neural fields, where each is defined in a local coordinate system of a selected keyframe. The experimental results demonstrate the superior performance of our method over existing world-centric neural field-based SLAM systems, in particular for large-scale scenes subject to camera pose updates.
更多查看译文
关键词
Cameras,Simultaneous localization and mapping,Training,Three-dimensional displays,Streams,Optimization,Trajectory,Vision-based navigation,SLAM,mapping
AI 理解论文
溯源树
样例
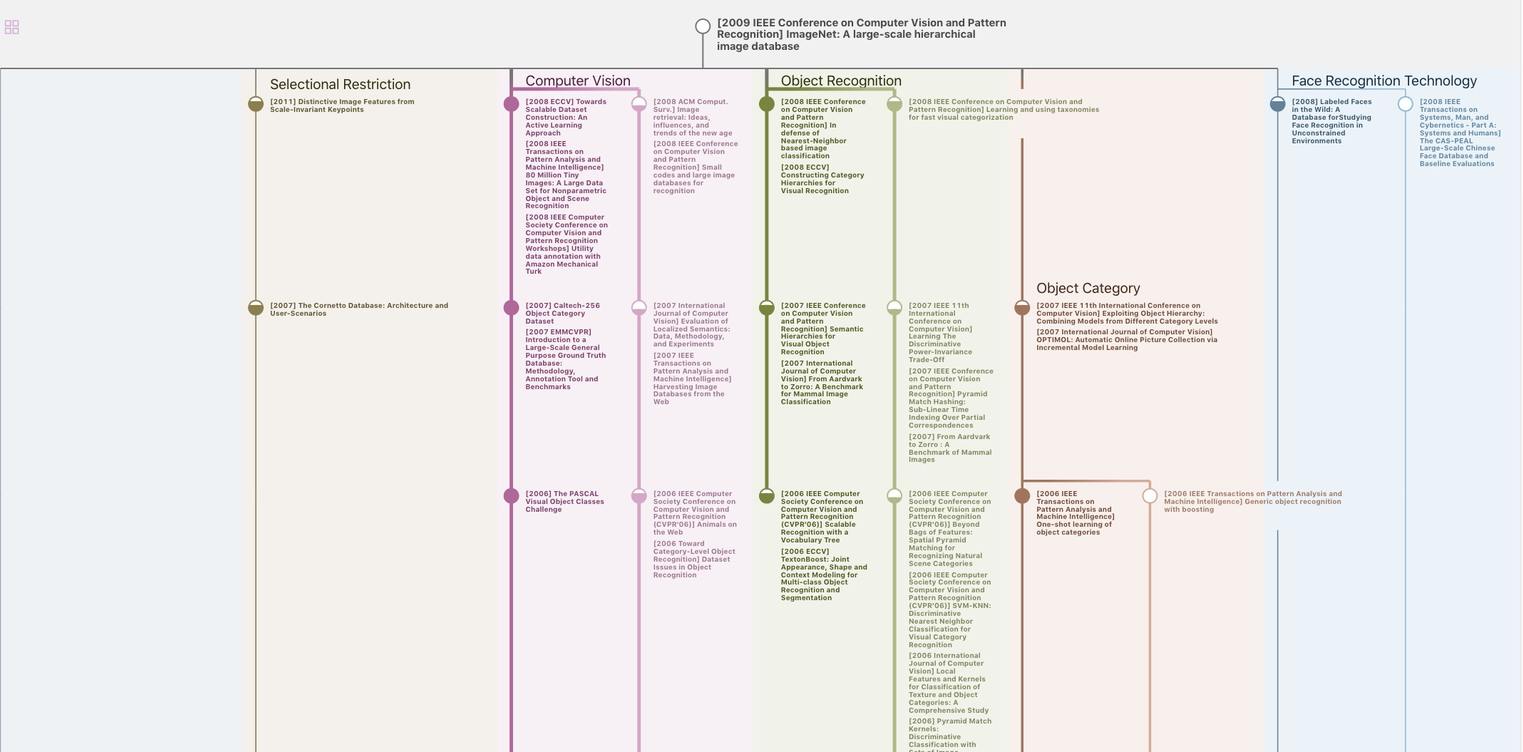
生成溯源树,研究论文发展脉络
Chat Paper
正在生成论文摘要