CT-defined Body Composition As a Prognostic Factor in Multiple Myeloma
Hematology (Amsterdam, Netherlands)(2023)SCI 4区
Abstract
Objectives Body composition assessment is comprised by skeletal muscle mass (SMM) and subcutaneous and visceral adipose tissue (SAT and VAT) and can be quantified by imaging. It can be predictive of several clinically outcomes in patients with hematological diseases. Our aim was to establish the effect of body composition parameters on overall survival (OS) and progression-free survival (PFS) in patients with multiple myeloma (MM). Materials and methods All patients with MM were retrospectively analyzed between 2009 and 2019. One hundred twenty-three patients were included into the analysis. Whole-body computed tomography (CT) was used to calculate SMM, VAT, and SAT. Results Overall, 22 patients (17.9%) of the patient sample died. Forty patients were sarcopenic (32.5%), 79 patients were visceral obese (64.2%), and 18 patients (14.6%) were sarcopenic obese. Parameter of body composition did not influence OS: sarcopenia, hazard ratio (HR) = 1.3 (95% CI 0.50-3.34), p = .59; visceral obesity, HR = 1.6 (95% CI 0.70-3.76), p = .26; sarcopenic obesity, HR = 2.3 (95% CI 0.90-5.63), p = 0.08. Patients with infectious complications showed higher VAT values. Conclusions CT-defined body composition parameters have no influence on survival in patients with MM undergoing autologous stem-cell therapy. These results corroborate previous smaller studies that body composition might have a limited role in this tumor entity. VAT may predict the occurrence of infectious complications.
MoreTranslated text
Key words
CT,body composition,multiple myeloma
求助PDF
上传PDF
View via Publisher
AI Read Science
AI Summary
AI Summary is the key point extracted automatically understanding the full text of the paper, including the background, methods, results, conclusions, icons and other key content, so that you can get the outline of the paper at a glance.
Example
Background
Key content
Introduction
Methods
Results
Related work
Fund
Key content
- Pretraining has recently greatly promoted the development of natural language processing (NLP)
- We show that M6 outperforms the baselines in multimodal downstream tasks, and the large M6 with 10 parameters can reach a better performance
- We propose a method called M6 that is able to process information of multiple modalities and perform both single-modal and cross-modal understanding and generation
- The model is scaled to large model with 10 billion parameters with sophisticated deployment, and the 10 -parameter M6-large is the largest pretrained model in Chinese
- Experimental results show that our proposed M6 outperforms the baseline in a number of downstream tasks concerning both single modality and multiple modalities We will continue the pretraining of extremely large models by increasing data to explore the limit of its performance
Upload PDF to Generate Summary
Must-Reading Tree
Example
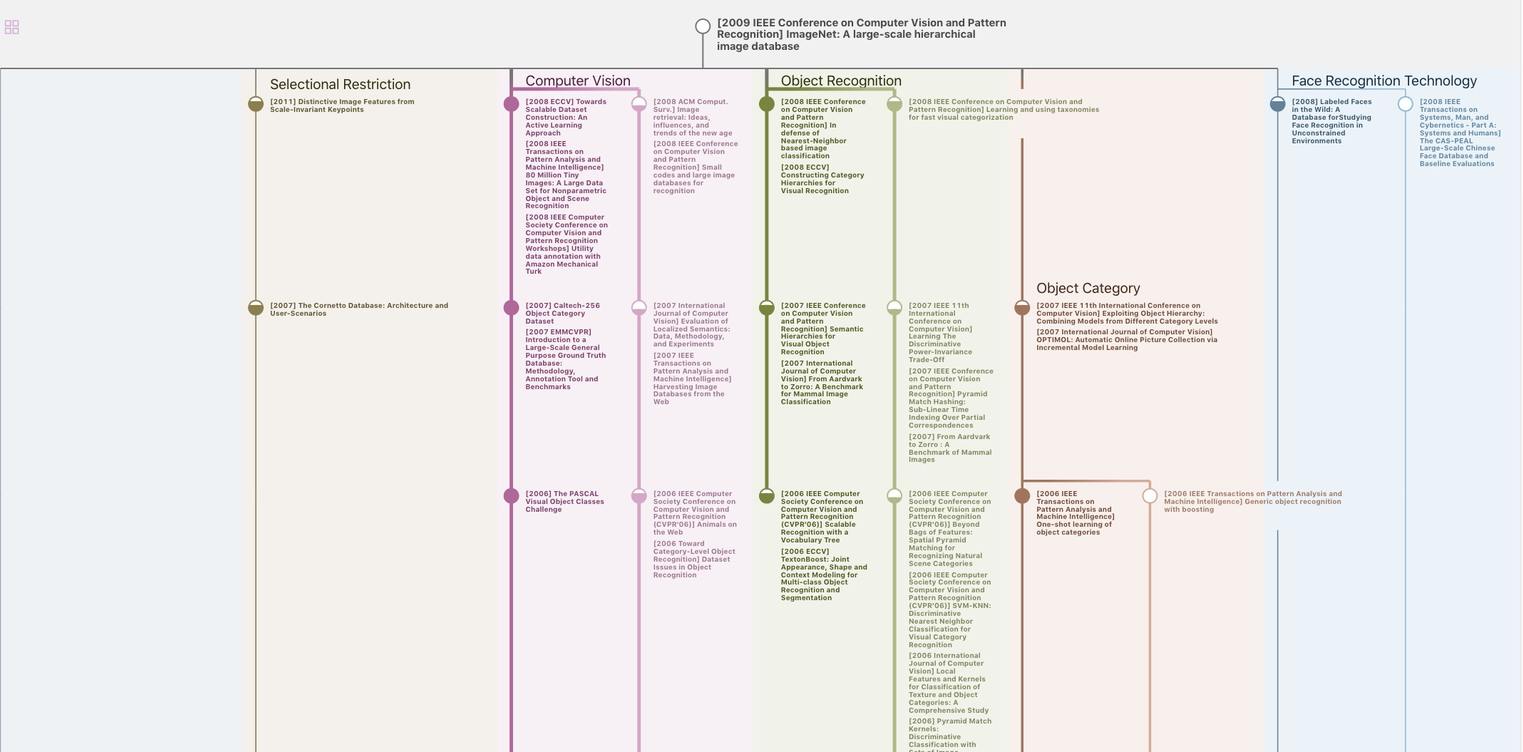
Generate MRT to find the research sequence of this paper
Related Papers
DIAGNOSTIC AND INTERVENTIONAL RADIOLOGY 2023
被引用0
Leukemia & lymphoma/Leukemia and lymphoma 2024
被引用1
Data Disclaimer
The page data are from open Internet sources, cooperative publishers and automatic analysis results through AI technology. We do not make any commitments and guarantees for the validity, accuracy, correctness, reliability, completeness and timeliness of the page data. If you have any questions, please contact us by email: report@aminer.cn
Chat Paper
GPU is busy, summary generation fails
Rerequest