A Multi-View Thermal-Visible Image Dataset for Cross-Spectral Matching.
Remote. Sens.(2023)
摘要
Cross-spectral local feature matching between visual and thermal images benefits many vision tasks in low-light environments, including image-to-image fusion and camera re-localization. An essential prerequisite for unleashing the potential of supervised deep learning algorithms in the area of visible-thermal matching is the availability of large-scale and high-quality annotated datasets. However, publicly available datasets are either in relative small quantity scales or have limited pose annotations due to the expensive cost of data acquisition and annotation, which severely hinders the development of this field. In this paper, we proposed a multi-view thermal-visible image dataset for large-scale cross-spectral matching. We first recovered a 3D reference model from a group of collected RGB images, in which a certain image (bridge) shares almost the same pose as the thermal query. We then effectively registered the thermal image to the model based on manually annotating a 2D-2D tie point between the bridge and the thermal. In this way, through simply annotating one same viewpoint image pair, numerous overlapping image pairs between thermal and visible could be available. We also proposed a semi-automatic approach for generating accurate supervision for training multi-view cross-spectral matching. Specifically, our dataset consists of 40,644 cross-modal pairs with well supervision, covering multiple complex scenes. In addition, we also provided the camera metadata, 3D reference model, depth map of the visible images and 6-DoF pose of all images. We extensively evaluated the performance of state-of-the-art algorithms on our dataset and provided a comprehensive analysis of the results. We will publish our dataset and pre-processing code.
更多查看译文
关键词
thermal and visible dataset,cross-modality matching,low-light environment
AI 理解论文
溯源树
样例
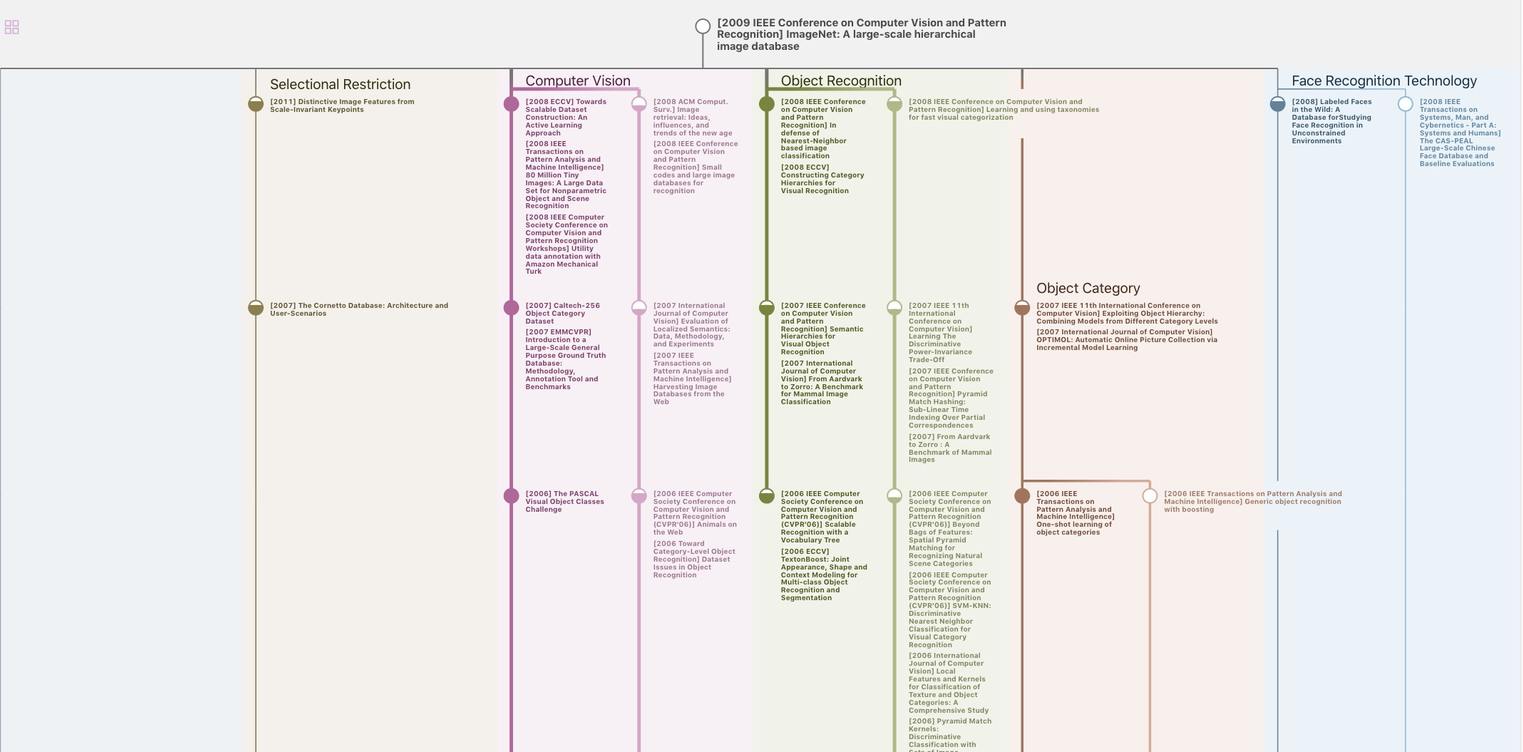
生成溯源树,研究论文发展脉络
Chat Paper
正在生成论文摘要