Domain Generalization via Nuclear Norm Regularization
arxiv(2023)
摘要
The ability to generalize to unseen domains is crucial for machine learning systems deployed in the real world, especially when we only have data from limited training domains. In this paper, we propose a simple and effective regularization method based on the nuclear norm of the learned features for domain generalization. Intuitively, the proposed regularizer mitigates the impacts of environmental features and encourages learning domain-invariant features. Theoretically, we provide insights into why nuclear norm regularization is more effective compared to ERM and alternative regularization methods. Empirically, we conduct extensive experiments on both synthetic and real datasets. We show that nuclear norm regularization achieves strong performance compared to baselines in a wide range of domain generalization tasks. Moreover, our regularizer is broadly applicable with various methods such as ERM and SWAD with consistently improved performance, e.g., 1.7% and 0.9% test accuracy improvements respectively on the DomainBed benchmark.
更多查看译文
关键词
nuclear norm regularization,domain generalization
AI 理解论文
溯源树
样例
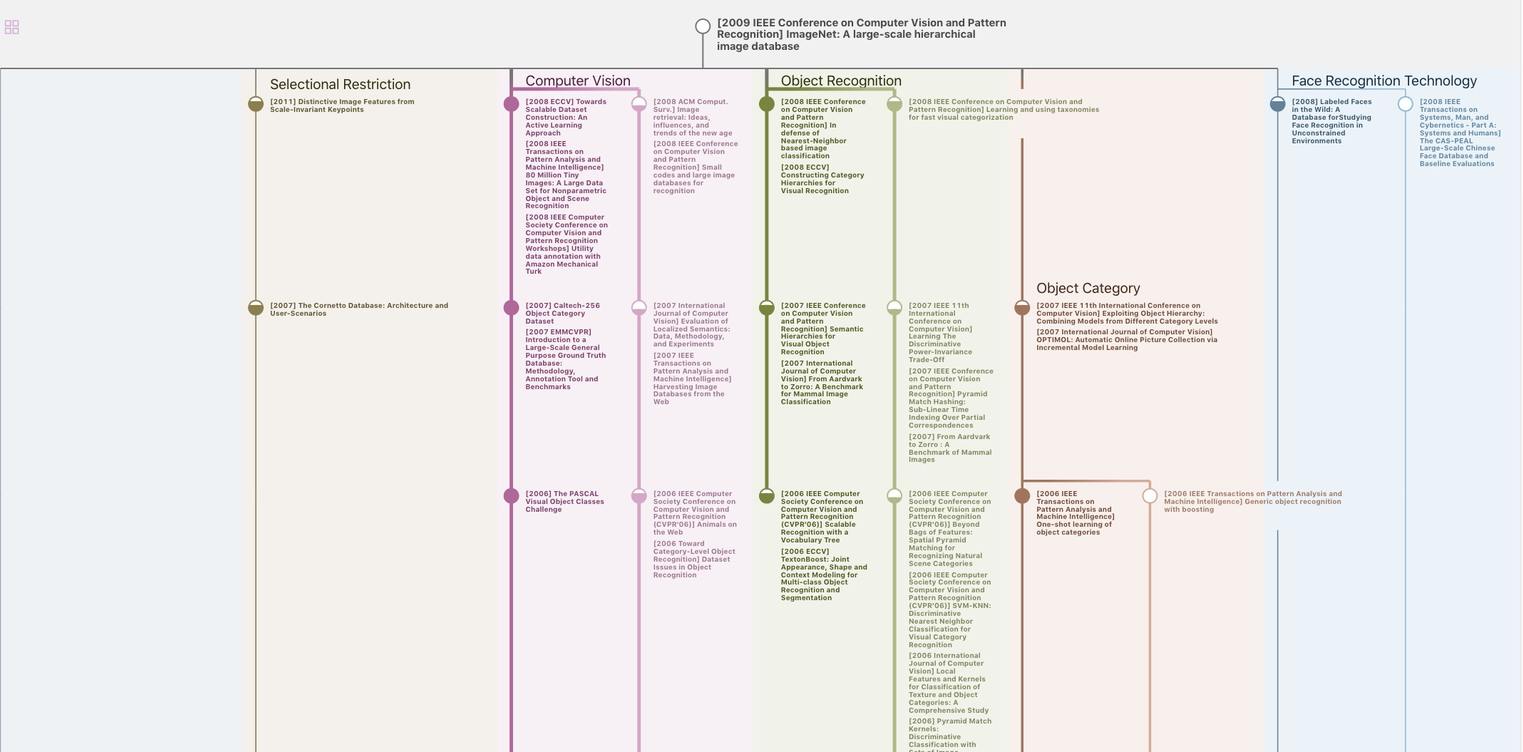
生成溯源树,研究论文发展脉络
Chat Paper
正在生成论文摘要