Development and validation of a novel radiomic model for predicting post-treatment prostate cancer recurrence
European Urology(2023)
摘要
317 Background: Multiparametric magnetic resonance imaging (mpMRI)-derived radiomic features have been shown to capture sub-visual patterns for quantitative characterization of tumor phenotypes in prostate cancer (PC) patients. The present study seeks to develop, test, and validate a mpMRI-derived radiomic model for predicting PC recurrence following initial treatment. Methods: mpMRI was obtained from 76 patients who had undergone radical prostatectomy for treatment of localized PC. All patients had >2 years follow-up with monitoring via prostate specific antigen (PSA). Patients with neo-adjuvant/adjuvant treatment were excluded. Regions of interest were manually delineated on each mpMRI and radiomics features were extracted. Stability on histogram matching was assessed via interclass correlation coefficients (ICC) and a pre-established feature selection pipeline excluded redundant features. The most important and non-redundant features were then aggregated into a radiomic model against a primary outcome of PC recurrence. Patients were randomly split 3 to 1 to training and validation datasets and the training dataset was used to iteratively train and test the best parameters for a Random Forest predictive model. The model with the best parameters as determined by receiver-operator curve (ROC) analysis was then applied to the validation set. ROC analysis was conducted and sensitivity, specificity, positive predictive value (PPV), and negative predictive value (NPV) were reported. Results: Clinicodemographic of the 76 patients included in analysis are displayed in Table. 924 radiomic features were initially extracted and 75 (8.1%) features were determined to be stable and robust following histogram matching. Based on the figure selection pipeline, six features were determined to be important and non-redundant. These features were iteratively tested in the training dataset (n=56) and the model with the best parameters yielded a mean ROC with area under the curve (AUC) of 0.95 ± 0.06. After application to validation dataset (n=20), the model yielded an AUC of 0.67. Sensitivity, specificity, PPV, and NPV were 33%, 100%, 40% and 100%, respectively. Conclusions: Radiomic analysis of 76 PC patients yielded six mpMRI-derived radiomic features significantly correlated with PC recurrence following primary treatment. When aggregated and applied to a validation dataset, the final radiomic model yielded 100% specificity in predicting PC recurrence. These findings represent excellent potential for the development of a radiomic-based diagnostic tool with high positive predictive value in identifying patients at high-risk for PC recurrence at time of initial PC diagnosis. Future projects will seek to incorporate patient demographics and disease characteristics to further improve model sensitivity and validation of this model will be pursued with an external cohort of patients.[Table: see text]
更多查看译文
关键词
prostate cancer,novel radiomic model,post-treatment
AI 理解论文
溯源树
样例
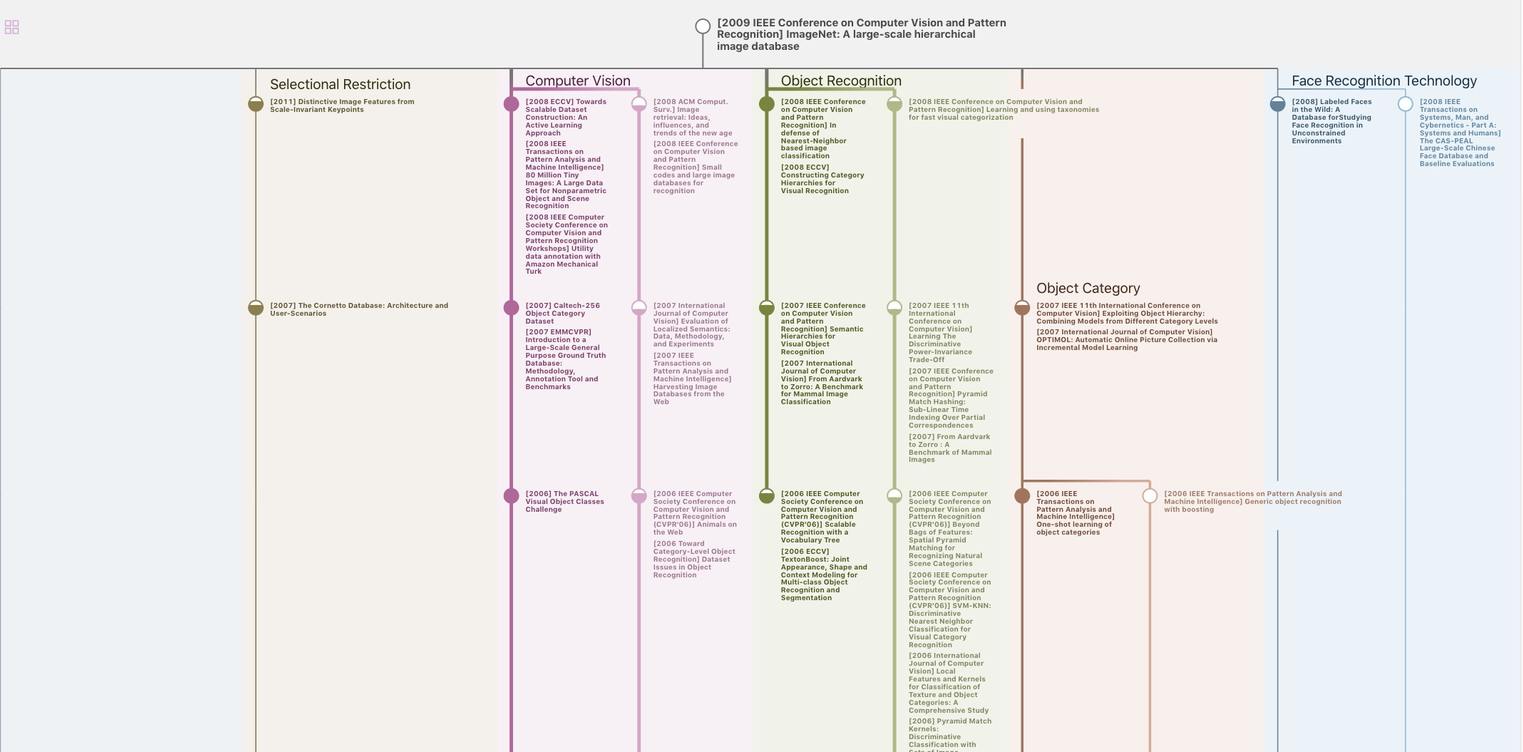
生成溯源树,研究论文发展脉络
Chat Paper
正在生成论文摘要