Compressed Heterogeneous Graph for Abstractive Multi-Document Summarization
arxiv(2023)
摘要
Multi-document summarization (MDS) aims to generate a summary for a number of related documents. We propose HGSUM, an MDS model that extends an encoder-decoder architecture, to incorporate a heterogeneous graph to represent different semantic units (e.g., words and sentences) of the documents. This contrasts with existing MDS models which do not consider different edge types of graphs and as such do not capture the diversity of relationships in the documents. To preserve only key information and relationships of the documents in the heterogeneous graph, HGSUM uses graph pooling to compress the input graph. And to guide HGSUM to learn compression, we introduce an additional objective that maximizes the similarity between the compressed graph and the graph constructed from the ground-truth summary during training. HGSUM is trained end-to-end with graph similarity and standard cross-entropy objectives. Experimental results over MULTI-NEWS, WCEP-100, and ARXIV show that HGSUM outperforms state-of-the-art MDS models. The code for our model and experiments is available at: https://github.com/oaimli/HGSum.
更多查看译文
关键词
compressed heterogeneous graph,summarization,multi-document
AI 理解论文
溯源树
样例
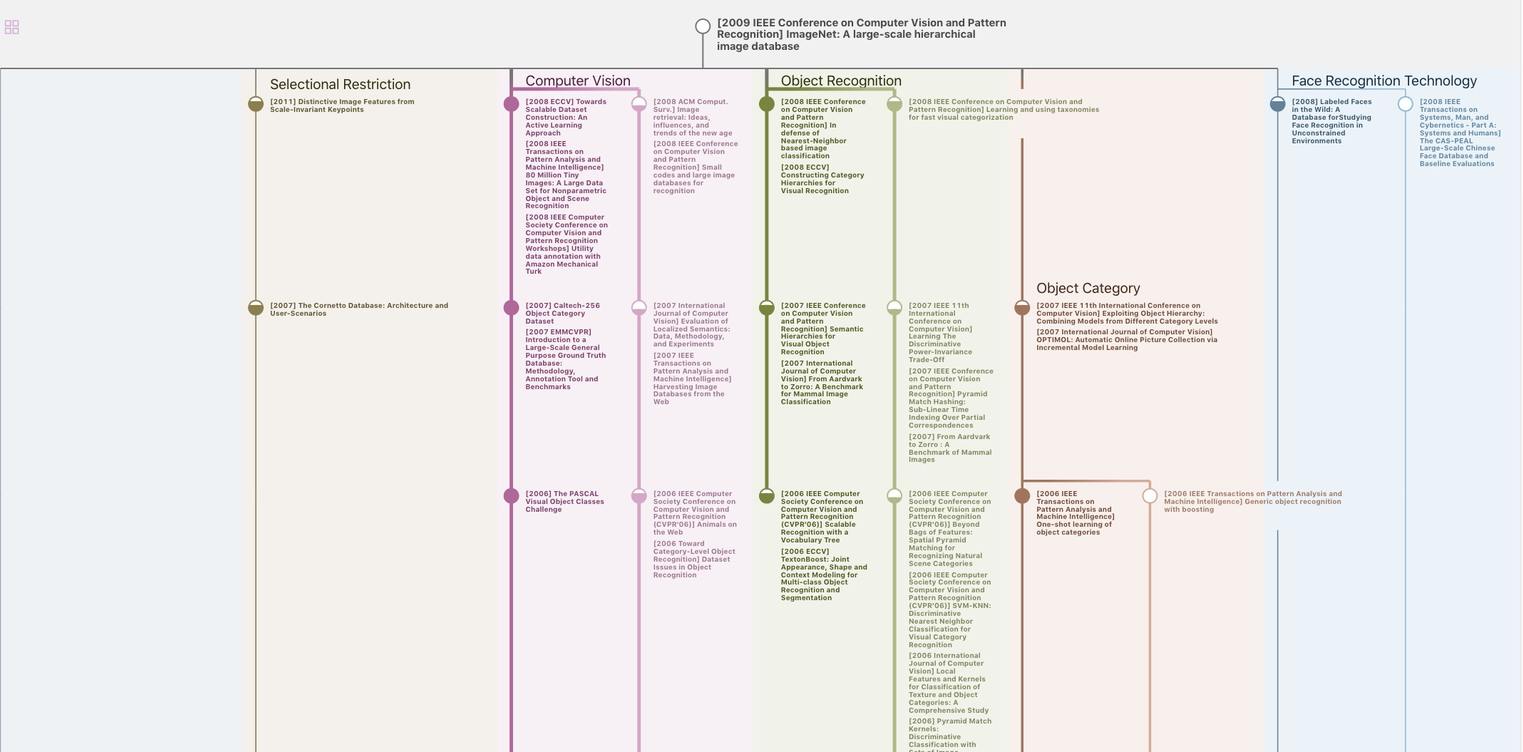
生成溯源树,研究论文发展脉络
Chat Paper
正在生成论文摘要